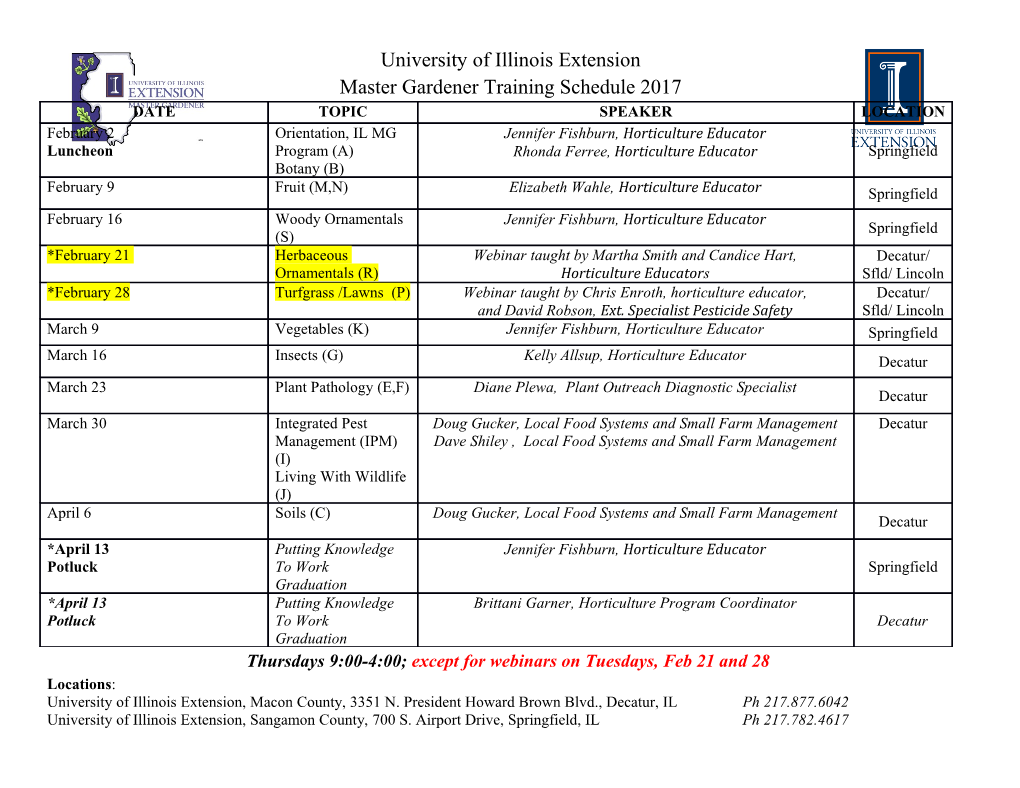
The random geometry of equilibrium phases Hans-Otto Georgii, Mathematisches Institut, Ludwig-Maximilians-Universit¨at, D-80333 M¨unchen, Germany. Email: [email protected] Olle H¨aggstr¨om, Department of Mathematics, Chalmers University of Technology, S-412 96 G¨oteborg, Sweden. Email: [email protected] Christian Maes, Instituut voor Theoretische Fysica, K.U.Leuven, B-3001 Leuven, Belgium. Email: [email protected] Contents 1 Introduction 3 2 Equilibrium phases 6 2.1 Thelattice .................................. 6 2.2 Configurations ................................ 6 2.3 Observables.................................. 7 2.4 Randomfields................................. 8 2.5 TheHamiltonian ............................... 8 2.6 Gibbsmeasures................................ 9 2.7 Phasetransitionandphases . 11 arXiv:math/9905031v1 [math.PR] 5 May 1999 3 Some models 14 3.1 TheferromagneticIsingmodel . 14 3.2 TheantiferromagneticIsingmodel . 15 3.3 ThePottsmodel ............................... 16 3.4 Thehard-corelatticegasmodel. 17 3.5 The Widom–Rowlinson lattice model . 18 4 Coupling and stochastic domination 19 4.1 Thecouplinginequality . 19 4.2 Stochasticdomination . 20 4.3 ApplicationstotheIsingmodel . 23 4.4 Applicationtoothermodels . 26 1 5 Percolation 28 5.1 Bernoullipercolation . 28 5.2 Dependent percolation: the role of the density . ....... 32 5.3 Examples of dependent percolation . 34 5.4 Thenumberofinfiniteclusters . 37 6 Random-cluster representations 41 6.1 Random-clusterandPottsmodels . 41 6.2 Infinite-volumelimits. 44 6.3 PhasetransitioninthePottsmodel . 47 6.4 Infinite volume random-cluster measures . ..... 50 6.5 An application to percolation in the Ising model . ...... 55 6.6 Cluster algorithms for computer simulation . ...... 56 6.7 Random-cluster representation of the Widom–Rowlinson model ..... 58 7 Uniqueness and exponential mixing from non-percolation 61 7.1 Disagreementpaths.............................. 61 7.2 Stochastic domination by random-cluster measures . ........ 66 7.3 Exponential mixing at low temperatures . 70 8 Phase transition and percolation 76 8.1 Agreement percolation from phase coexistence . ....... 77 8.2 Plus-clustersfortheIsingferromagnet . ...... 78 8.3 Constant-spin clusters in the Potts model . ..... 82 8.4 Further examples of agreement percolation . ...... 84 8.5 Percolation of ground-energy bonds . 86 9 Random interactions 93 9.1 Diluted and random Ising and Potts ferromagnets . ..... 94 9.2 Mixing properties in the Griffiths regime . 96 10 Continuum models 102 10.1 Continuumpercolation. 102 10.2 The continuum Widom–Rowlinson model . 103 2 1 Introduction Equilibrium statistical mechanics intends to describe and explain the macroscopic be- havior of systems in thermal equilibrium in terms of the microscopic interaction between their great many constituents. As a typical example, let us take some ferromagnetic ma- terial like iron; the constituents are then the spins of elementary magnets at the sites of some crystal lattice. Or we may think of a lattice approximation to a real gas, in which case the constituents are the particle numbers in the elementary cells of any partition of space. The central object is the Hamiltonian describing the interaction between these constituents. This interaction determines the relative energies between configurations that differ only microscopically. The equilibrium states with respect to the given interac- tion are described by the associated Gibbs measures. These are probability measures on the space of configurations which have prescribed conditional probabilities with respect to fixed configurations outside of finite regions. These conditional probabilities are given by the Boltzmann factor, the exponential of the inverse temperature times the relative energy. This allows one to compute, at least in principle, equilibrium expectations and spatial correlation functions following the standard Gibbs formalism. Most important are the so called extremal Gibbs measures since they describe the possible macrostates of our physical system. In such a state, macroscopic observables do not fluctuate while the correlation between local observations made far apart from each other decays to zero. Since the early days of statistical mechanics, geometric notions have played a role in elucidating certain aspects of the theory. This has taken many different forms. Ar- guably, the thermodynamic formalism, as first developed by Gibbs, already admits some geometric interpretations primarily related to convexity. For example, entropy is a concave function of the specific energy, the pressure is convex as a function of the interaction potential, the Legendre–Fenchel transformation relates various fundamental thermodynamic quantities to each other, and the set of Gibbs measures for an interac- tion is a simplex