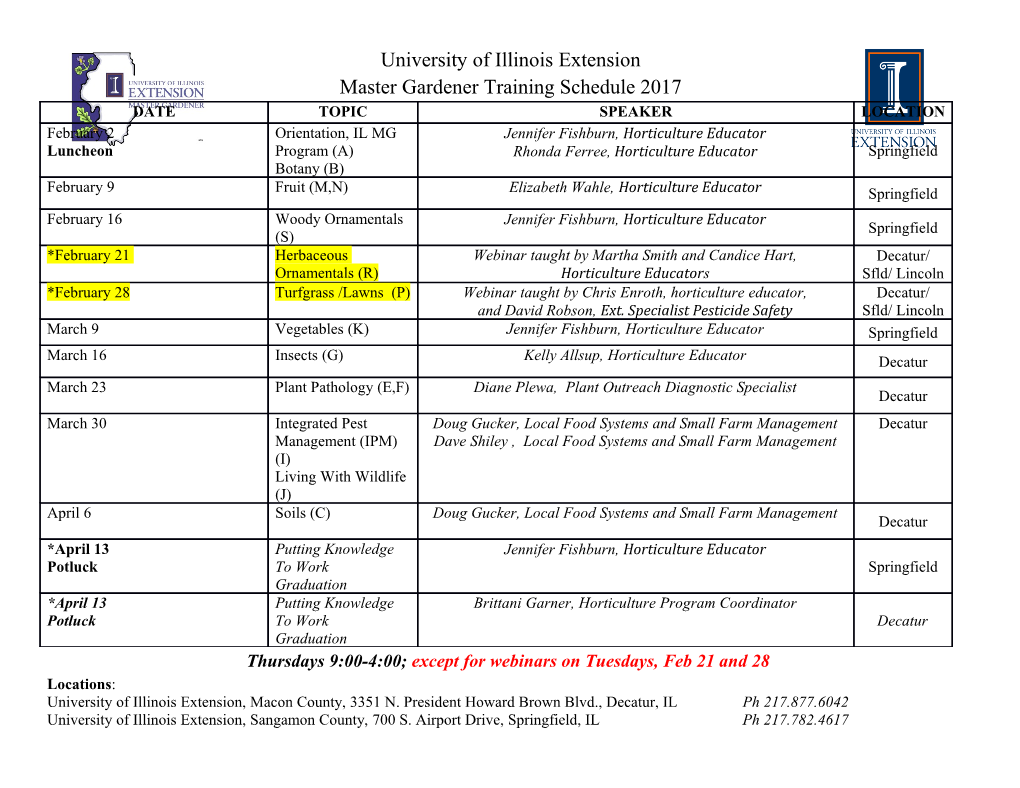
Journal of Sports Analytics 4 (2018) 153–163 153 DOI 10.3233/JSA-170199 IOS Press Who’s good this year? Comparing the information content of games in the four major US sports Julian Wolfson∗, Joseph S. Koopmeiners and Andrew DiLernia Division of Biostatistics, University of Minnesota, Minneapolis, MN, USA Abstract. In all four major North American professional sports (baseball, basketball, football, and hockey), the primary aim of the regular season is to identify the best teams. Yet the number of regular season games used to distinguish between the teams differs dramatically between the sports, ranging from 16 (football) to 82 (basketball and hockey) to 162 (baseball). While length of season is partially determined by factors including travel logistics, rest requirements, playoff structure and television contracts, from a statistical perspective the 10-fold difference in the number of games between leagues suggests that some league’s games are more “informative” than others. In this paper, we propose a method to quantify the amount of information games yield about the relative strengths of the teams involved. Our strategy is to estimate a predictive accuracy curve which assesses how well simple paired comparison models fitted from X% of games within a season predict the outcomes of the remaining (100 − X)% of games, across multiple values of X. We compare predictive accuracy curves between seasons within each sport and across all sports, and find dramatic differences in the amount of information yielded by game results in the four major North American sports leagues. Keywords: Prediction, information, paired comparison models, season length 1. Introduction Mullan, 2012) and that Tom Brady was the favorite for the MVP award (Reiss, 2012). Others opined that In week 14 of the 2012 National Football League the game provided evidence that the Texans were season, the New England Patriots squared off on in reality less talented than they appeared over the Monday Night Football against the Houston Texans first 13 weeks of the season (Kuharsky, 2012). These in a game with major implications for both teams. are strong conclusions to reach based on the results At the time, the Texans had the best record in the of a single game, but the power of such “statement conference (11 wins versus 1 loss) and were in games” is accepted wisdom in the NFL. In contrast, line to earn home-field advantage throughout the it is rare for the outcome of a single regular-season playoffs, while the New England Patriots (9 wins, 3 game to create or change the narrative about a team in losses) had the best record in their division and were the NBA, NHL, or MLB. While one might argue that hoping to solidify their playoff position and establish the shorter NFL season simply drives commentators themselves as conference favorites. The Patriots ulti- to imbue each game with greater metaphysical mean- mately defeated the Texans 42-14, which led some ing, an alternative explanation is that the outcome commentators to conclude that the Patriots were the of a single NFL contest actually does carry more favorites to win the Super Bowl (Walker, 2012, Mac- information about the relative strengths of the teams involved than a single game result in the other major ∗ North American professional sports. In this paper, Corresponding author: Julian Wolfson, Division of Biostatis- tics, University of Minnesota, Minneapolis, MN, USA. E-mail: we ask and attempt to answer the basic question: [email protected]. how much does the outcome of a single game tell 2215-020X/18/$35.00 © 2018 – IOS Press and the authors. All rights reserved This article is published online with Open Access and distributed under the terms of the Creative Commons Attribution Non-Commercial License (CC BY-NC 4.0). 