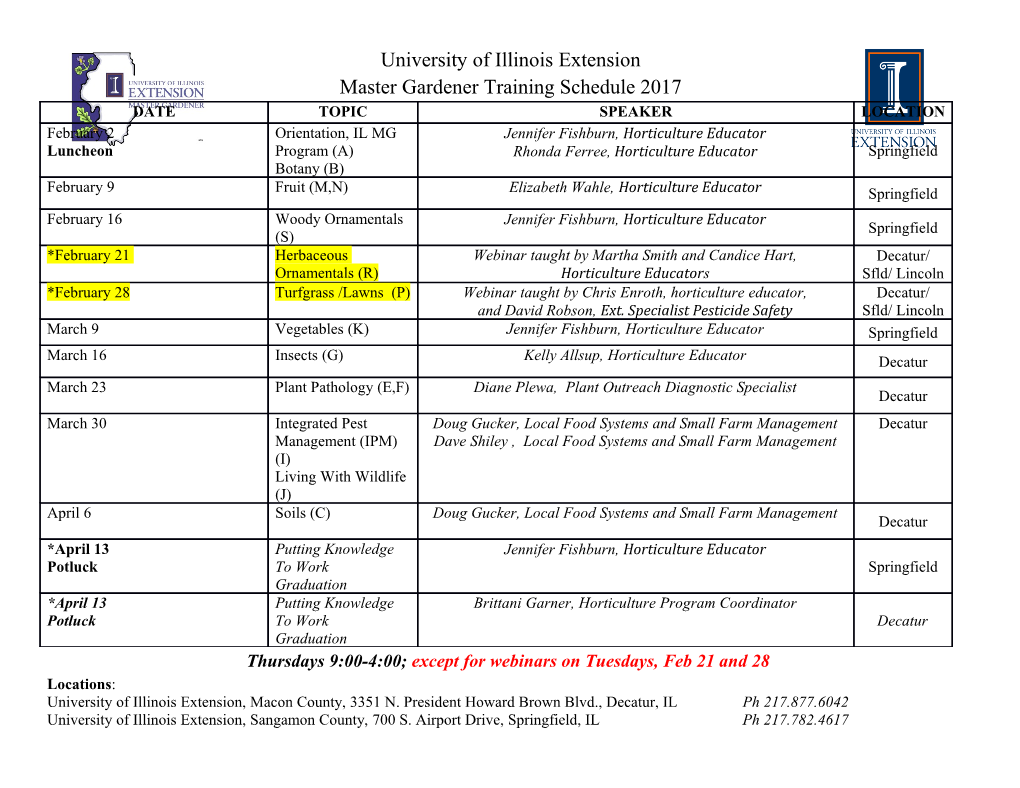
Communication for individual-level questions: Assessing predictive value of biomarkers. Coefficients of Determination based on Parametric Models for Survival Data Alvaro Muñoz. PhD Department of Epidemiology Johns Hopkins Bloomberg School of Public Health 1 Background 4Viral Load Æ decline of CD4 T cells in the untreated natural history Strong statistical significance (Mellors, Muñoz, …, Rinaldo. Ann Intern Med 1997) but poor R2 (explained variability) (Rodriguez, Sethi,…, Lederman. JAMA 2006) 2 Background 4Viral Load and CD4 Æ Disease progression: • AIDS-free time • Survival time (Mellors, et al, Ann Int Med 1997) 3 Proportion AIDS-free by plasma HIV-1 RNA (copies/ml) 1.0 <500 0.8 500 to 0.6 3,000 3,001 to 0.4 10,000 0.2 10,001 to 2 30,000 R = ? >30,000 0.0 0246810 Years since HIV-1 RNA quantification (bDNA) 4 Mellors, Muñoz,…,Phair. Ann Intern Med 1997 Background 4R2 for normally distributed outcomes Y= a + bX + {V(Y|X)}1/2 N(0,1) Var(Y)= b2 Var(X) + Var(Y|X) R2 = 1 - Var(Y|X) / Var(Y) 2 = b Var(X) / Var(Y) Equivalently, via maximum likelihood R2 = 1 – exp( - LikelihoodRatioStatistic / N) 5 Background 4R2 for survival data with m uncensored and N-m censored times LRS-based extensions: 2 RN =− 1exp( −LRS / N ) 2 and Rm =− 1exp( −LRS /) m Kent&O’Quigley’88; Schemper&Stare’96 2 2 Neither R N nor R m handles the incomplete data of censored times appropriately 6 Methods 4R2 for survival data with m uncensored and N-m censored times Data augmentation: Parametric approach provides basis to complete the partial information of censored times. Working model: Generalized Gamma (GG) 7 Parametric models for Time-to-Event data fGeneralized Gamma: GG(, ), ) f3-parameters: ÅÆlocation; median )ÅÆscale; Q3/Q1 ÅÆshape; GG(0, 1, ) f λ = 1 corresponds to Weibull λ = 0 corresponds to Lognormal λ = σ corresponds to standard Gamma Stacy 1962 Ann Math Stat; Prentice 1974 Bka f hazard function can be decreasing, increasing, bathtub or arc-shaped. Cox, Chu, Schneider, Muñoz. 2007 Stat Med8 3 2 σ = λ Shape (λ a m m a G 1 Weibull ) 0 σ Lognormal 1/ ) λ = = -1/ ag Amm -1 Inverse Ammag Inverse Weibull 9 Inverse Gamma = -) σ ) Scale ( Cox, Chu, Schneider, Muñoz. 