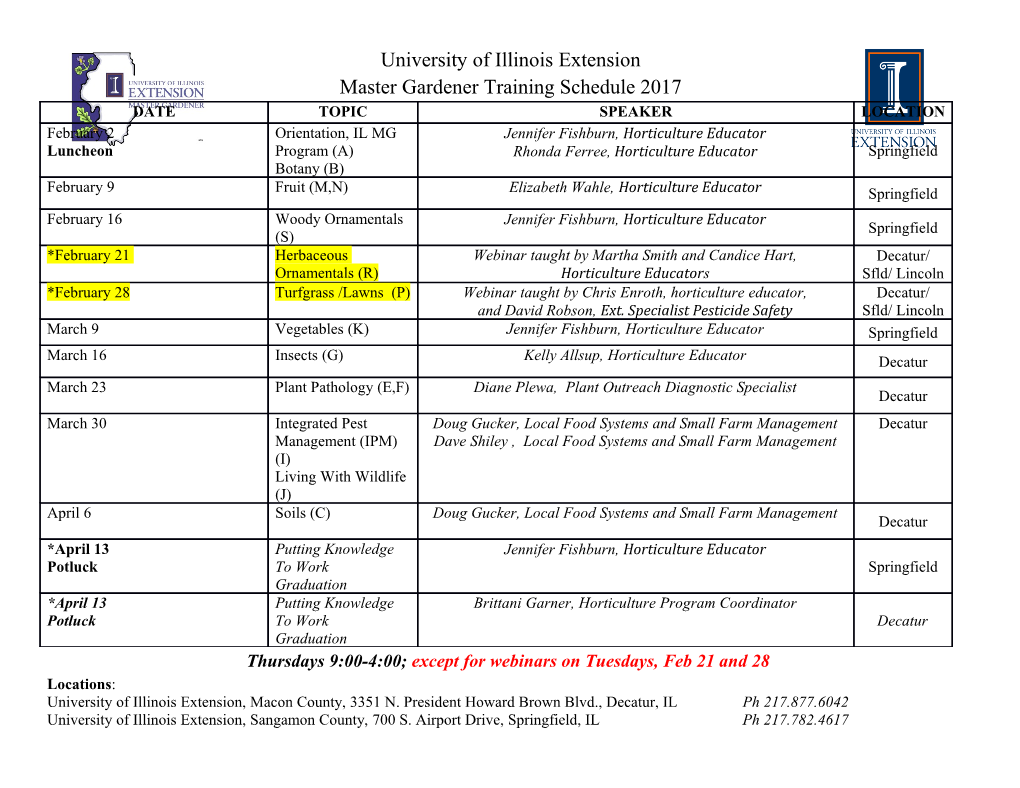
Hierarchical Attention Prototypical Networks for Few-Shot Text Classification Shengli Sun1 Qingfeng Sun1 Kevin Zhou2 † Tengchao Lv1 1 2 ∗ Peking University∗ Microsoft [email protected] fsunqingfeng, [email protected] [email protected] Abstract prototypical networks is rough and does not con- sider the adverse effects of various noises in the Most of the current effective methods for text data, which weakens the discrimination and ex- classification task are based on large-scale la- beled data and a great number of parame- pressiveness of the prototype. ters, but when the supervised training data are In this paper, we propose a hierarchical atten- few and difficult to be collected, these mod- tion prototypical networks for few-shot text clas- els are not available. In this paper, we pro- sification by using attention mechanism in three pose a hierarchical attention prototypical net- levels. For feature level attention, we use convo- works (HAPN) for few-shot text classifica- lutional neural networks to get the feature scores tion. We design the feature level, word level, which is different for various classes. For word and instance level multi cross attention for our model to enhance the expressive ability of se- level attention, we adopt an attention mechanism mantic space. We verify the effectiveness of to learn the importance of each word hidden state our model on two standard benchmark few- in an instance. For instance level multi cross atten- shot text classification datasets - FewRel and tion, with the help of multi cross attention between CSID, and achieve the state-of-the-art perfor- support set and target query, we can determine the mance. The visualization of hierarchical atten- importance of different instances in the same class tion layers illustrates that our model can cap- and enable the model to get a more discriminative ture more important features, words, and in- stances separately. In addition, our attention prototype of each class. mechanism increases support set augmentabil- In the actual scenario, we apply HAPN on in- ity and accelerates convergence speed in the tention detection of our open domain chatbots with training stage. different character. If we create a chatbot for old people, the user intentions will focus on children, 1 Introduction health or expectation, so we can define specific The dominant text classification models in deep intentions and supply related responses. And be- learning (Kim, 2014; Zhang et al., 2015a; Yang cause of only few data are needed, we can expand et al., 2016; Wang et al., 2018) require a consider- the number of classes quickly. The model helps able amount of labeled data to learn a large num- chatbot to identify user intentions precisely, makes ber of parameters. However, such methods may the dialogue process smoother, more knowledge- have difficulty in learning the semantic space in able and more controllable. the case that only few data are available. Few-shot There are three main parts of our contribution: learning has became an effective approach to solve first of all, we propose a hierarchical attention pro- this challenge, it can train a neural network with a totypical networks for few-shot text classification, few parameters using few data but achieve good then we achieve state-of-the-art performance on performance. A typical example of this approach FewRel and CSID datasets, and the experiments is prototypical networks (Snell et al., 2017), which prove our model is faster and more extensible. averages the vector of few support instances as the class prototype and computes distance between 2 Related Works target query and each prototype, then classify the query to the nearest prototype’s class. However, 2.1 Text Classification ∗ They contribute equally to this work. Text Classification is an important task in Natu- † Corresponding author. ral Language Processing, and many models are 476 Proceedings of the 2019 Conference on Empirical Methods in Natural Language Processing and the 9th International Joint Conference on Natural Language Processing, pages 476–485, Hong Kong, China, November 3–7, 2019. c 2019 Association for Computational Linguistics Figure 1: Hierarchical Attention Prototypical Networks architecture proposed to solve it. The traditional methods an unlabelled example to its label, and obviate the mainly focus on feature engineerings such as bag- need for fine-tuning to adapt to new class types. of-words or n-grams (Wang and Manning, 2012) Prototypical networks (Snell et al., 2017) learns a or SVMs (Tang et al., 2015). The neural network metric space in which the model can perform well based methods like Kim(2014) applies convolu- by computing distance between query and proto- tional neural networks for sentence classification. type representations of each class and classify the Then, Johnson and Zhang(2015) use a one-hot query to the nearest prototype’s class. Sung et al. word order CNN, and Zhang et al.