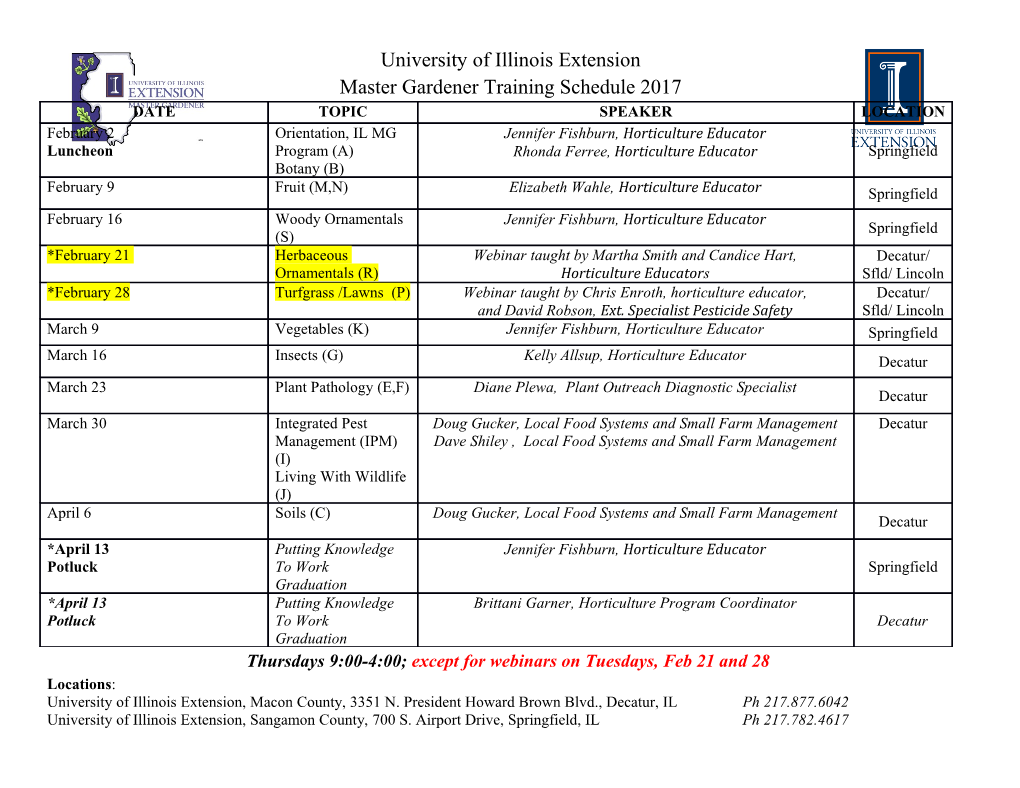
HIGHLIGHTED ARTICLE GENETICS | COMMUNICATIONS Do Molecular Markers Inform About Pleiotropy? Daniel Gianola,*,†,‡,1 Gustavo de los Campos,§ Miguel A. Toro,** Hugo Naya,†† Chris-Carolin Schön,†,‡ and Daniel Sorensen‡‡ *Departments of Animal Sciences, Dairy Science, and Biostatistics and Medical Informatics, University of Wisconsin–Madison, Wisconsin 53706, †Department of Plant Sciences Technical University of Munich, Center for Life and Food Sciences, D-85354 Freising-Weihenstephan, Germany, ‡Institute of Advanced Study, Technical University of Munich, D-85748 Garching, Germany, §Department of Biostatistics, Michigan State University, East Lansing, Michigan 48824, **Escuela Técnica Superior de Ingenieros Agrónomos, Universidad Politécnica de Madrid, 20840 Madrid, Spain, ††Institut Pasteur de Montevideo, Mataojo 2020, Montevideo 11400, Uruguay, and ‡‡Department of Molecular Biology and Genetics, Aarhus University, DK-8000 Aarhus C, Denmark ABSTRACT The availability of dense panels of common single-nucleotide polymorphisms and sequence variants has facilitated the study of statistical features of the genetic architecture of complex traits and diseases via whole-genome regressions (WGRs). At the onset, traits were analyzed trait by trait, but recently, WGRs have been extended for analysis of several traits jointly. The expectation is that such an approach would offer insight into mechanisms that cause trait associations, such as pleiotropy. We demonstrate that correlation parameters inferred using markers can give a distorted picture of the genetic correlation between traits. In the absence of knowledge of linkage disequilibrium relationships between quantitative or disease trait loci and markers, speculating about genetic correlation and its causes (e.g., pleiotropy) using genomic data is conjectural. KEYWORDS genetic correlation; genomic correlation; genomic heritability; linkage disequilibrium; pleiotropy HE interindividual differences for a trait or disease risk 1996; Lynch and Ritland 1999). This development has Tthat can be explained by genetic factors, such as trait opened new opportunities for study of the genetic architec- 2 heritability (h ), the genetic correlation (rG), and the coher- ture of complex traits and diseases. For instance, Yang et al. itability between two traits (rGh1h2), are very important pa- (2010) suggested using whole-genome regressions (WGRs) rameters in quantitative genetic studies of animals, humans, (Meuwissen et al. 2001) to assess the proportion of variance and plants. These quantities play a role in the study of evo- of a trait or disease risk that can be explained by a regression lution due to artificial and natural selection, and knowledge of phenotypes on common SNPs or genomic heritability and thereof is required for statistical prediction of outcomes in a related parameter, the “missing heritability.” More recently, animal and plant breeding as well as medicine. Traditionally, WGR models have been extended for the analysis of systems these parameters have been estimated using phenotypes and of multiple traits, so the concept of genomic correlation also pedigrees, e.g., family and twin data in human genetics. The has entered into the picture (Jia and Jannink 2012; Lee et al. availability of dense panels of common single-nucleotide 2012). For instance, Maier et al. (2015) used multivariate polymorphisms (SNPs) and