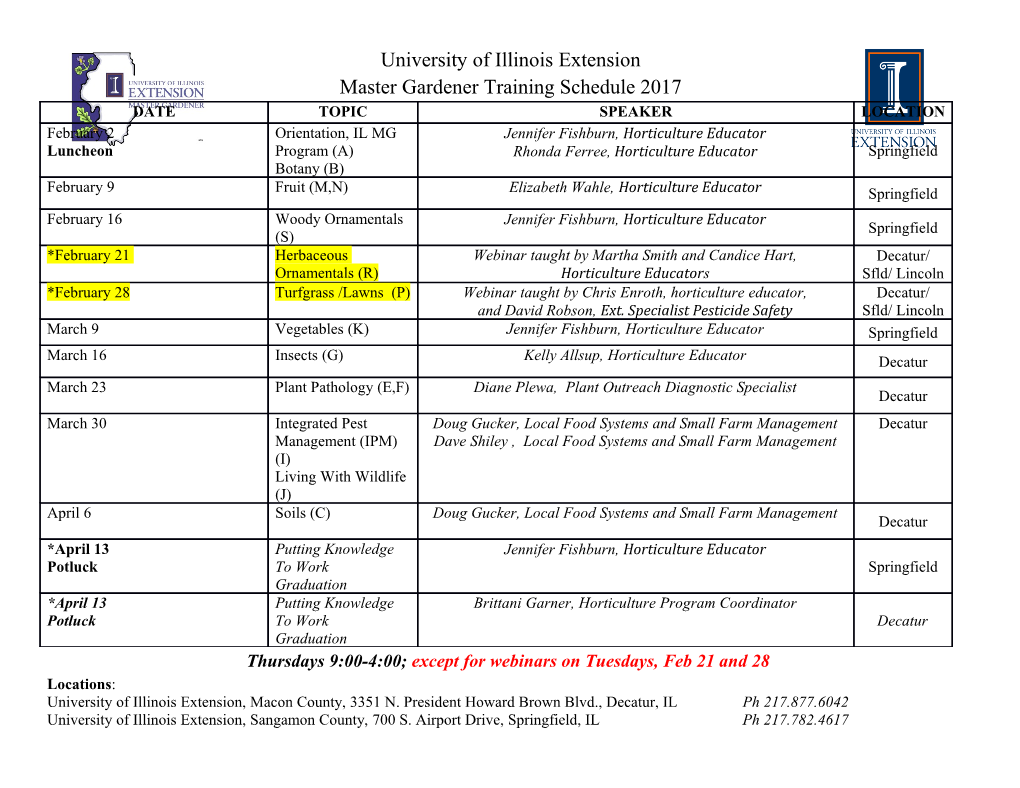
LETTER doi:10.1038/nature13827 Long-term phenotypic evolution of bacteria Germa´n Plata1,2, Christopher S. Henry3 & Dennis Vitkup1,4 For many decades comparative analyses of protein sequences and is sometimes observed within the same species, a transition from high structures have been used to investigate fundamental principles of to low phenotypic similarity occurs primarily at the genus level. molecular evolution1,2. In contrast, relatively little is known about Analyses of phenotypic evolution, such as the morphological varia- the long-term evolution of species’ phenotypic and genetic properties. tion of beaks in Darwin’s finches3, provided the original impetus and This represents an important gap in our understanding of evolu- context for understanding natural selection. Because the evolutionary tion, as exactly these proprieties play key roles in natural selection importance and physiological role of specific phenotypic traits change and adaptation to diverse environments. Here we perform a com- over time, it is often difficult to connect genotype to phenotype to fit- parative analysis of bacterial growth and gene deletion phenotypes ness across long evolutionary distances, especially for metazoan organ- using hundreds of genome-scale metabolic models. Overall, bacte- isms. For microbial species, on the other hand, the ability to metabolize rial phenotypic evolution can be described by a two-stage process different nutrient sources, although clearly not the only important phe- with a rapid initial phenotypic diversification followed by a slow notype, always remains an essential determinant of their fitness and long-term exponential divergence. The observed average divergence lifestyle. Even though a large-scale comparative analysis of microbial trend, with approximately similar fractions of phenotypic properties phenotypes—such as growth on different nutrients or the impact of changing per unit time, continues for billions of years. We experimen- genetic perturbations—is currently challenging owing to a relative pau- tally confirm the predicted divergence trend using the phenotypic city of experimental data, we rationalized that thoroughly validated com- profiles of 40 diverse bacterial species across more than 60 growth putational methods can be used to investigate the phenotypic evolution conditions. Our analysissuggests that, atlong evolutionary distances, of diverse bacterial species. Flux balance analysis (FBA)4, in particular, gene essentiality is significantly more conserved than the ability to has been previously used to accurately predict gene and nutrient essen- utilize different nutrients, while syntheticlethality issignificantly less tiality, growth yields, and evolutionary adaptations to environmental conserved. We also find that although a rapid phenotypic evolution and genetic perturbations5. Notably, the accuracy of FBA methods has Pseudoalteromonadaceae (2) Alphaproteobacteria Psychromonadaceae (1) Idiomarinaceae (1) Shewanellaceae (9) Betaproteobacteria Vibrionaceae (11) Pseudomo Piscirickettsiaceae (1)P asteurellaceae (7) Gammaproteobacteria ae (1) Alte Legionellaceae (4) Ectothiorhodospiraceaer (1) Deltaproteobacteria omonadaceae (1) M