2007 Stat Med 0123 Methods Cox, Chu, Schneider, Muñoz. 2007 Stat Med 4If the times are GG(α + βX, σ, λ): • Var[ logT | X ] = σ2 Var{ log[GG(0,1, λ)] } • R2 = 1 – Var[logT | X] / Var[logT] • Var[ logT ] = βT Cov(X) β + σ2 Var{ log[GG(0,1, λ)] } • Direct calculation of Var[logT] needs imputation of event of censored times10 Inadequate Method 4 Using the m:(N-m) uncensored:censored data, fit GG(,,) a11+ b X s 11 c 4 Instead of completing the partially missing information of the censored times, simply fit the null model GG (,,) a%%% 000 s c using the m:(N-m) uncensored:censored data. 4 2 2 sV11{log[ GG (0,1, c )]} R =−1 2 sV%%00{log[ GG (0,1, c )]} 11 What is inadequate about the Inadequate Method • Fitting GG (,,) a 11 + b X s 11 c induces one imputation for the incomplete data of censored times. • Fitting of GG (,,) a %%% 000 s c induces another imputation of the incomplete data of censored times which is inadequate if the X explains a significant portion of the variation of the times to event. 12 Methods Study Population N=1,640 HIV-positive (prevalent or seroconverters) Baseline visit: earliest semiannual visit after the first seropositive visit at which plasma HIV-1 RNA and CD4 cell count were available and visit took place before 1987.5 bDNA converted to RT-PCR values as RT-PCR= 5.13(bDNA)0.9 [Mellors Ann Int Med’97] HIV-1 RNA values below assay detection limits were handled in analyses using methods for left-censored data 13 Methods Simulation to characterize R2 measures N=1,640 AIDS-free times from GG (2.05 – 1.39 log10(HIV RNA / 30000); 0.97; 0.37) and Var[ log10(HIV RNA / 30000) ]= 0.53 that is, • For those with HIV RNA= 30000, median AIDS-free is exp(2.05) [ median of GG(0, 1, 0.37) ]0.97 = 7.0 yrs • for each one log increase in HIV RNA, the median is reduced by the factor exp(-1.39)= 0.25= ¼ • True R square: 2 2 › b1 Var(X)= (-1.39) x 0.53= 1.024 2 2 › s1 V{ log[GG(0,1, c1)] }= 0.97 x V{ log[GG(0,1, 0.37)] }= 1.008 14 › R2= 1.024 / [1.024+1.008] = 51% no censoring True R square= 51% time to AIDS (log) time to AIDS (log) 20246 20246 - - 23456 23456 15 HIV RNA (log10) HIV RNA (log10) Summary of Simulations True R2= 51% Censoring doa tail Imputation 50% 51% Inadequate 49% 77% 2 PH&LRS R N 33% 61% 2 PH&LRS R m 