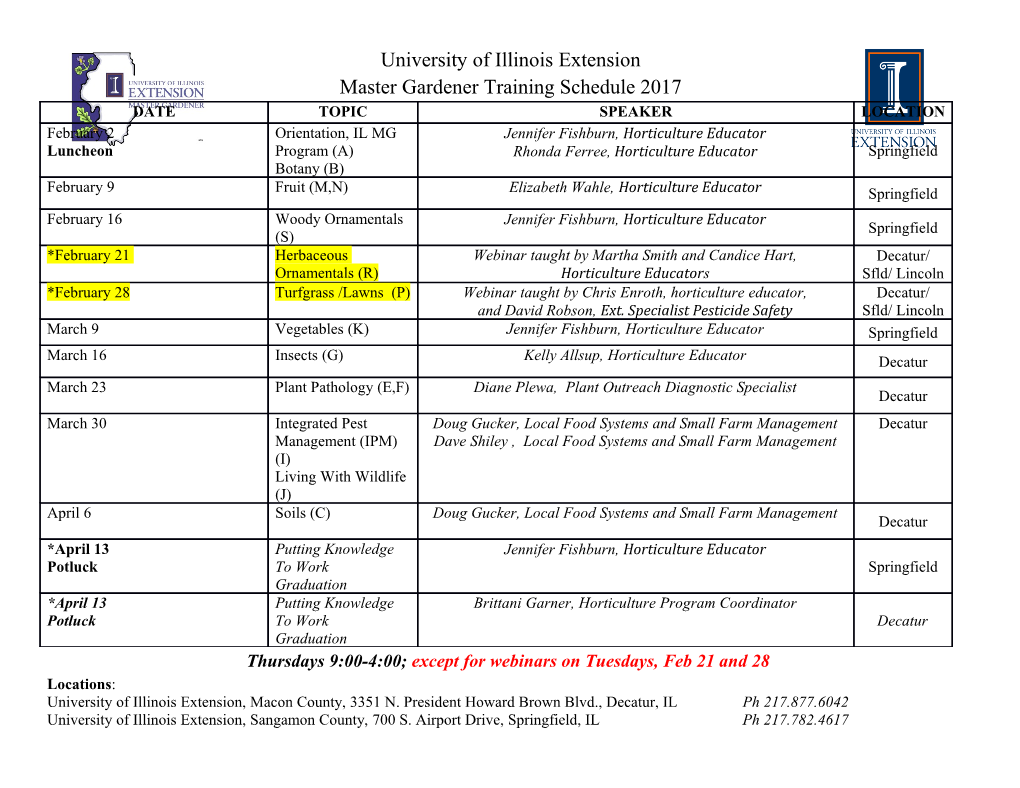
Statistical methods for predicting genetic regulation by Nisar Ahmed Shar Submitted in accordance with the requirements for the degree of Doctor of Philosophy The University of Leeds in the Faculty of Biological Sciences School of Molecular and Cellular Biology November 2016 ii Intellectual Property and Publication Statements The candidate confirms that the work submitted is his own, except where work which has formed part of jointly authored publications has been included. The contribution of the candidate and other authors to this work has been explicitly indicated below. The candidate confirms that appropriate credit has been given within the thesis where reference has been made to the work of others. The work in Chapter 5 and 6 of the thesis has appeared in publication as follows Shar, Nisar A., M. S. Vijayabaskar, and David R. Westhead. "Cancer somatic mutations cluster in a subset of regulatory sites predicted from the ENCODE data." Molecular Cancer 15.1 (2016): 76. I was responsible for carrying out this study and writing the paper along with the David R. Westhead. M.S. Vijayabaskar helped with data analysis, paper writing and supervision of the work. David R. Westhead conceived and designed the study. This copy has been supplied on the understanding that it is copyright material and that no quotation from the thesis may be published without proper acknowledgement. © 2016 The University of Leeds and Nisar Ahmed Shar The right of Nisar Ahmed Shar to be identified as Author of this work has been asserted by his in accordance with the copyright, Designs and Patents Act 1988. iii Acknowledgements First and foremost, I would like to thank my supervisor, David Westhead, for huge support, guidance and unending patience. He has been very kind and approachable throughout my PhD and I was lucky to have learned supervisor like him. Dave has been encouraging and kind to me whenever I get confused and frustrated. I would like to thank everybody in the laboratory specially Francis, Fatin and Chulin for providing moral support and a good company whenever I needed. A special thanks to Vijaya Baskar and Mathew Care for helping me during PhD. A big thanks to my family for their unending support and understanding my limitations being thousands of miles away from me. Lastly, I would like to thank NED university of Engineering & Technology, Karachi and University of Leeds for sponsoring my PhD, and anyone who has helped me in anyway during my PhD duration. iv Abstract Transcriptional regulation of gene expression is essential for cellular differentiation and function, and defects in the process are associated with cancer. Transcription is regulated by the cis-acting regulatory regions and trans- acting regulatory elements. Transcription factors bind on enhancers and repressors and form complexes by interacting with each other to control the expression of the genes. Understanding the regulation of genes would help us to understand the biological system and can be helpful in identifying therapeutic targets for diseases such as cancer. The ENCODE project has mapped binding sites of many TFs in some important cell types and this project also has mapped DNase I hypersensitivity sites across the cell types. Predicting transcription factors mutual interactions would help us in finding the potential transcription regulatory networks. Here, we have developed two methods for prediction of transcription factors mutual interactions from ENCODE ChIP-seq data, and both methods generated similar results which tell us about the accuracy of the methods. It is known that functional regions of genome are conserved and here we identified that shared/overlapping transcription factor binding sites in multiple cell types and in transcription factors pairs are more conserved than their respective non-shared/non-overlapping binding sites. It has been also studied that co-binding sites influence the expression level of genes. Most of the genes mapped to the transcription factor co-binding sites have significantly higher level of expression than those genes which were mapped to the single transcription factor bound sites. The ENCODE data suggests a very large number of potential regulatory sites across the complete genome in many cell types and methods are needed to identify those that are most relevant and to connect them to the genes that they control. A penalized regression method, LASSO was used to build correlative models, and choose two regulatory regions that are predictive of gene expression, and link them to their respective gene. Here, we show that our identified regulatory regions accumulate significant number of somatic mutations that occur in cancer cells, suggesting that their effects may drive cancer initiation and development. Harboring of somatic v mutations in these identified regulatory regions is an indication of positive selection, which has been also observed in cancer related genes. vi Table of Contents Publications ii Acknowledgements iii Abstract iv Contents vi List of Figures x List of Tables xii Abbreviations xiii 1 Introduction .................................................................................................... 