(2015b) ap- (2018) propose a two-branch relation networks, ply a character level CNN. C-LSTM (Zhou et al., which learns to compare query against few-shot 2015) combines CNN and RNN for sentence rep- labeled sample support data. Dual TriNet struc- resentation and text classification. Yang et al. ture (Chen et al., 2018) can efficiently and directly (2016) explore the hierarchical structure of docu- augment multi-layer visual features to boost the ments classification, they use a GRU-based atten- few-shot classification.But all of the above works tion to build representations of sentences and an- mainly concentrate on computer vision field, the other GRU-based attention to aggregate them into research and applications in NLP field are ex- a document representation. But above supervised tremely limited. Recently, Yu et al.(2018) propose learning methods require large-scale labeled data an adaptive metric learning approach that automat- and can’t classify unseen classes. ically determines the best weighted combination from a set of metrics obtained from meta-training 2.2 Few-Shot Learning tasks for a newly seen few-shot task such as in- Few-Shot Learning (FSL) aims to solve classifi- tention classification, Han et al.(2018) present cation problems by training a classifier with few a relation classification dataset - FewRel, and instances in each class, and it can apply to un- adapt most recent state-of-the-art few-shot learn- seen classes. The early works aim to use transfer ing methods for it, Gao et al.(2019) propose a learning approaches, Caruana(1994) and Bengio hybrid attention-based prototypical networks for (2011) adopt the target task from the pre-trained noisy few-shot relation classification. However, models. Then Koch et al.(2015) explore a method these methods do not consider mining semantic for learning siamese neural networks which em- information or reducing the impact of noise more ploys an unique structure to rank similarity be- precisely. And in most of the realistic settings, we tween inputs. Vinyals et al.(2016) use matching may increase the number of instances gradually, networks to map a small labeled support set and so model capacity needs more attention. 477 3 Task Definition erarchical attention mechanism. Feature level at- tention enhances or reduces the importance of dif- In few-shot text classification task, our goal is to ferent feature in each class, word level attention learn a function : G D; S; x y. D is the la- ( ) → highlight the important words for meaning of the beled data, we divide D into three parts: D , train instance, and instance level multi cross attention D , and D , and each part has specific validation test can extract the important support instances for dif- label space. We use D to optimize parame- train ferent query instances, these three attention mech- ters, D to select best hyper parameters, validation anisms work together to improve the classification and D to evaluate the model. test performance of our model. The “episode” training strategy that Vinyals Prototypical Networks Prototypical networks et al.(2016) proposed has proved to be effective. compute a prototype vector as the representation For each training episode, we first sample a label of each class, and this vector is the mean vector of set from D , then use to sample the sup- L train L the embedded support instances belonging to its port set S and the query set Q, finally, we feed S class. We compare the distance between all proto- and Q to the model and minimize the loss. If L type vectors and a target query vector, then clas- includes N different classes and each class of S sify this query to the nearest one. contains K instances, we call the target problem N-way K-shot learning. For this paper, we con- 4.2 Instance Encoder sider N = 5 or 10, and K = 5 or 10. The instance encoder part consists of two layers: For exactly, in an episode, we are given a sup- embedding layer and instance encoding layer. port set S 4.2.1 Embedding Layer 1 2 n1 S ={(x1; l1); (x1; l1);:::; (x1 ; l1); Given an instance x = {wt; w2; : : : ; wT } with T ⋯; words. We use an embedding matrix WE,wt = (1) 1 2 nm WEwt to embed each word to a vector (xm; lm); (xm; lm);:::; (xm ; lm)}; w ; w ;:::; w ; w d (2) l1; l2; ⋯; lm ∈ L { 1 2 T } t ∈ R where d is the word embedding dimension. consists of ni text instances for each class li ∈ L, j xi means it is the j support instance belonging 4.2.2 Encoding Layer j to calss li, and instance xi includes Ti;j words Following we apply a convolutional neural net- {w1; w2; : : : ; wTi;j }. work Zeng et al.(2014) as encoding layer to get Then x is an unlabeled instance of query set Q the hidden annotations of each word by a convolu- to classify, and y ∈ L is the output label followed tion kernel with the window size m by the prediction of G.
Details
-
File Typepdf
-
Upload Time-
-
Content LanguagesEnglish
-
Upload UserAnonymous/Not logged-in
-
File Pages10 Page
-
File Size-