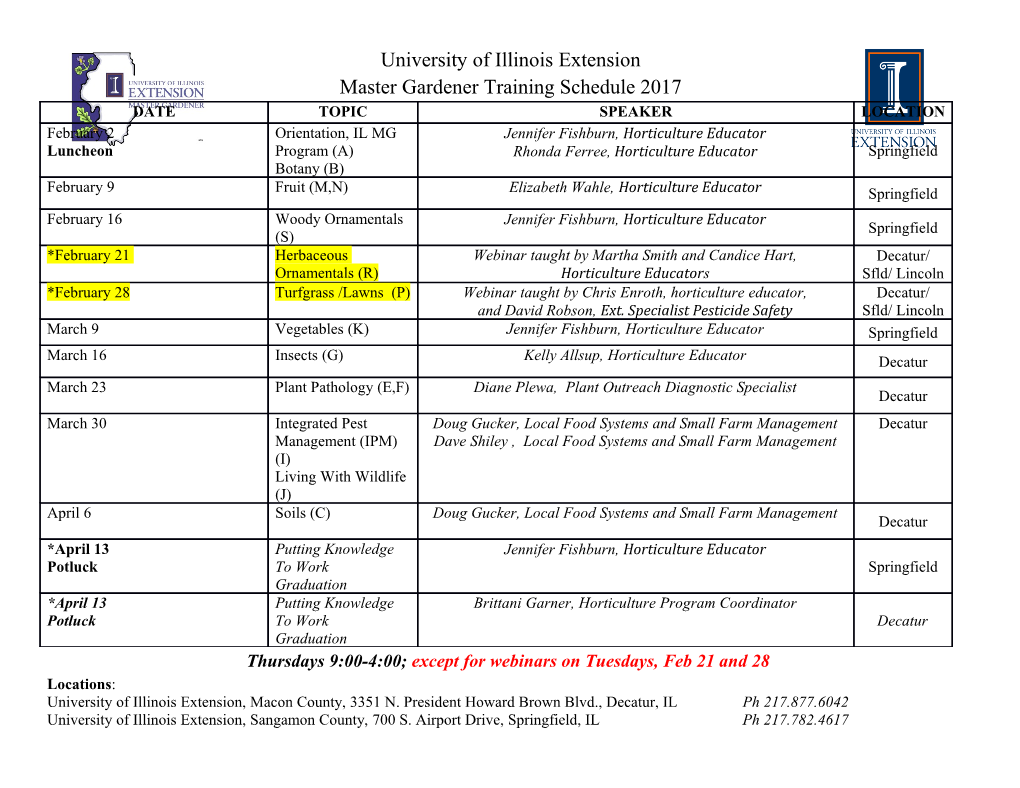
Zurich Open Repository and Archive University of Zurich Main Library Strickhofstrasse 39 CH-8057 Zurich www.zora.uzh.ch Year: 2019 Wiring the Microbial Web of Earth – New Approaches for Scalable Computational Prediction of Interpretable Microbial Ecosystem Structure Tackmann, Janko Abstract: Microorganisms are the principal biotic driver of life on Earth. They shape virtually every aspect of the planet’s biosphere, through both the maintenance of global biogeochemical cycles and via essential symbiotic relationships with multi-cellular organisms. Questions related to how individual mi- crobial species form interacting communities (ecosystems)—with a drastic impact on their hosts and environments— are being studied with rapidly accelerating intensity by the Microbial Ecology field. En- abled through recent innovations in sequencing technologies, staggering amounts of knowledge are lately being generated, which already yielded fascinating insights: microorganisms have now been identified in even the most extreme environments, all across the globe, and intriguing connections between hosts and their microbiota are continuously being discovered, including for instance links to disease develop- ment and host behavior. So far, insights have mainly been gained through comparatively straightfor- ward, descriptive analyses of static microbial community snapshots. Such workflows employ for instance diversity-based comparisons of community profiles or the identification of community members thatare strongly associated with a condition of interest (e.g. a disease or lifestyle factor). Less research has how- ever focused on disentangling the underlying interaction structures, which dictate ecosystem dynamics and thus ultimately mold the observed community patterns. Elucidating these complex relationships would allow a system-level understanding of microbial communities and inform experiments aimed at mechanistic understanding. Unfortunately, experimental validation of ecological interactions is currently impossible for all but the smallest communities and is furthermore restricted to microbes culturable under laboratory conditions, which constitute only a tiny fraction of the known diversity. Nonetheless, modern quantities of culture-independent microbial sequencing data offer a wealth of information to fuel compu- tational prediction approaches and alleviate these shortcomings. In particular, such data can be mined for statistical co-occurrence or co-avoidance patterns—indicative of positive (mutualist, commensal) or negative (competitive, parasitic, predatory or amensal) ecological interactions—to enable the prediction of microbial interaction network models. Applying this approach to globally distributed sequencing data, covering diverse habitats and conditions, would result in a model of the microbial web of Earth that could allow a first glimpse at global microbial interaction patterns. Throughout the last decade, many methods have been proposed for the statistical prediction of ecological interactions. However, these ap- proaches typically do not account for a variety of artifacts, including for instance shared ecological and environmental dependencies. Such artifacts are particularly widespread in global, heterogeneous data sets and thus severely hamper the ecological interpretation of networks inferred from such data. More- over, current methods generally do not scale to modern (cross-study) sequencing data quantities, which seriously limits the comprehensiveness of predicted models. In this thesis, I present a new approach to address these shortcomings: FlashWeave. The method uses a flexible Probabilistic Graphical Modeling (PGM) framework to infer direct associations. These predictions are depleted of indirect (i.e. spuri- ous) associations and thus enable sparser and more interpretable ecosystem models. In contrast to the majority of current methods, FlashWeave furthermore scales to data sets with hundreds of thousands of samples and can explicitly integrate environmental and technical factors into model inference. We found that FlashWeave outperformed other approaches in recovering the structure of simulated micro- bial ecosystems and, additionally, surpassed them in detecting verified interactions within a real-world data set of marine sequencing samples. We furthermore used FlashWeave to predict the to date largest microbial interaction network of the human gastrointestinal tract, which revealed striking signals of po- tential biological relevance. These include for instance unusually pronounced phylogenetic assortativity, extensive interactions within the rare biosphere and novel mutualist hub species. Moreover, FlashWeave allowed us to infer a global cross-biome interaction network, based on more than half a million sequencing samples that cover highly diverse