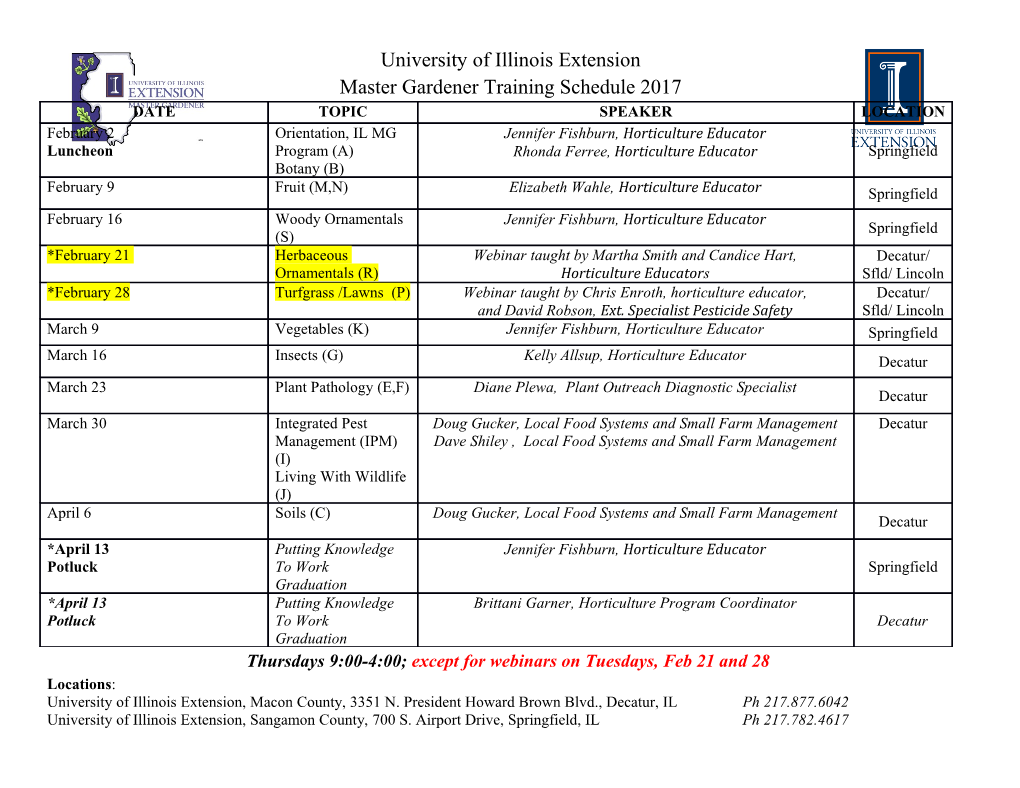
Going Beyond T-SNE: Exposing whatlies in Text Embeddings Vincent D. Warmerdam Thomas Kober Rachael Tatman Rasa Rasa Rasa Schonhauser¨ Allee 175 Schonhauser¨ Allee 175 Schonhauser¨ Allee 175 10119 Berlin 10119 Berlin 10119 Berlin [email protected] [email protected] [email protected] Abstract We introduce whatlies, an open source toolkit for visually inspecting word and sen- tence embeddings. The project offers a unified and extensible API with current support for a range of popular embedding backends includ- ing spaCy, tfhub, huggingface transformers, gensim, fastText and BytePair embeddings. The package combines a domain specific lan- guage for vector arithmetic with visualisation tools that make exploring word embeddings more intuitive and concise. It offers support for many popular dimensionality reduction techniques as well as many interactive visual- isations that can either be statically exported Figure 1: Projections of wking, wqueen, wman, wqueen − wking or shared via Jupyter notebooks. The project and wman projected away from wqueen − wking. Both the vector arithmetic and the visualisation were done using the https:// documentation is available from whatlies. The support for arithmetic expressions is integral rasahq.github.io/whatlies/. in whatlies because it leads to more meaningful visualisa- tions and concise code. 1 Introduction The use of pre-trained word embeddings (Mikolov of how representations for queen, king, man, and et al., 2013a; Pennington et al., 2014) or language woman can be projected along the axes vqueen−king model based sentence encoders (Peters et al., 2018; and vmanjqueen−king in order to derive a visualisation Devlin et al., 2019) has become a ubiquitous part of the space along the projections. of NLP pipelines and end-user applications in both Perhaps the most widely known tool for visu- industry and academia. At the same time, a grow- alising embeddings is the tensorflow projector1 ing body of work has established that pre-trained which offers 3D visualisations of any input em- embeddings codify the underlying biases of the beddings. The visualisations are useful for under- text corpora they were trained on (Bolukbasi et al., standing the emergence of clusters and the neigh- 2016; Garg et al., 2018; Brunet et al., 2019). Hence, bourhood of certain words and the overall space. practitioners need tools to help select which set of However, the projector is limited to dimensionality embeddings to use for a particular project, detect reduction as the sole preprocessing method. More potential need for debiasing and evaluate the debi- recently, Molino et al.(2019) have introduced par- ased embeddings. Simplified visualisations of the allax which allows explicit selection of the axes latent semantic space provide an accessible way to on which to project a representation. This creates achieve this. an additional level of flexibility as these axes can Therefore we created whatlies, a toolkit of- also be derived from arithmetic operations on the fering a programmatic interface that supports vec- embeddings. tor arithmetic on a set of embeddings and visual- The major difference between the tensorflow pro- ising the space after any operations