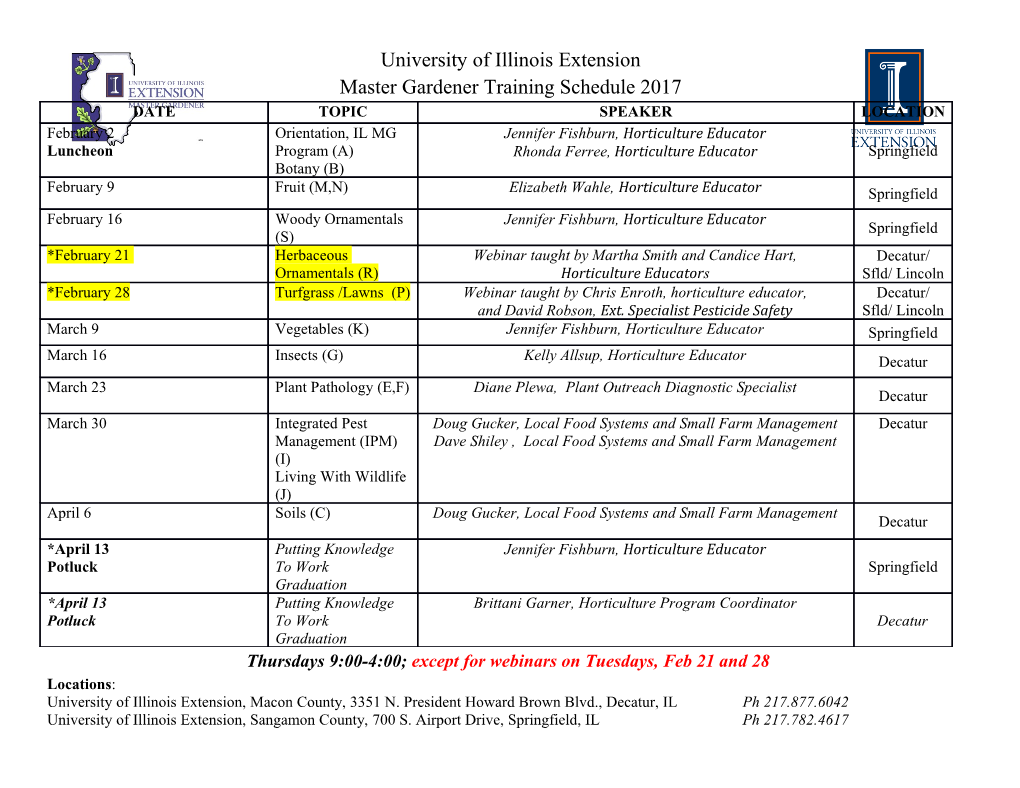
Supporting Information for Mackenzie et al., “Tertiary Alphabet for the Observable Protein Structural Universe” SI Methods strongly correlate, resulting in shallow angles and large positive average cosines. Fig. S1 shows this general behavior, except that it also exhibits some oscillations RMSD Cutoff Derivation before it converges to zero. These oscillations have to do with a characteristic length of secondary structural elements (SSEs) separated by turns and loops, such Fundamentally, optimal rigid-body superposition is an instance of linear that within 15-30 residues a chain is expected to dramatically turn, often in regression, whose goal is to minimize the sum squared deviation (SSD) between almost the opposite direction. In fact, the overall curve fits very closely to a corresponding coordinates of two sets of atoms. Regression quality is typically product of exponential decay (due to the chain “forgetting” its direction) and a assessed using the mean squared error (MSE), an estimate of the error variance, sinusoid (from SSE-related oscillations), as shown in Fig. S1. Specifically, the computed as SSD �, where � is the number of degrees of freedom. The RMSD equation we used was exp − � � ∙ cos � ∙ 2� � , where � is the separation (in metric used in structural superposition is similar to the square root of MSE, but residues), � is the characteristic persistence length, and � is the period of uses the number of atoms in place of �. This is statistically justified only when oscillations. Optimally fitting parameters were � ≈ 33 and � ≈ 10 residues. atoms are entirely independent of each other, which is clearly not the case in Based on this, we chose to use a correlation length parameter for our RMSD general. For example, consider comparing two contiguous segments of structure. cutoff function in the range between 15 to 25 residues (corresponding to a drop- Residues consecutive in sequence are joined by peptide bonds of relatively fixed off in the exponential component to ~0.2 and ~0.1, respectively). Specifically, we geometry, so that the position of each next residue in a chain is highly tested all combinations of correlation lengths 15, 20, and 25 with resolution constrained by the previous residues (e.g., Cα-to-Cα distances between adjacent parameters of 0.9 Å, 1.0 Å, and 1.1 Å. From our initial sequence recovery results, residues are generally ~3.8 Å). On the other hand, two residues that are either it appeared that a correlation length of 20 with a resolution of 1.0 Å produced distant in sequence or not part of the same chain more closely resemble best results, although results from all combinations were similar. We thus chose independent data points. We thus reasoned that to formulate a systematic RMSD the RMSD cutoff function based on � ≈ 10 and resolution of 1.0 Å for our final cutoff function, we must consider how the effective number of degrees of set cover and most other experiments in this study. freedom changes with structure size and topology. Using the PDB, we found that a typical protein chain “forgets” its direction only after ~20 residues (see section Effective Number of Degrees of Freedom “Persistence Length” below and Fig. S1), suggesting that positions of residues separated by fewer amino acids would be expected to be significantly inter- Let us consider a structural query composed of � disjoint segments, with the �-th constrained. Considering residues not required to be part of the same chain as segment of length �!. Our goal is to estimate the number of degrees of freedom completely independent of each other, we then estimated the total effective in such a structure, �. Based on the analysis in the section above, we shall number of degrees of freedom in a structural motif as: assume that two residues in the same chain possess some amount of positional !!!! !! “correlation” that decays exponentially with sequence separation, while residue 2 � = � 1 − � !!! ! (S1) pairs in different chains are completely independent. The average degree of � � − 1 ! !!! !!!!! correlation across all residue pairs in the structure is then: ! !!!! !! !!! ! where the first sum extends over disjoint segments of the motif, � is the length !!! !!! !!!!! � ! � = of the �-th segment, � is the total length of the motif (i.e., � = ! �!), and � is a � � − 1 2 correlation length parameter emergent from the above observation of positional where � !!! ! is the correlation between positions � and � of the same segment, correlation in protein chains (see section “Effective Number of Degrees of with � being a correlation length parameter, whereas a correlation value of zero Freedom” below for a derivation). Notice that � ranges between zero and �. Zero is assumed for residue pairs across different chains (and these are thus skipped in is reached when the correlation length � is very large (infinite), meaning that the summation). An aggregate parameter that describes the overall amount of positions of all residues are highly inter-correlated and no degrees of freedom inter-dependence, � varies from 0 to 1 corresponding to all residues in the remain. On the other hand, the value of � is approached either for very short structure being either fully independent (i.e., � = �) or fully dependent (i.e., correlation lengths or very long segment lengths. In these cases, each residue is � = 0) of each other, respectively. We thus express � as � 1 − � or: effectively independent of the bulk of other residues, such that (nearly) all !!!! !! 