of sequence data more recently WGR models and reported estimates of genetic correlations has made it possible to assess kinship among distantly related between psychiatric disorders, and Furlotte and Eskin (2015) individuals (Morton et al. 1971; Thompson 1975; Ritland presented a methodology that incorporates genetic marker information for the analysis of multiple traits that, according to the authors, “provide fundamental insights into the nature Copyright © 2015 by the Genetics Society of America of co-expressed genes.” In a similar spirit, Korte et al. (2012) doi: 10.1534/genetics.115.179978 Manuscript received June 26, 2015; accepted for publication July 21, 2015; published argued that multitrait-marker-enabled regressions can be useful Early Online July 23, 2015. for understanding pleiotropy. More recently, Bulik-Sullivan et al. 1Corresponding author: Department of Animal Sciences, University of Wisconsin– “ Madison, 1675 Observatory Drive, Madison, WI 53706. (2015) proposed a methodology for estimating genetic corre- E-mail: [email protected] lation” using statistics derived from single-marker genome-wide Genetics, Vol. 201, 23–29 September 2015 23 2 ¼ð 2 r2 Þ 2 association studies (GWAS) and reported estimates of such missing heritability is hmissing 1 QX h .Hence,missing correlations among 25 human traits. heritability is a function of the LD between the marker and de los Campos et al. (2015) discussed potential problems the QTL. Genomic heritability has h2 as an upper bound (de that emerge when trying to infer genetic parameters using los Campos et al. 2015). molecular markers that are imperfectly associated with the gen- The regression model just described can be extended to the otypes at the causal loci. In this paper, the framework described analysis of multiple traits affected by multiple QTL. For in de los Campos et al. (2015) is extended for the analysis of simplicity, we consider only two markers (X1 and X2) and systems of traits, and it is demonstrated that correlation param- two QTL (Q1 and Q2). A multivariate representation of the eters inferred using markers can give a distorted picture of the model with an arbitrary number of QTL and markers is pro- genetic correlation between traits. For instance, it is shown that vided in the Appendix. Figure 1 depicts a system with two an analysis based on markers may suggest a genetic correlation traits, two QTL, and two markers. The left panel represents when none exists or may fail to detect a genetic correlation the regression of the phenotypes on the two QTL, with blue when one does exist. It is concluded that in the absence of arrows denoting effects from QTL on traits and green arcs knowledge about linkage disequilibrium (LD) relationships be- denoting LD between QTL. In the QTL model of Figure 1, the tween quantitative trait loci (QTL) and markers, speculating genetic correlation is (see Appendix) about genetic correlations, and even more about their causes a9S a 1 Q 2 (e.g., pleiotropy), using genomic data is conjectural. rG ¼ qÀffiffiffiffiffiffiffiffiffiffiffiffiffiffiffiffiffiffiffiffiffiffiffiffiffiffiffiffiffiffiffiffiffiffiffiffiffiffiffiffiffiÁÀ Á (3) a9S a a9S a 1 Q 1 2 Q 2 Theory a9 ¼ða a Þ where 1 11 12 contains the effects of QTL 1 and 2 To set the stage, consider a single-locus model. In an additive- a9 ¼ða a Þ on trait 1, and 2 21 22 contains the effects of QTL 1 inheritance framework, a phenotype (y) is regressed on a QTL and 2 on trait 2. The variance-covariance matrix between genotype code Q (0, 1, and 2 for genotypes aa, Aa, and AA, QTL genotypes is given by SQ. If genotypes are standardized, respectively) according to the linear model 1 r Q12 SQ ¼ y ¼ a0 þ a1Q þ E (1) r Q21 1 where a0 and a1 are fixed parameters, and Q and E are in- r with Q12 being the correlation between genotypes at QTL 1 dependent random variables, the latter representing a model and 2. In the QTL model of Figure 1, there are two sources of residual. The proportion of phenotypic variance explained by genetic correlation: pleiotropy (i.e., the same QTL affects the linear regression on Q, or narrow-sense heritability, is more than one trait) and LD between QTL, in this case rep- resented by r 6¼ 0. This is well known in quantitative ge- a2S Q12 h2 ¼ 1 Q netics (Falconer and Mackay 1996; Knott and Haley 2000). a2S þ s2 1 Q E We now bring the two markers into the picture, as shown in therightpanelofFigure1;heregrayarrowsareregressionson where S ¼ varðQÞ is the variance in allelic content, and Q markers (these are distinct from regressions on QTL genotypes), s2 ¼ varðEÞ is the residual variance. If Q is standardized to E and arcs denote correlations between genotypes due to LD. In a unit variance, the Appendix, we show that the genomic correlation is 2 a 2 S ¼ 1 and h2 ¼ 1 a9S S 1S a Q a2 þ s2 ¼ rffiffiffiffiffiffiffiffiffiffiffiffiffiffiffiffiffiffiffiffiffiffiffiffiffiffiffiffiffiffiffiffiffiffiffiffiffiffiffiffiffiffiffiffiffiffiffiffiffiffiffiffiffiffiffiffiffiffiffiffiffiffiffiffiffiffiffiffiffiffiffiffiffiffiffiffiffiffiffiffiffiffiffi1 QX X XQ 2 1 E rG;marked a9S S21S a a9S S21S a 1 QX X XQ 1 2 QX X XQ 2 In quantitative genomic analysis, marker genotypes (X) are (4) used in lieu of the QTL genotypes Q because the latter are unknown or unobserved. The marker-based or instrumental In this expression, SQX is the covariance matrix between QTL model, assuming a single marker, is a linear regression on and marker genotypes (reflecting marker-QTL LD), and SX is marker genotype X with form the covariance matrix between marker genotypes, reflecting mutual LD relationships among markers. If markers and gen- ¼ a þ b þ 9 y 0 1X E (2) otypes are in standard deviation units, where E9 is a regression residual. Assuming without loss of r r r S ¼ 1 X12 S ¼ Q1X1 Q1X2 X r and QX r r generality that both X and Q are in standard deviation units, X 1 Q X Q X b ¼ r a r 21 2 1 2 2 the marker effect can be shown to be 1 QX 1, where QX is the correlation between the marker and the QTL genotypes, Comparison of the genomic correlation (4) with the genetic S S21S which depends on their LD. In this setting, the proportion of correlation (3) indicates that in rrG;marked , QX X XQ replaces variance of phenotypes explained by the linear regression on SQ: Inspection of (4) reveals that the sources of the genomic 2 ¼ r2 2 a a the marker, or genomic heritability, is hmarked QX h ,and correlation are (1) pleiotropic QTL effects via 1 and 2, (2) 24 D. Gianola et al. Figure 1 Two-trait system. A system of two traits (Y) involving two QTL and two markers (X). Single-pointed blue arrows denote causal effects, green double-pointed arrows denote LD, and single-pointed gray arrows represent regression coefficients.
Details
-
File Typepdf
-
Upload Time-
-
Content LanguagesEnglish
-
Upload UserAnonymous/Not logged-in
-
File Pages7 Page
-
File Size-