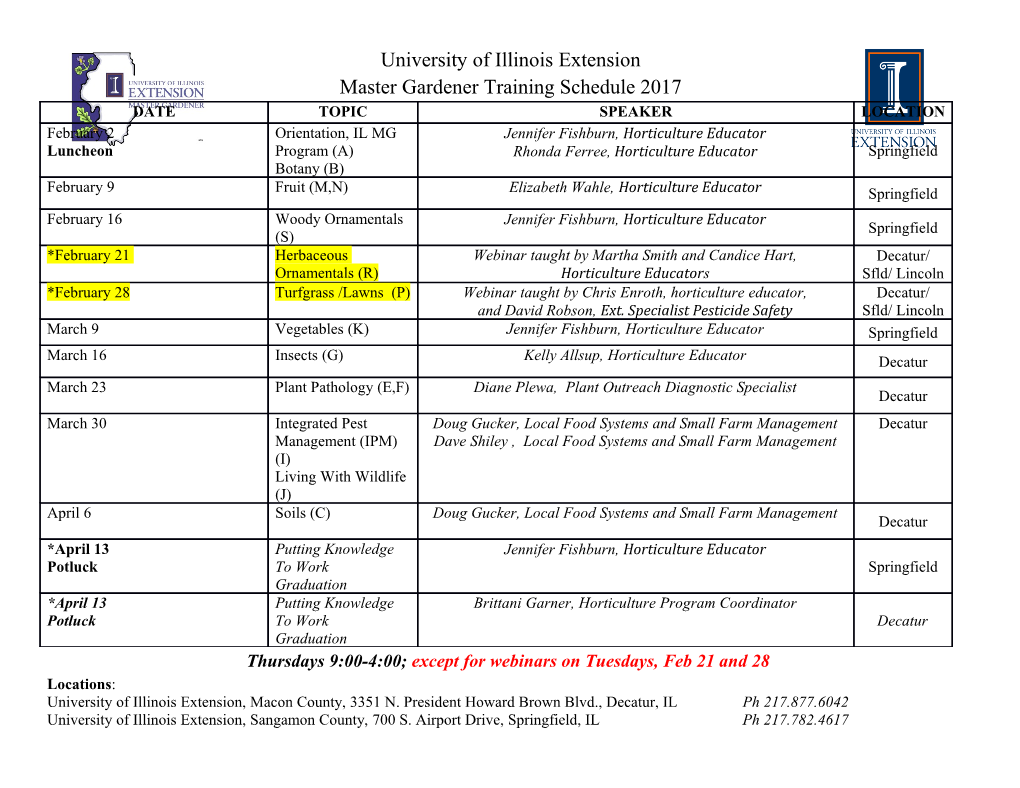
Network Performance Model for Urban Rail Systems by Baichuan Mo B.E., Tsinghua University (2018) Submitted to the Department of Urban Studies and Planning and Department of Electrical Engineering and Computer Science in partial fulfillment of the requirements for the degree of Master of Science in Transportation and Master of Science in Electrical Engineering and Computer Science at the MASSACHUSETTS INSTITUTE OF TECHNOLOGY May 2020 ○c Massachusetts Institute of Technology 2020. All rights reserved. Author............................................................................ Department of Urban Studies and Planning and Department of Electrical Engineering and Computer Science May 15, 2020 Certified by. Haris N. Koutsopoulos Professor of Civil and Environmental Engineering, Northeastern University Thesis Supervisor Certified by. Jinhua Zhao Associate Professor of City and Transportation Planning, MIT Thesis Supervisor Certified by. Patrick Jaillet Professor of Electrical Engineering and Computer Science, MIT Thesis Supervisor Accepted by....................................................................... P. Christopher Zegras Professor of Transportation and Urban Planning, MIT Chair, Program Committee Accepted by....................................................................... Leslie A. Kolodziejski Professor of Electrical Engineering and Computer Science, MIT Chair, Department Committee on Graduate Students 2 Network Performance Model for Urban Rail Systems by Baichuan Mo Submitted to the Department of Urban Studies and Planning and Department of Electrical Engineering and Computer Science on May 15, 2020, in partial fulfillment of the requirements for the degree of Master of Science in Transportation and Master of Science in Electrical Engineering and Computer Science Abstract Urban rail transit is an important component of transportation systems and plays a crit- ical role in providing smooth and efficient mobility in many metropolitan areas. Network performance monitoring, i.e. assessing the level of service and operation information of the network (e.g. train loads), is a fundamental task for urban rail transit management. The objective of this thesis is to develop a data-driven network performance model (NPM) for urban rail system performance monitoring. Specifically, this work focuses on two major components of the NPM: 1) a network loading engine which takes train movement data, origin-destination (OD) flows, network, train capacity, and path choices as inputs, andout- puts performance indicators such as train loads and crowding levels, and 2) a calibration engine which can estimate path choice and train capacity parameters using automatically collected data. The automated fare collection (AFC) and train movement data from Hong Kong’s Mass Rail Transit (MTR) network are used as a case study for the analysis. An event-based network loading engine is proposed. The model can distribute passengers in the network given OD demand, path choices, train capacity with a capacity constraint, and a first-come-first-board criterion. The event-based is computationally efficient while retaining the necessary performance information, which enables it to be applied with large- scale urban rail systems. An effective train capacity model is proposed, which assumes that train capacity is a function of train load and number of queuing passengers on platforms. The model recognizes that train capacity may vary across stations, which is seldom considered in the literature. The use of NPM for performance monitoring is demonstrated by analyz- ing the spatial-temporal crowding patterns in the MTR system and evaluating dispatching strategies. The model is validated by comparing its outputs (with effective capacity) with field observations at a busy station and the outputs of a benchmark fixed-capacity model. Results show that the output of the model matches the ground truth observations well and outperform the benchmark model. NPM is also used to identify crowding stations and evaluate different dispatching strategies. To calibrate path choices, an assignment-based path choice estimation framework using AFC data is proposed. The framework captures the crowding correlation among stations and the interaction between path choices and passenger denied boarding, which are usu- ally neglected in the literature. The path choice estimation is formulated as an optimization problem, which attempts to minimize the error between assignment outputs (which is a func- tion of path choices) and the corresponding quantities observed from the AFC data. The original problem is intractable because of a non-linear multinomial logit equation constraint 3 and a non-analytical black-box function constraint (i.e. assignment model). A solution pro- cedure is proposed to decompose the original