nada o e (1) Francisellaceraxellaceae (10) Epsilonproteobacteria ceae (10) ) Methyloc (1 Coxiellac Methanosarcinaceae (1) Aquificaceae (3) Thermotogaceae (1) Actinobacteria Deinococcaceae (2) Thermaceae (1) Bifidobacteriaceae (1) ae (2) Streptomycetaceae (3) cetaceae (1) Chromatiaceaeoccaceae (2) (1) Pseudonocardiace Bacilli Burkholderiaceae (28) Mycobacteriaceae (6) ales Family III eae (1) Nocardiaceae (1) eriaceae (2) Frankiaceae (1) t Micromonosporacea Bacteroidetes Alcalige Actinomy Oxalobacteraceae (1) Kineosporiaceae (1) Hydrogenophilaceaenaceae (1) (3) Microbac Clostridia Thermoanaerobacteraceae (3)VIII (1) Thermoanaerobacter Comamonadaceae (9) Rubrobacteraceae (1) Deinococci Alicyclobacillaceae (1) Rhodocyclaceae (1) Clostridiales Family X Mollicutes Gallionellaceae (1) Acholeplasmataceae (1) Mycoplasmataceae (2) Spirochaetales Methylophilaceae (1) Peptostreptococcaceae (1) Neisseriaceae (9) Clostridiaceae (10) Ruminococcaceae (1) Other Nitrosomonadaceae (1) Veillonellaceae (1) Streptococcaceae (18) Cardiobacteriaceae (1) Leuconostocaceae (1) Xanthomonadaceae (2) Lactobacillaceae (2) Enterococcaceae (2) Acidithiobacillaceae (1) Listeriaceae (4) Brucellaceae (1) B Staphylococcaceaeacillaceae (20) (5) Bartonellaceae (1) Elusimicr Chlamydiaceae (2) Rhizobiaceae (4) Lept Rhodobiaceae (1) Spirochaetaceaeobiaceae (1) Prochlorocospiraceaeoccaceae (2) (2) Fu Beijerinckiaceae (1) Rhodotherm Chlorobiaceaes (4) Cytophagacea o Flavobacteriaceae (1) b Bacteroidaceae (1) acteriaceae Xanthobacteraceae (2) Nautiliaceae Porphyromonadaceae (1) (2) Campylobacteraceae (7) Helicobacteraceae (11) Deferribacteraceae (1) Desulfohalobiaceae (1) (2) Methylobacteriaceae (3) Bradyrhizobiaceae (4) a cea Rhodospirillaceae (2) (1 e ( ) e 1) SphingomonadaceaeRickettsiaceae (2) (1) (1) Erythrobacteraceae (1) RhodobacterAcetobacteraceaeaceae (15) (8) Anaplasmataceae (3) Bdellovibrionaceae (1)Myxococcaceae (1) Geobacteraceae (2) Syntrophobacteraceae (1) Desulfobacteraceae (1) Desulfovibrionaceae (2) Desulfomicrobiaceae (1) Figure 1 | Diversity of considered bacterial families. The cladogram shows (see Methods). The numbers of considered species in each family are shown in the evolutionary relationship between the 100 bacterial families that include the parentheses. Different colours represent different bacterial classes. The tree 322 species considered in our study. The tree is based on the average 16S was rooted using the Methanosarcina barkeri rRNA sequence. ribosomal RNA (rRNA) genetic distances between species in each family 1Department of Systems Biology, Center for Computational Biology and Bioinformatics, Columbia University, New York, New York 10032, USA. 2Integrated Program in Cellular, Molecular, Structural and Genetic Studies, Columbia University, New York, New York 10032, USA. 3Mathemathics and Computer Science Division, Argonne National Laboratory, Argonne, Illinois 60439, USA. 4Department of Biomedical Informatics, Columbia University, New York, New York 10032, USA. 00 MONTH 2014 | VOL 000 | NATURE | 1 ©2014 Macmillan Publishers Limited. All rights reserved RESEARCH LETTER been independently demonstrated for many dozens of species encom- evolution (,0.2 genetic distance in Fig. 2), the divergence of growth passing