have been car- ried out. For example, Figure1 shows an example 1https://projector.tensorflow.org/ 52 Proceedings of Second Workshop for NLP Open Source Software (NLP-OSS), pages 52–60 Virtual Conference, November 19, 2020. c 2020 Association for Computational Linguistics jector, parallax and whatlies is that the first two model= tf_hub+ 'nnlm-en-dim50/2' provide a non-extensible browser-based interface, lang_tf= TFHubLanguage(model) emb_tf= lang_tf['whatlies is awesome'] whereas whatlies provides a programmatic one. Therefore whatlies can be more easily extended # Huggingface to any specific practical need and cover individ- bert= 'bert-base-cased' lang_hf= HFTransformersLanguage(bert) ual use-cases. The goal of whatlies is to of- emb_hf= lang['whatlies rocks'] fer a set of tools that can be used from a Jupyter notebook with a range of visualisation capabili- Retrieved embeddings are python objects that ties that goes beyond the commonly used static contain a vector and an associated named. It comes T-SNE (van der Maaten and Hinton, 2008) plots. with extra utility methods attached that allow for whatlies can be installed via pip, the code easy arithmetic and visualisation. is available from https://github.com/RasaHQ/ The library is capable of retreiving embeddings whatlies2 and the documentation is hosted at for sentences too. In order to retrieve a sentence https://rasahq.github.io/whatlies/. representation for word-level embeddings such as fastText, whatlies returns the summed repre- 2 What lies in whatlies — Usage and sentation of the individual word vectors. For pre- Examples trained encoders such as BERT (Devlin et al., 2019) or ConveRT (Henderson et al., 2019), whatlies Embedding backends. The current version uses its internal [CLS] token for representing a of whatlies supports word-level as well as sentence. sentence-level embeddings in any human language that is supported by the following libraries: Similarity Retrieval. The library also supports retrieving similar items on the basis of a number of • BytePair embeddings (Sennrich et al., 2016) commonly used distance/similarity metrics such as via the BPemb project (Heinzerling and cosine or Euclidean distance: Strube, 2018) from whatlies.language import \ • fastText (Bojanowski et al., 2017) SpacyLanguage • gensim (Rehˇ u˚rekˇ and Sojka, 2010) lang= SpacyLanguage('en_core_web_md') lang.score_similar("man", n=5, • huggingface (Wolf et al., 2019) metric='cosine') [(Emb[man], 0.0), • sense2vec (Trask et al., 2015); via spaCy (Emb[woman], 0.2598254680633545), (Emb[guy], 0.29321062564849854), • spaCy3 (Emb[boy], 0.2954298257827759), (Emb[he], 0.3168887495994568)] • tfhub4 # NB: Results are cosine _distances_ Embeddings are loaded via a unified API: Vector Arithmetic. Support of arithmetic ex- pressions on embeddings is integral in any from whatlies.language import \ whatlies functions. For example the code for SpacyLanguage, FasttextLanguage, \ TFHubLanguage, HFTransformersLangauge creating Figure1 from the Introduction highlights that it does not make a difference whether the plot- # spaCy ting functionality is invoked on an embedding itself lang_sp= SpacyLanguage('en_core_web_md') emb_king= lang_sp["king"] or on a representation derived from an