problem into three tractable sub-problems: rough path shares estimation, choice parameters estimation, and path exit rates estimation. The sub-problems can all be solved efficiently. We prove the solution of the decomposed problem is equivalent to the original problem under certain conditions. The model is val- idated using both synthetic data and real-world AFC data. Results from synthetic data show estimated path choice parameters are very close to the “true” (synthetic) ones. The proposed method outperforms the benchmark models in both the convergence rate and final solutions quality. Results from real-world data show the estimated coefficients are similar to the previous survey results. The model’s robustness is verified through a sensitivity analysis. As the observed information in AFC data may also be affected by train capacity, a simul- taneous calibration of path choices and train capacity is more reasonable than calibrating one set of parameters alone. We propose a simulation-based optimization (SBO) framework to calibrate path choices and train capacity simultaneously using AFC and train movement data. The calibration problem is formulated as an optimization problem with a black-box objective function. Seven optimizers (solving algorithms) from four brunches of SBO solving methods are evaluated. The algorithms are evaluated using an experimental design that in- cludes five scenarios, representing different degrees of path choice randomness and crowding sensitivity. Results show that some of the algorithms can estimate the path choice and train capacity parameters well. In general, the response surface methods have better convergence speed, stability, and estimation accuracy. They exhibit consistently good performance under all scenarios. Future research directions include: 1) Developing a more efficient simultaneous path choices and train capacity calibration framework. The proposed SBO framework in this work is not computationally efficient due to a large number of expensive simulation-based function evaluations. Modifying the assignment-based framework to incorporate co-calibration is an interesting direction. 2) Developing a behavior-based effective train capacity model that incorporates passengers’ willingness to board explicitly. 3) Extending the NPM for real- time operation control and future planning. A reinforcement learning-based control engine and an automated timetable design engine can be added to the current NPM framework to enable control and planning applications, respectively. Thesis Supervisor: Haris N. Koutsopoulos Title: Professor of Civil and Environmental Engineering, Northeastern University Thesis Supervisor: Jinhua Zhao Title: Associate Professor of City and Transportation Planning, MIT Thesis Supervisor: Patrick Jaillet Title: Professor of Electrical Engineering and Computer Science, MIT 4 Acknowledgments Throughout my two years at MIT, I am indebted to many people for their companion, support, encouragement, and help. First, I would like to express my deepest gratitude to my advisors Prof. Jinhua Zhao and Prof. Haris N. Koutsopoulos. Jinhua and Haris are two different spirits that consistently inspire my life. Jinhua is a creator, showing me rich and colorful research ideas. He is like the compass in my research ship, guiding me towards a vast and attractive ocean. I pretty much enjoyed the extensive discussions and sparkling moments with him. Haris is a craftsman, digging into depth of techniques with thorough understanding. He is like the sail in my research ship, providing the essential energy support in a challenging journey. I pretty much appreciated his dedicated edition to every corner in my papers, rigorous attitudes towards every problem encountered, and enlightening suggestions in even the complicated methodology details. From them, I learned what a great mentor should be. I feel very honored to have both of them as my advisors during my studies at MIT. I would also like to thank Prof. Patrick Jaillet for being my thesis reader and offering advice to this dissertation. Thanks to John Attanucci for providing insightful comments for my various research jobs. I am thankful to my friends at JTL-Transit Urban Mobility Lab. I would like to especially thank Qing Yi Wang, with whom I discussed a lot of research ideas, had a lot of fun, and stayed up all night for thesis writing together. I would also like to thank Yunhan Zheng and Xiaotong Guo, who accompanied my daily study life, brought me much joy, and worked with me closely. Thanks to Zhenliang (Mike) Ma who worked with me collaboratively in the many research works. Mike is such a kind mentor that I can always ask questions for, helping me to own a wonderful start at MIT.
Details
-
File Typepdf
-
Upload Time-
-
Content LanguagesEnglish
-
Upload UserAnonymous/Not logged-in
-
File Pages104 Page
-
File Size-