1 1.1 Overview of Molecular Biology .................................................................. 1 1.1.1 Gene regulation ................................................................................... 1 1.1.2 Transcription factors ............................................................................ 4 1.1.3 Cis-acting regulatory regions ............................................................... 6 1.1.4 Chromatin region ................................................................................. 7 1.1.4.1 Histone modifications .................................................................... 7 1.1.4.2 Chromatin looping ......................................................................... 8 1.1.4.3 Epigenetic regulation/ DNA methylation ........................................ 9 1.2 Available data ............................................................................................ 9 1.2.1 ENCODE ............................................................................................. 9 1.2.1.1 ChIP-seq ..................................................................................... 11 1.2.1.2 DNase-seq .................................................................................. 14 1.2.1.3 RNA-seq ...................................................................................... 16 1.2.2 Conservation data ............................................................................. 18 1.3 Cancer ..................................................................................................... 19 1.4 Statistical methods of machine learning .................................................. 20 1.5 Thesis objectives and structure ............................................................... 21 2 Preliminary studies of potential methods for predicting transcription factor interactions by binding site overlap analysis .................................... 23 2.1 Introduction .............................................................................................. 23 2.1.1 TF Co-association ............................................................................. 23 2.1.2 Distinguishing indirect transcription factor occupancy ....................... 25 2.2 Methods and data .................................................................................... 26 vii 2.2.1 Dataset .............................................................................................. 26 2.2.2 Methods ............................................................................................. 27 2.2.2.1 Randomisation ............................................................................ 29 2.2.2.2 Poisson distribution ..................................................................... 30 2.2.2.3 Optimisation of method ............................................................... 31 2.2.2.4 Multiple testing correction............................................................ 31 2.2.2.5 Validation of method ................................................................... 32 2.3 Results ..................................................................................................... 32 2.3.1 Gm12878 cell type............................................................................. 32 2.3.1.1 Intersection (overlapping) of transcription factors ........................ 33 2.3.1.2 Statistical significance of the overlaps. ........................................ 34 2.3.1.3 Optimised results ........................................................................ 35 2.3.1.4 Validation (Comparison of significant overlaps and known protein- protein interactions) ................................................................................. 40 2.3.2 K562 cell type .................................................................................... 43 2.3.2.1 Optimised results for K562 cell type ............................................ 43 2.3.2.2 Validation (Comparison of significant overlaps and known protein- protein interactions) ................................................................................. 49 2.4 Discussion ............................................................................................... 52 3 Conservation analyses of transcription factor binding sites and effect of co-binding sites on gene expression
Details
-
File Typepdf
-
Upload Time-
-
Content LanguagesEnglish
-
Upload UserAnonymous/Not logged-in
-
File Pages197 Page
-
File Size-