arithmetic emb_queen= lang_sp["queen"] operation: # fastText import matplotlib.pylab as plt ft= 'cc.en.300.bin' from whatlies import Embedding lang_ft= FasttextLanguage(ft) emb_ft= lang_ft['pizza'] man= Embedding("man",[0.5, 0.1]) woman= Embedding("woman",[0.5, 0.6]) # TF-Hub king= Embedding("king",[0.7, 0.33]) tf_hub= 'https://tfhub.dev/google/' queen= Embedding("queen",[0.7, 0.9]) man.plot(kind="arrow", color="blue") 2Community PRs are greatly appreciated . woman.plot(kind="arrow", color="red") 3 https://spacy.io/ , king.plot(kind="arrow", color="blue") 4https://www.tensorflow.org/hub queen.plot(kind="arrow", color="red") 53 diff= (queen- king) orth= (man| (queen- king)) es.score_similar(emb_es, n=5, metric='cosine') diff.plot(color="pink", [(Emb[rey], 0.04499000310897827), show_ops=True) (Emb[monarca], 0.24673408269882202), orth.plot(color="pink", (Emb[Rey], 0.2799408435821533), show_ops=True) (Emb[reina], 0.2993239760398865), # See Figure 1 for the result :) (Emb[prı´ncipe], 0.3025314211845398)] This feature allows users to construct custom nl.score_similar(emb_nl, n=5, metric='cosine') queries and use it e.g. in combination with the sim- ilarity retrieval functionality. For example, we can [(Emb[koning], 0.48337286710739136), validate the widely circulated analogy of Mikolov (Emb[koningen], 0.5858825445175171), (Emb[koningin], 0.6115483045578003), et al.(2013b) on spaCy’s medium English model (Emb[Koning], 0.6155656576156616), in only 4 lines of code (including imports): (Emb[kroonprins], 0.658723771572113)] While for Spanish, the correct answer reina is wqueen ≈ wking − wman + wwoman only at rank 3 (excluding rey from the list), the from whatlies.language import \ second ranked monarca (female form of monarch) SpacyLanguage is getting close. For Dutch, the correct answer lang= SpacyLanguage('en_core_web_md') koningin is at rank 2, surpassed only by koningen (plural of king). Another interesting observation >e= lang["king"]- lang["man"]+\ lang["woman"] is that the cosine distances — even of the query > lang.score_similar(e, n=5, words — vary wildly in the embeddings for the two metric='cosine') [(Emb[king], 0.19757413864135742), languages. (Emb[queen], 0.2119154930114746), (Emb[prince], 0.35989218950271606), Sets of Embeddings. In the previous examples (Emb[princes], 0.37914562225341797), we have typically only retrieved single embeddings. (Emb[kings], 0.37914562225341797)] However, whatlies also supports the notion of an “Embedding Set”, that can hold any number of Excluding the query word king5, the analogy embeddings: returns the anticipated result: queen. The library also allows the user to add/subtract from whatlies.language import \ embeddings but also project unto (via the > oper- SpacyLanguage ator) or away from them (via the j operator). This lang= SpacyLanguage("en_core_web_lg") means that the user is very flexible when it comes words=["prince", "princess", "nurse", to retrieving embeddings. "doctor", "man", "woman", "sentences also embed"] Multilingual Support. whatlies supports # NB: 'sentences also embed' will be any human language that is available from
Details
-
File Typepdf
-
Upload Time-
-
Content LanguagesEnglish
-
Upload UserAnonymous/Not logged-in
-
File Pages9 Page
-
File Size-