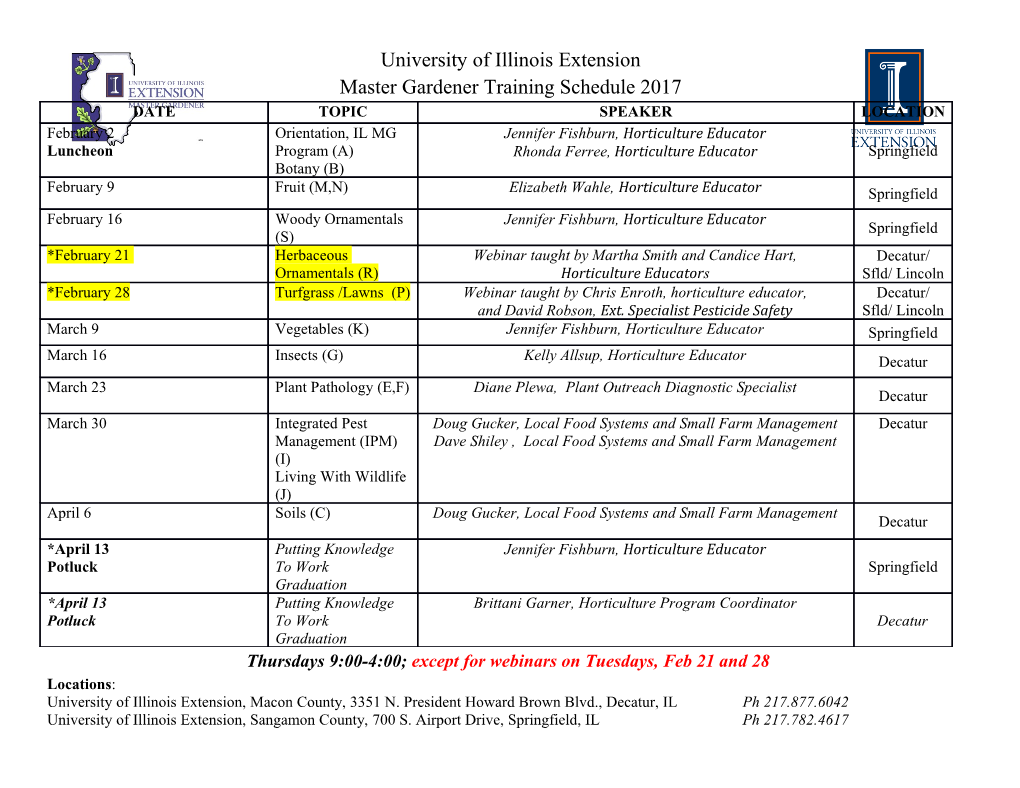
Air Force Institute of Technology AFIT Scholar Theses and Dissertations Student Graduate Works 6-16-2016 A Multi-Objective Approach to Tactical Maneuvering Within Real Time Strategy Games Christopher D. Ball Follow this and additional works at: https://scholar.afit.edu/etd Part of the Computer Sciences Commons Recommended Citation Ball, Christopher D., "A Multi-Objective Approach to Tactical Maneuvering Within Real Time Strategy Games" (2016). Theses and Dissertations. 457. https://scholar.afit.edu/etd/457 This Thesis is brought to you for free and open access by the Student Graduate Works at AFIT Scholar. It has been accepted for inclusion in Theses and Dissertations by an authorized administrator of AFIT Scholar. For more information, please contact [email protected]. A MULTI-OBJECTIVE APPROACH TO TACTICAL MANUVERING WITHIN REAL TIME STRATEGY GAMES THESIS Christopher D. Ball, Capt, USAF AFIT-ENG-MS-16-J-004 DEPARTMENT OF THE AIR FORCE AIR UNIVERSITY AIR FORCE INSTITUTE OF TECHNOLOGY Wright-Patterson Air Force Base, Ohio DISTRIBUTION STATEMENT A APPROVED FOR PUBLIC RELEASE; DISTRIBUTION UNLIMITED. The views expressed in this document are those of the author and do not reflect the official policy or position of the United States Air Force, the United States Department of Defense or the United States Government. This material is declared a work of the U.S. Government and is not subject to copyright protection in the United States. AFIT-ENG-MS-16-J-004 A MULTI-OBJECTIVE APPROACH TO TACTICAL MANEUVERING WITHIN REAL TIME STRATEGY GAMES THESIS Presented to the Faculty Department of Electrical and Computer Engineering Graduate School of Engineering and Management Air Force Institute of Technology Air University Air Education and Training Command in Partial Fulfillment of the Requirements for the Degree of Master of Science in Computer Science Christopher D. Ball, B.S.C.E. Capt, USAF June 2016 DISTRIBUTION STATEMENT A APPROVED FOR PUBLIC RELEASE; DISTRIBUTION UNLIMITED. AFIT-ENG-MS-16-J-004 A MULTI-OBJECTIVE APPROACH TO TACTICAL MANEUVERING WITHIN REAL TIME STRATEGY GAMES THESIS Christopher D. Ball, B.S.C.E. Capt, USAF Committee Membership: Dr. G. Lamont Chair Dr. B. Borghetti Member Maj B. Woolley, PhD Member AFIT-ENG-MS-16-J-004 Abstract The real time strategy (RTS) environment is a strong platform for simulating complex tactical problems. The overall research goal is to develop artificial intelligence (AI) RTS planning agents for military critical decision making education. These agents should have the ability to perform at an expert level as well as to assess a players critical decision-making ability or skill-level. The nature of the time sensitivity within the RTS environment creates very complex situations. Each situation must be analyzed and orders must be given to each tactical unit before the scenario on the battlefield changes and makes the decisions no longer relevant. This particular research effort of RTS AI development focuses on constructing a unique approach for tactical unit positioning within an RTS environment. By utilizing multiobjective evolutionary algorithms (MOEAs) for finding an \optimal" positioning solution, an AI agent can quickly determine an effective unit positioning solution with a fast, rapid response. The development of such an RTS AI agent goes through three distinctive phases. The first of which is mathematically describing the problem space of the tactical positioning of units within a combat scenario. Such a definition allows for the de- velopment of a generic MOEA search algorithm that is applicable