31% 52% 16 Results Observed Data N=1,640 followed from baseline to 1991.0 598:1042 D uncensored:censored D AIDS:AIDS-free Median of YOB baseline CD4* HIVRNA* CD4slope** time N=598 1951.32 1985.72 427 57,741 -103 2.81 N=1042 1952.78 1985.74 615 12,972 - 46 5.22 *CD4 and HIVRNA at baseline **cd4slope per year in the baseline to 1988.5 period Mellors, Margolick,…, Muñoz. 2007 JAMA 17 censored observed data ^^^^^^^ ^^ ^^^^^^ ^^^^^^^^^^^^^^^^^^^^^^^^^^^^^^^^^ ^ ^ ^^^^^^^^^^^^^^^^^^^^^^^^^^^^^^^^^^^^^^^^^^^^^^^^^^^^^^^^^^^^^^^^^^^^^^^^^^^^^^^^^^^^^^^^^^^^^^^^^^^^^^^^^^^^^^^^^^^^^^^^^^^^^^^^^^^^^^^^^^^^^^^^^^^^^ ^ ^ ^ ^^ ^^^^^^^^^^^^^^^^^^^^^^^^^^^^^^^^^^^^^^^^^^^^^^^^^^^^^^^^^^^^^^^^^^^^^^^^^^^^^^^^^ ^ ^ ^ ^^^^ ^ ^^^ ^^ ^^^^^^^^^^^^^^^^^^^^^ ^^^^^ ^ ^ ^ ^^ ^^ ^^^^^^ ^^^^^^ ^ ^^ ^ ^ ^ ^^^ time to AIDS (log) ^ ^ ^ ^ ^ ^ time to AIDS (log) ^ ^^ ^ ^ ^ ^ ^^^^ ^ ^ ^ ^^ ^ ^ ^ ^ ^ ^ ^ ^ ^ ^^ ^ ^ ^ ^ ^ ^ ^ ^ ^ ^ ^ ^ 20246 20246 - - inadequate R square= undef PH&LRS R squares= 30% and 62% 23456 23456 18 HIV RNA (log10) HIV RNA (log10) completed times for censored observed data (1042 of1640) after imp R square= 53% time to AIDS (log) time to AIDS (log) 20246 20246 - - inadequate R square= undef% PH&LRS R squares= 30% and 62% 23456 23456 19 HIV RNA (log10) HIV RNA (log10) Caveats 4Exceedingly long imputed times divulge the presence of competing risks. Possible approach: exclude them. 4Alternatively, treat right censored as interval censored with the assumption that in untreated HIV infection, all will develop AIDS and died before 30 years of infection or age 90. 4Additional work: Updated markers • Stacking: baseline is a moving target; apt for single measurement interest; simpler. • Classical: sc as origin; staggered entries; apt for longitudinal interest. 20 Summary 4Completing missing data for R-squares in survival analysis is a must. 4And if you think that you are not imputing, you are. 4When imputing, do it multiple times; or better yet, bootstrap it. 4HIV replication “explains” 50%, does activation explain part of the other 50 ? 4Make no denial, HIV is the cause of AIDS. 21.
Details
-
File Typepdf
-
Upload Time-
-
Content LanguagesEnglish
-
Upload UserAnonymous/Not logged-in
-
File Pages21 Page
-
File Size-