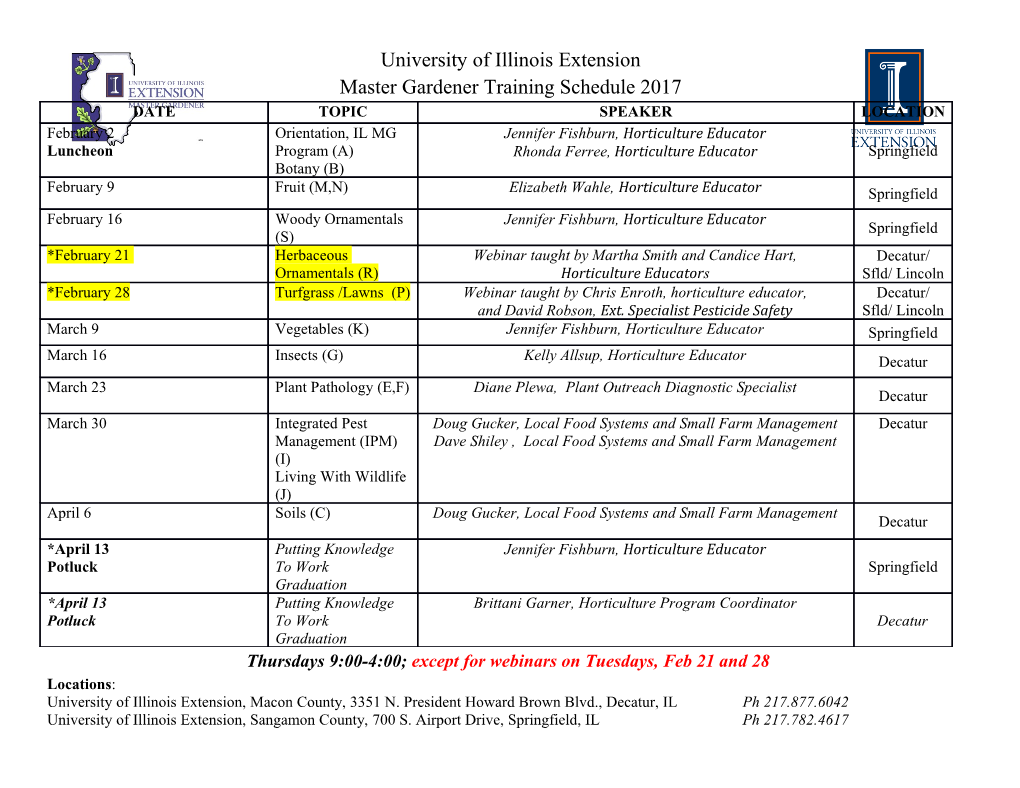
A HMM Approach to Identifying Distinct DNA Methylation Patterns for Subtypes of Breast Cancers Thesis Presented in Partial Fulfillment of the Requirements for the Degree Master of Science in the Graduate School of The Ohio State University By Maoxiong Xu, B.S. Graduate Program in Computer Science and Engineering The Ohio State University 2011 Thesis Committee: Victor X. Jin, Advisor Raghu Machiraju Copyright by Maoxiong Xu 2011 Abstract The United States has the highest annual incidence rates of breast cancer in the world; 128.6 per 100,000 in whites and 112.6 per 100,000 among African Americans.[1,2] It is the second-most common cancer (after skin cancer) and the second-most common cause of cancer death (after lung cancer).[1] Recent studies have demonstrated that hyper- methylation of CpG islands may be implicated in tumor genesis, acting as a mechanism to inactivate specific gene expression of a diverse array of genes (Baylin et al., 2001). Genes have been reported to be regulated by CpG hyper-methylation, include tumor suppressor genes, cell cycle related genes, DNA mismatch repair genes, hormone receptors and tissue or cell adhesion molecules (Yan et al., 2001). Usually, breast cancer cells may or may not have three important receptors: estrogen receptor (ER), progesterone receptor (PR), and HER2. So we will consider the ER, PR and HER2 while dealing with the data. In this thesis, we first use Hidden Markov Model (HMM) to train the methylation data from both breast cancer cells and other cancer cells. Also we did hierarchy clustering to the gene expression data for the breast cancer cells and based on the clustering results, we get the methylation distribution in each cluster. Finally, we correlate the HMM training results with the methylation distribution and get the biology meanings for the states in the HMM results. ii Dedicated to my father, mother, and wife, for all of their love and support. iii Acknowledgments I have many people to thank for my making it this far: my advisor, Dr. Victor Jin, for everything he's done; Dr. Raghu Machiraju, for his help and support; all of my lab mates, for their knowledge, assistance, and encouragement; and the incredible Biomedical Informatics Department staff for everything they do. iv Vita 2005……………………………...Mudu Central High School 2009……………………………...B.S. Computer Science, Southeast University 2009 to present……….……..……M.S. Computer Science & Engineering, The Ohio State University Sep. 2010 to present……………...Graduate Teaching Associate, Department of Bioinformatics, The Ohio State University Publications Cao AR, Rabinovich R, Xu M, Xu X, Jin VX, Farnham PJ: Genome-wide analysis of transcription factor E2F1 mutant proteins reveals that N- and C-terminal protein interaction domains do not participate in targeting E2F1 to the human genome. J Biol Chem. 2011 Apr 8; 286(14):11985-96. Epub 2011 Feb 10. Fields of Study Major: Computer Science & Engineering Machine Learning applied in Bioinformatics v Table of Contents Abstract……........................................................................................................................ii Dedication………………………………………………………………………..