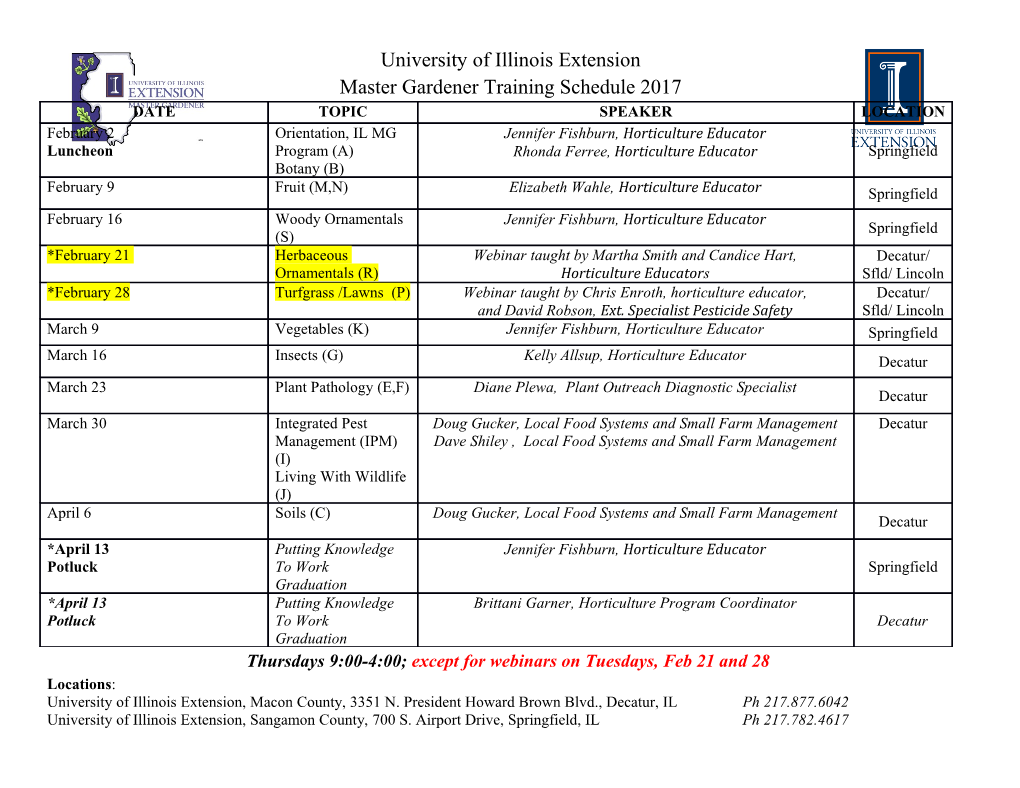
Perceptual Feature based Music Classification - A DSP Perspective for a New Type of Application H. Blume, M. Haller M. Botteck, W. Theimer Chair for Electrical Engineering and Computer Systems Nokia Research Center RWTH Aachen University Meesmannstr. 103, Schinkelstraße 2, 52062 Aachen, Germany 44807 Bochum, Germany {blume,haller}@eecs.rwth-aachen.de {martin.botteck,wolfgang.theimer}@nokia.com Abstract — Today, more and more computational power is popular music portals (AMG allmusic, amazon, mp3.com): available not only in desktop computers but also in portable these three share only 70 common genre names of several devices such as smart phones or PDAs. At the same time the hundred available at each portal. availability of huge non-volatile storage capacities (flash memory etc.) suggests to maintain huge music databases even in mobile As of today, genre classification data needs to be created devices. Automated music classification promises to allow manually at some point in time and is subject to corruption keeping a much better overview on huge data bases for the user. upon transfer of the collection from one device to another. In Such a classification enables the user to sort the available huge the absence of a common genre taxonomy an automated music archives according to different genres which can be either classification approach promises to take into account individual predefined or user defined. It is typically based on a set of listening habits and preferences. Such classification needs to perceptual features which are extracted from the music data. rely on how the music sounds rather than how it is named in Feature extraction and subsequent music classification are very order to provide desired listening experiences. The main computational intensive tasks. Today, a variety of music features process of music classification is depicted in Fig. 1. and possible classification algorithms optimized for various application scenarios and achieving different classification Genre 1 qualities are under discussion. In this paper results concerning the computational needs and the achievable classification rates on Genre 2 different processor architectures are presented. The inspected Music- Feature Classifi- Database Extraction cation processors include a general purpose P IV dual core processor, Genre 3 heterogeneous digital signal processor architectures like a Digital Signal Processor Nomadik STn8810 (featuring a smart audio accelerator, SAA) as Genre N well as an OMAP2420. In order to increase classification performance, different forms of feature selection strategies Figure 1. Principle of perceptual feature based classification (heuristic selection, full search and Mann-Whitney-Test) are applied. Furthermore, the potential of a hardware-based A variety of algorithms for this purpose has been developed acceleration for this class of application is inspected by ranging from data mining machine learning approaches [8] to performing a fine as well as a coarse grain instruction tree techniques known from speech recognition [1]. A good analysis. Instruction trees are identified, which could be overview of existing approaches can be found in [19], latest attractively implemented as custom instructions speeding up this developments are evaluated against each other in the MIREX class of applications. contest [9]. All these algorithms impose serious requirements on computation power moving huge amounts of data. Up to Keywords — music information retrieval, music classification, now, their implementation on mobile end-user hardware has feature extraction, processor performance, processor architecture not been studied. optimization, ASIP The work presented here is based on a study of the computational effort for simplified but representative I. INTRODUCTION classification use cases. Three major approaches were Listening to music from digital storage becomes ever more identified to reduce these needs: popular. Storage capacity available in current mobile and portable devices increased dramatically; future devices will • On algorithmic level an optimal selection of the set of provide even larger memories. Consequently, users carry perceptual features used for classification is around huge music collections containing thousands of tracks. investigated. Here, performing a parameter full search Maintaining overview on such collections becomes a difficult as well as a statistical approach (Mann-Whitney-Test) undertaking. Common approaches attempt to sort musical derives the most significant music features for content by "genre" thus expressing commonalities between classification purposes. tracks. Albeit the definitions for "genre" as well as the • On software implementation level all available boundaries between them lack a common taxonomy. [10] and processor specific code optimization techniques (e.g. [14] describe in detail the existing genre diversity analyzing use of specific custom instructions) are discussed. 