with vertices corresponding to the physically realized macrostates, the equilibrium phases. Here, however, we will not be concerned with this kind of convex geometry which is described in detail e.g. in the books by Israel [138] and Georgii [96]. Rather, the geometry considered here is a way of visualizing the structure in the typical realizations of the system’s constituents. To be more specific let us consider for a moment the case of the standard ferromagnetic Ising model on the square lattice. At each site we have a spin variable taking only two possible values, +1 and 1. The interaction is nearest- − neighbor and tends to align neighboring spins in the same direction. By the ingenious arguments first formulated in 1936 by Peierls [187] (see also [63, 213, 96]), the phase transition in this model can be understood from looking at the typical configurations of contours, i.e., the broken lines separating the domains with plus resp. minus spins. The plus phase (the positively magnetized phase) is realized by an infinite ocean of plus spins with finite islands of minus spins (which in turn may contain lakes of plus spins, and so on). On the other hand, above the Curie temperature (first computed by Onsager) there is no infinite path joining nearest neighbors with the same spin value. So, for this model the geometric picture is rather complete (as we will show later). In general, however, much less is known, and much less is true. Still, certain aspects of this geometric analysis have wide applications, at least in certain regimes of the phase diagram. These ‘certain regimes’ are, on the one hand, the high-temperature (or, in a 3 lattice gas setting, low-density) regime and, to the other extreme, the low temperature behavior. At high temperatures, all thermodynamic considerations are based on the fact that entropy dominates over energy. That is, the interaction between the constituents is not effective enough to enforce a macroscopic ordering of the system. As a result, every constituent is more or less free to behave at random, not much influenced by other constituents which are far apart. So, the system’s behavior is almost like that of a free system with independent components. This means, in particular, that in the center of a large box we will typically encounter more or less the same configurations no matter what boundary conditions outside this box are imposed. That is, if we compare two independent realizations of the system in the box with different boundary conditions outside then, still at high temperatures, the difference between the boundary conditions cannot be felt by the spins in the center of the box; specifically, there should not exist any path from the boundary to the central part of the box along which the spins of the two realizations disagree. This picture is rather robust and can for example also be applied when the interaction is random; see Sections 7 and 9. At low temperatures, or large densities (when the interaction is sufficiently strong), the picture above no longer holds. Rather, the specific characteristics of the interaction will come into play and determine the specific features of the low temperature phase. In many cases, the low temperature behavior can be described as a random perturbation of a ground state, i.e., of a fixed configuration of minimal energy. Then we can expect that at low temperatures, and sometimes even up to the critical temperature, the equilibrium phases are realized as a deterministic ground state configuration, perturbed by finite random islands on which the configuration disagrees with the ground state. This means that the ground state pattern can percolate through the space to infinity. One prominent way of confirming this picture is provided by the so called Pirogov–Sinai theory which is described in detail e.g. in [230]. In Section 8 we will discuss some other techniques of establishing the same geometric picture. It is evident from the above that percolation theory will play an important role in this text. In fact, we will mainly be concerned with dependent percolation, but one can say that independent percolation stands as a prototype for the study of statistical equilibrium properties in geometric terms. In independent percolation, the model is extremely simple: the components are binary-valued and independent from each other. What is hard is the type of question one asks, namely the question of existence of infinite paths of 1’s and their geometry. We will introduce percolation below but refer to other publications (such as the book by Grimmett [108]) for a systematic account of the theory. Percolation will come into play here on various levels. Its concepts like clusters, open paths, connectedness
Details
-
File Typepdf
-
Upload Time-
-
Content LanguagesEnglish
-
Upload UserAnonymous/Not logged-in
-
File Pages118 Page
-
File Size-