diverse phylogenetic distributions and growth environments6. phenotypes approaches saturation around a similarity of 21% (Fig. 2a), We selected for our analysis more than 300 phylogenetically diverse which is higher than the value expected by chance (,12%) given the bacteria (Fig. 1) for which genome-scale metabolic models were recon- average number of carbon compounds used by the models. This dif- structed using a recently developed protocol7 (see Methods). ference is likely due to a widespread utilization of common nutrient Toinvestigate the long-term evolutionof growth phenotypes, we con- sources across bacterial species (see Extended Data Table 3)10. sidered 62 carbon sources that are commonly used by microbial species Notably, before the evolution of growth phenotypes settles into the for growth and energy production8. For each considered species we used aforementioned average trend, a much higher rate of phenotypic evo- FBA to determine a subset of the compounds that could be used for lution is observed for pairs of bacteria at very close genetic distances biomass synthesis or ATP generation—two key metabolic objectives of (,0.01, or ,50 million years11). Our computational analysis predicts bacterial growth9. This analysis resulted in binary phenotypic profiles ,71% phenotypic similarity for closely related bacteria (Fig. 2, Extended that describe the ability of each microbial species to use each of the con- Data Table 1), which agrees well with available experimental data on intra- sidered compounds (see Methods). The evolution of these phenotypic species phenotypic similarity: for example, 75% for the utilization of profiles—that is, the change in phenotypic similarity as a function of spe- carbon sources in Escherichia coli12 and 69% for Campylobacter jejuni13. cies divergence (genetic distance)—is shown in Fig. 2a, (see also Extended The diversity of bacteria observed at close distances reflects a well- Data Fig. 1). Notably, this analysis demonstrated that the average long- documented genetic and phenotypic variability within bacterial pan- term evolution of growth phenotypes can be approximated well by an genomes14. The observed patterns also suggest that phenotypic evolution exponential decay (see Methods and Extended Data Table 1). A three- proceeds in two different stages, namely through fast phenotypic diversifi- parameter exponential model fits the data in Fig. 2a significantly better cation of closely related strains followed by a slower exponential divergence than simpler alternative models (Extended Data Table 2). Similar diver- lastingbillions of years. Notably, patterns of multi-stage and hierarchical gence trends were observed for larger sets of carbon source compounds evolution have been observed in other systems, for example in bacterial (Extended Data Fig. 2), and for compounds that could be used as a and eukaryotic developmental networks15,16. source of nitrogen (Fig. 2b). The observed trend was also robust towards To validate experimentally the predicted patterns of long-term phe- subsampling or removal of specific species and families used
Details
-
File Typepdf
-
Upload Time-
-
Content LanguagesEnglish
-
Upload UserAnonymous/Not logged-in
-
File Pages16 Page
-
File Size-