2 degrees of freedom are preserved. Given Eq. S1, we can now establish a � = � 1 − � !!! ! � � − 1 ! “universal” RMSD metric ��� �, in place of the traditional RMSD, ��� �. !!! !!!!! Then, if we choose a specific cutoff in terms of our universal metric, �!"#, the When applying this to TERMs, we treated disjoint segments as separate chains. corresponding traditional RMSD cutoff for a given TERM � becomes: Given this formulation for the number of degrees of freedom, the modified � � = �!"# � � � � (S2) universal RMSD metric follows readily as detailed above. We note that this formulation is entirely empirical. However, we have found the resulting This value is no larger than �!"# itself, being much smaller than �!"# for functional form for an RMSD cutoff to work very well in practice, returning small/simple TERMs (i.e., when � ≪ � ), and approaching �!"# for match ensembles that generally agree well with our intuition on structural large/complex TERMs (e.g., those with many segments) as � approaches �. similarity across a large range of motif sizes and complexities (Fig. S2). Thus, �!"# can be thought of as a resolution parameter (i.e., the RMSD cutoff imposed in the limit of large structures), with Eq. S2 computing the RMSD TERM Secondary Structure Classification cutoff for a structure of any finite size. Secondary-structural (SS) assignments were used to analyze TERM diversity and Persistence Length to generate representative sets of TERMs across different SSE classes. First, STRIDE was used to determine the SS class of each residue (1): helix (including We sought to determine how quickly the protein chain in a typical folded STRIDE’s α-helix, 3-10 helix, and pi-helix classes; H), strand (including structure “forgets” its direction. Towards this end, we defined a chain tangent STRIDE’s extended and isolated β-bridge classes; E), turn (T), or coil (C). Next, vector at each residue � to be along the largest principal axis of the local each residue of a TERM segment was assigned an SS class based on the majority backbone window (backbone atoms from residues � through � + 5; sign of vector of residue assignments in the surrounding three-residue window. If there was no chosen to align with the N-to-C direction of the window—i.e., last minus first majority, the residue maintained its original class. The SS class of an entire backbone atom). Then, for all pairs of residues in a protein, the cosine of the segment was then assigned as a string of concatenated residue SS classes, with angle between their respective chain tangent vectors, ��� � , was computed. consecutive repeats removed SS. For example, a six residue segment with two Having done this for all chains in DB80, we plotted the average ��� � as a turn residues followed by four helix residues, TTHHHH, would be represented as function of inter-residue separation, shown in Fig. S1. At large separations there the string TH. Finally, a TERM’s SS class was represented with the set of its should be no relationship between chain tangent vectors (i.e., chain direction is segment classifications. For example a two-segment beta sheet TERM would be fully randomized after a certain number of amino acids), such that the average represented as {E,E}, a two-segment TERM with turn-helix and helix segments cosine should be zero. Whereas at short separations, chain directions should as {TH, H} and a three-segment TERM with a beta sheet and a helix as {E,E,H}. Two TERMs were considered to share a secondary-structure class if they were average. In particular, if �!" is the set of all TERMs that cover ��(�, �), with the assigned identical sets. positions of TERM � ∈ �!" aligned with � and � being �! and �!, respectively, then the pair enrichment was calculated as: � (� , � )� �, �, � , � Metal and Water Binding Motifs !∈!!" ! ! ! ! ! ! � �, �, �, � = (S10) � (� , � ) TERMs for which at least � % of matches interacted with a metal of interest (i.e., !∈!!" ! ! ! where � (� , � ) is the weight assigned to TERM �, in conjunction with had a non-hydrogen atom within 3 Å of the metal
Details
-
File Typepdf
-
Upload Time-
-
Content LanguagesEnglish
-
Upload UserAnonymous/Not logged-in
-
File Pages14 Page
-
File Size-