to nearly every scenario. The next major phase requires the development and integration of this algorithm into the Air Force Institute of Technology RTS AI agent. Finally, the last phase involves experimenting with the positioning agent in order to determine the effectiveness and efficiency when placed against various other tactical options. Ex- perimental results validate that controlling the position of the units within a tactical situation is an effective alternative for an RTS AI agent to win a battle. iv Acknowledgements I would like to thank Dr. Gary Lamont for guiding me his vast experience in the field of Artificial Intelligence, being of great assistance in this research effort. Gratitude also extends towards Jason Blackford and Donald Gruber for their prior efforts and inspiration for my project. Christopher D. Ball v Table of Contents Page Abstract . iv Acknowledgements . .v List of Figures . ix List of Tables . xi I. Introduction . .1 1.1 Military Tactical Decision Making . .1 1.2 Real Time Strategy Games . .2 1.3 Research Goal . .2 1.4 Research Objectives . .2 1.5 Research Approach . .3 1.6 Thesis Organization . .4 II. Background . .6 2.1 Decision Making . .6 OODALoop....................................................7 Thin Slicing . .8 Current State Analysis . .9 2.2 Real Time Strategy (RTS) Games . .9 DuneII....................................................... 10 WarCraft and StarCraft . 11 Total Annihilation . 12 Company of Heroes . 13 Spring RTS Engine . 14 Tactical Airpower Visualizaton . 15 2.3 RTS Development Platforms . 15 Wargus ....................................................... 16 SparCraft . 16 2.4 Strategic Decision Making . 17 Strategy in RTS Games . 18 2.5 Tactical Decision Making . 19 Tactics in RTS Games . 20 Maneuvering Tactics in Combat Scenarios . 21 2.6 Developing a RTS AI Agent . 22 RTS Agent Subfunction Breakdown . 24 2.7 Previous AFIT Agent Developments . 24 Adaptive Response - Weissgerber's Agent . 24 vi Page Strategy Optimization - Di Trapani's Agent . 25 Build Order Optimization - Blackford's Agent . 25 Tactics Optimization - Gruber's Agent . 25 Unit Management - AFIT RTS AI Agent Continuing Work ................................................... 26 2.8 Current Research in RTS AI Tactics Optimization . 27 David Churchill's Research . 27 Formation Management . 28 Boids......................................................... 29 RTS AI Genetic Algorithm Implementations . 30 2.9 Multi-Objective Evolutionaly Algorithms . 30 MOEA Software Packages . 31 Description of MOEA Algorithms . 32 2.10 Using MOEAs in RTS AI Tactics . 35 2.11 Chapter Summary . 35 III. Methodology of the Positional MOEA . 36 3.1 Phase 1 - Defining the Problem Space . 36 Tactical Movement in RTS Games . 36 High Level Design . 37 Low Level Design. 43 3.2 Phase II - Integration into AFIT Agent . 48 AFIT RTS AI Agent . 49 Controlling Units in the Spring Engine . 52 3.3 Phase III - Real-time execution in Spring RTS . 53 Functionality Testing in the Spring Engine . 53 Adjustments for a Real Time Environment . 56 3.4 Chapter Summary . 57 IV. Design of Experiments . 58 4.1 Introduction . 58 4.2 Experiment Test Equipment . 58 4.3 First Experiment: Finding the best MOEA . 59 First Experimental Objective . 59 Test Establishment . 59 Sequence for Testing . 59 Metrics for Analysis . 60 Structure of Results . 61 Experimental Hypothesis . 61 4.4 Second Experiment: Determining Effectiveness of the Positioning Algorithm in the Spring Engine . ..
Details
-
File Typepdf
-
Upload Time-
-
Content LanguagesEnglish
-
Upload UserAnonymous/Not logged-in
-
File Pages141 Page
-
File Size-