978-1-4244-1985-2/08/$25.00 ©2008 IEEE 92 Furthermore, the impact of reducing computation word (denoted as feature F19) is defined in the frequency domain. length from floating-point to fixed-point on compu- S(k) is computed using the amplitude spectrum A(k) = |X(k)| tational performance as well as on classification quality with X(k) being the discrete Fourier transform of x(n) is inspected. ⎛ 1 2 ⎞ • On processor architecture level the design of an kS ⋅= log10)( 10 ⎜ kA )( ⎟ . (2) ⎝ N ⎠ application specific instruction set processor (ASIP) is explored. Therefore, the results of a fine grain as well as TABLE I. PERCEPTUAL FEATURES DEPLOYED a coarse grain instruction tree analysis are presented thus identifying the optimization potential as well as the No. Feature design baseline for such a processor. F1 discrete spectrum (computed by FFT) F2 autocorrelation (performed in time domain) The paper is organized as follows: Chapter II introduces F3 autocorrelation (performed in frequency domain) some basics on algorithms for machine based music locating the first three maxima of the F4 classification. Chapter III shortly sketches the hardware autocorrelation platforms deployed in the investigation. The following chapter F5 root mean square describes the music classification experiments used for F6 low energy windows reference. Chapter V discusses the results of a computation F7 sum of correlated components time analysis of these experiments and elaborates possible F8 zero crossing rate algorithmic optimizations like e.g. optimization technique for F9 relative periodicity amplitude peaks significant feature identification. Chapter VI quantifies possible F10 ratio of second and first periodicity peak performance gains through identification of instruction trees F11 fundamental frequency which could be implemented as custom instructions. Finally, a F12 magnitude of spectrum conclusion is given in chapter VII. F13 MFCC F14 spectral centroid II. BASICS OF FEATURE BASED MUSIC CLASSIFICATION F15 spectral rolloff Automatically evaluating similarities in listening F16 spectral extend experience between music tracks implies (at least) two F17 amplitude of spectrum processing steps (see Fig. 1): F18 spectral flux F19 power spectrum • compute music features based on technical definitions F20 spectral kurtosis of music properties (feature extraction) and F21 spectral bandwidth F22 position of main peaks • evaluate similarities according to given sets F23 chroma vector (classification). F24 amplitude of maximum in chromagram pitch interval between two max peaks in The entire automated music classification is based on the F25 assumption that specific listening experiences somehow chromagram translate to properties expressed in technical terms. And, Classifying the music tracks by perceptual features requires indeed, there is e.g. a resemblance between musical rhythm pre-determining feature values for the target category: A subset patterns and certain properties of the autocorrelation function – of tracks needs to be selected and categorized manually however inexact this may be [15]. Characteristic sounds will (training phase). This "ground truth" will determine common somehow determine signal spectral shape as well as influence properties of the desired category and determine which feature the Cepstral Coefficients on a Mel frequency scale (MFCC) specifically leads to a track not being part of that category. [18, 11]. Tab. I contains a list of such features, i.e. the ones Then, classification processing can be brought down to which have been inspected in the course of the experiments computing the Euclidian distance between feature vectors and discussed here. Several more complex ones [19, 1, 11] have selecting suitably close ones. According to the rules of a also been proposed. classifier algorithm a song is assigned to the most common Two exemplary features shall be shortly sketched here: The category amongst its neighbors. Actually, a variety of classifier algorithms has been introduced [8, 19] in order to subdivide the zero-crossing rate (rzero-crossing, denoted as feature F8) provides a rough estimate of the dominating frequency within a time feature vector design space and to assign a new entry to one of window based on the number of signal sign changes by: the existing feature classes (genres). Some of the classifiers discussed in literature and referenced in this work are listed in 1 N −2 Tab. II. r = ⋅ nx −+ nx )(sgn)1(sgn (1) − ingcrosszero ∑ ()N − 12 n=0 In the course of this work performance and computational requirements of the
Details
-
File Typepdf
-
Upload Time-
-
Content LanguagesEnglish
-
Upload UserAnonymous/Not logged-in
-
File Pages8 Page
-
File Size-