……….iii Acknowledgments…..........................................................................................................iv Vita......................................................................................................................................v Table of Contents ...............................................................................................................vi List of Tables .....................................................................................................................ix List of Figures.....................................................................................................................xi Chapter 1: Introduction........................................................................................................1 1.1 Methylation……………………………………………………………………1 1.1.1 What Is Methylation? ......................................................................1 1.1.2 DNA Methylation…………………………………………………2 1.1.3 DNA Methylation Mechanism……………………………….........3 1.1.4 DNA Methylation in Cancer...……………………………….........5 1.2 Gene Expression………………………………………………………………6 1.2.1 Gene Expression Measurement……………………………….…….7 1.2.2 mRNA Quantification……………………………………………8 1.2.3 Regulation of Gene Expression……………………….….……...10 1.3 Hidden Markov Model………………………………………………...…….11 1.3.1 Introduction to Hidden Markov Model…………………….……12 vi 1.3.2 Hidden Markov Model……………………………………..…….13 1.3.3 Model Architecture...…………………………………………….13 1.3.4 HMM Training and Decoding……………………………..…….14 1.3.5 HMMs in Computational Biology………………………..……...15 1.3.6 Application of HMMs to Specific Problems……………..……...16 Chapter 2: Methods and Algorithms……………………………....….………………….18 2.1 The Probabilistic Model…………………….………….…………….………18 2.2 Baum-Welch Algorithm…………………………….……………….….……19 2.3 Work Flow…………………………………………….…………….….……23 Chapter 3: Data Process…..…………………………………………………………..….26 3.1 Data Sets……………………………………………………………..………26 3.2 MBD-seq Protocol…………………………………………………..……….27 3.3 Data Preprocess…………………………………………………….….……..27 3.4 Input for HMM……………………….………………………………..…….30 3.5 Methylation Distribution Overview………………….……………….….…..33 3.6 Gene Expression Data………………………………………………….……34 Chapter 4: Results and Discussion………………………………………………….…...35 4.1 Results from HMM………………………………………………….………35 vii 4.2 Biology Meanings………………………………………………………..…..41 4.2.1 Gene Expression Results for 33 Breast Cancer Cell Lines........…..41 4.2.2 Results Based on Different Clusters…………………………..…...42 4.2.3 States Meanings and Group Patterns……………………….....…...50 Chapter 5: Data Visualization……………………………………………………..……..56 Chapter 6: Conclusions and Suggestions for Further Work………………………..…....59 6.1 Conclusion……………………………………………………………..….…59 6.2 Future Work…………………………………….……………….…..…….…60 References………………………………………………………………………...….…..61 Appendix_Formats………………………………………………………….…..…….…66 A. BAM format………………………………………………………..…..…….66 B. SAM format………………………………………………………..….….….66 C. Export format………………………………………………………..…...…..67 D. BED format………………………………………………………..…………68 E. Fastq format………………………………………………………..………...70 F. Bowtie output format………………………………………………..……….71 viii List of Tables Table 3.1 Data summary for 36 cell lines……………………...………..……………….29 Table 3.2 12 Groups for 36 cell lines……………..