habitats. In-depth analysis of this network in future studies promises interesting ecological insights. In the second part of this thesis, I present a parallel line of work that demonstrates how biomarker discovery can also strongly benefit from the removal of indirect associa- tions. In this context, spurious associations may appear between microbes and non-microbial variables of interest (driven, for instance, by ecological microbe-microbe interactions) and can result in numerous re- dundant biomarkers, which negatively impact prediction quality and complicate biological interpretation. We found that FlashWeave, applied to the exemplary task of identifying microbes directly association to a selection of human body sites, generated highly parsimonious and interpretable biomarker sets. The resulting biomarkers furthermore yielded outstanding predictive performance on both pure and mixed body site microbiota. The work presented in this thesis is a major step towards a better understanding of global microbial interaction trends, with potential applications for instance in probiotics development, next-generation culturing efforts and ecosystem engineering. It furthermore highlights approaches for more parsimonious and interpretable biomarker discovery, which can be crucial for instance in clinical or forensic applications. Posted at the Zurich Open Repository and Archive, University of Zurich ZORA URL: https://doi.org/10.5167/uzh-189835 Dissertation Published Version The following work is licensed under a Creative Commons: Attribution 4.0 International (CC BY 4.0) License. Originally published at: Tackmann, Janko. Wiring the Microbial Web of Earth – New Approaches for Scalable Computational Prediction of Interpretable Microbial Ecosystem Structure. 2019, University of Zurich, Faculty of Science. 2 Wiring the Microbial Web of Earth – New Approaches for Scalable Computational Prediction of Interpretable Microbial Ecosystem Structure Dissertation zur Erlangung der naturwissenschaftlichen Doktorwürde (Dr. sc. nat.) vorgelegt der Mathematisch-naturwissenschaftlichen Fakultät der Universität Zürich von Janko Tackmann aus Deutschland Promotionskommission Prof. Dr. Christian von Mering (Vorsitz) Prof. Dr. Reinhard Furrer Prof. Dr. Jeroen Raes Zürich, 2019 Licensed under Creative Commons Attribution (CC BY) 4.0 Abstract Microorganisms are the principal biotic driver of life on Earth. They shape virtually every aspect of the planet’s biosphere, through both the maintenance of global biogeochemical cycles and via essential symbiotic relationships with multi-cellular organisms. Questions related to how individual microbial species form interacting communities (ecosystems)—with a drastic impact on their hosts and environments— are being studied with rapidly accelerating intensity by the Microbial Ecology field. Enabled through recent innovations in sequencing technologies, staggering amounts of knowledge are lately being generated, which already yielded fascinating insights: microorganisms have now been identified in even the most extreme environments, all across the globe, and intriguing connections between hosts and their microbiota are continuously being discovered, including for instance links to disease development and host behavior. So far, insights have mainly been gained through comparatively straightforward, descriptive analyses of static microbial community snapshots. Such workflows employ for instance diversity-based comparisons of community profiles or the identification of community members that are strongly associated with a condition of interest (e.g. a disease or lifestyle factor). Less research has however focused on disentangling the underlying interaction structures, which dictate ecosystem dynamics and thus ultimately mold the observed community patterns. Elucidating these complex relationships would allow a system-level understanding of microbial communities and inform experiments aimed at mechanistic understanding. Unfortunately, experimental validation of ecological interactions is currently impossible for all but the smallest communities and is furthermore restricted to microbes culturable under laboratory conditions, which constitute only a tiny fraction of the known diversity. Nonetheless, modern quantities of culture-independent microbial sequencing data offer a wealth of information to fuel computational prediction approaches and alleviate these shortcomings. In particular, such data can be mined for statistical co-occurrence or co-avoidance patterns—indicative of positive (mutualist, commensal) or negative (competitive, parasitic, predatory or amensal) ecological interactions—to enable the prediction of microbial interaction network models. Applying this
Details
-
File Typepdf
-
Upload Time-
-
Content LanguagesEnglish
-
Upload UserAnonymous/Not logged-in
-
File Pages249 Page
-
File Size-