………………………..…….….…...31 Table 4.1 BIC results for HMM results…………………………………..………….…..35 Table 4.2 Transition Matrix…………………………………………………..…….……36 Table 4.3 Emission probabilities for each mark in each state……………………..….…38 Table 4.4 Ordered emission probabilities for each mark in each state-mark………...…..39 Table 4.5 Ordered emission probabilities for each mark in each state- probabilities.…...39 Table 4.6 Filtered ordered emission probabilities for each mark in each state- marks….40 Table 4.7 Number of genes in each cluster……………………………………..………..43 Table 4.8 First 3 marks for each state…………………………………………………..50 ix Table 4.9 States and interval correlation results………………………………………..51 Table 4.10 States meanings…………………………………………………………….52 Table 4.11 Patterns for subtypes of Breast cancers…………………………………….52 x List of Figures Fig 1.1 Methylation…………………………………………………………………….…1 Fig 1.2 DNA methylation……………………………………………………………..…..2 Fig 1.3 DNA methylation mechanism……………………………………….……….…...4 Fig 1.4 DNA methylation in cancer…………………………………………….…….…...6 Fig 1.5 Gene Expression………………………………………………………….….……6 Figure 1.6: A simple HMM λ= (A,B, π),where N = 3, M = 3, a12,a23,a32 are non-zero, b1(a), b2(t),b3(g) = 1 and π = 1, 0, 0. ……………………………………………..……...13 Fig2.1 A Broad overview of the HMM work-flow, highlighting the most significant inputs, transformations, and outputs at each step from start to end. ……………..…...…23 Fig 3.1 Bar figure for 36 cell lines……...……………………………………………..…30 Fig 3.2 Methylation distribution for 33 breast cancer cell lines……...…………..……...34 Fig 4.1 Heatmap for transition matrix…………………………………………….……..37 Fig 4.2 33 Breast Cancer Cell Gene Expression One-Way Hierarchy Clustering……....41 Fig 4.3 Grouped 33 Breast Cancer Cell Gene Expression One-Way Hierarchy Clustering …………………………………………………………………….….……...42 Fig 4.4 Methylation distribution based on cluster 1 genes……………………...……….44 Fig 4.5 Methylation distribution based on cluster 2 genes…………………...…..……...45 xi Fig 4.6 Methylation distribution based on cluster 3 genes………………………...…….45 Fig 4.7 Methylation distribution based on cluster 4 genes…………………...……….....46 Fig 4.8 Methylation distribution based on cluster 5 genes…………………….………...46 Fig 4.9 Methylation distribution based on cluster 6 genes…………………...…….........47 Fig 4.10 Methylation distribution based on cluster 7 genes………………..….………...48 Fig 4.11 Methylation distribution based on cluster 8 genes………………….……........48 Fig 4.12 Methylation distribution based on cluster 9 genes………………….……........49 Fig 5.1 Database Web Tool……………………………………………………………..56 xii Chapter 1: Introduction 1.1 Methylation 1.1.1 What Is Methylation? In the view of chemical sciences, methylation means the addition of a methyl group to a substrate or the substitution of an atom or group by a methyl group. Methylation is a form of alkylation with, to be specific, a methyl group, rather than a larger carbon chain, replacing a hydrogen atom. In the view of biological systems, methylation is catalyzed by enzymes; such methylation can be involved in modification of heavy metals, regulation of gene expression, regulation
Details
-
File Typepdf
-
Upload Time-
-
Content LanguagesEnglish
-
Upload UserAnonymous/Not logged-in
-
File Pages85 Page
-
File Size-