154 J. Wolfson et al. / Who’s good this year? Comparing the information content of games in the four major US sports us about the relative strength of the two teams into training and test sets at random to reduce the involved? impact of longitudinal trends over the course of a In the four major North American professional season). A predictive accuracy curve is also obtained sports (baseball, basketball, football, and hockey), for describing how paired-comparison models fit- the primary purpose of the regular season is to ted from X number of games from a season predict determine which teams most deserve to advance to the outcomes of the remaining games in the season, the playoffs. Interestingly, while the ultimate goal of across multiple values of X. We begin by describing identifying the best teams is the same, the number the data and analysis methods we used in Section 2. of regular season games played differs dramatically Section 3 presents results from recent seasons of the between the sports, ranging from 16 (football) to 82 four major North American sports, and compares (basketball and hockey) to 162 (baseball). Though the “information content” of games across the four length of season is partially determined by many sports. In Section 4 we discuss the strengths and factors including travel logistics, rest requirements, limitations of our analysis. playoff structure and television contracts, it is hard to reconcile the 10-fold difference in the number of games in the NFL and MLB seasons unless 2. Methods games in the former are somehow more informative about team abilities than games in the latter. Indeed, 2.1. Data while it would be near-heresy to determine playoff eligibility based on 16 games of an MLB season We consider game results (home and away (even if each of the 16 games was against a different score) for the 2010-2015 seasons for the NFL, the opponent), this number of games is considered 2010-2011 to 2015-2016 seasons of the NBA, the adequate for the same purpose in the NFL. 2010-2011 to 2015-2016 seasons of the NHL, and There is a well-developed literature on the topic the 2010-2015 seasons of MLB. Game results for the of competitive balance and parity in sports leagues NFL, NBA and NHL were downloaded from Sports- (Owen, 2010; Horowitz, 1997; Mizak et al., 2005; Reference.com (Drinen, 2014) and game results for Lee, 2010; Hamlen, 2007; Cain and Haddock, 2006; MLB were downloaded from Retrosheet (Smith, Larsen et al., 2006; Ben-Naim et al., 2006; Kesenne,´ 2014). Only regular season games were considered 2000; Vrooman, 1995; Koopmeiners, 2012). How- in our analysis. A small number of tie games were ever, most papers focus on quantifying the degree removed from the data to simplify the fitting of mod- of team parity over consecutive years along with the els for binary win/loss outcomes, though we note that effects of measures taken to increase or decrease it. In there are models that can accommodate tied game papers which compare multiple sports, season length results (Rao and Kupper, 1967). is often viewed as a nuisance parameter to be adjusted for rather than a focus of inquiry. As a result, little 2.2. Quantifying Informativeness attention has been directed at the question of how information on relative team strength accrues over Let G represent the outcomes (either a win/loss the course of a single season. indicator or margin of victory) from all the games In this paper, we aim to quantify the amount within a single season of a sport. Our goal is to of information each game yields about the rela- quantify the amount of information on relative team tive strength of the teams involved. We estimate strength contained in a set of games G ⊂ G,asthe team strength via paired-comparison (Bradley and proportion of the total number of games in the set Terry, 1952) and margin-of-victory models which varies. We consider two types of paired compari- have been applied to ranking teams in a variety of son models in our work. Each game g ∈ G provides sports (McHale and Morton, 2011; Koehler and Rid- information on the home team (Hg = i), away team path, 1982; Sire and Redner, 2009; Martin, 1999). (Ag = j) and the game result as viewed from the The growth in information about the relative strength home team’s perspective. Let the binary win/loss of teams is quantified via a predictive accuracy curve game result Wg = 1 if the home team wins the game, which describes how paired-comparison models fit- otherwise Wg = 0, and let πi,j = P(Wg = 1), i.e., ted from X% of the games in a season predict the the probability that the home team, team i, defeats outcomes of the remaining (100 − X)% of games, the visiting team, team j in game g. We fit a logis- across multiple values of X (games are partitioned tic regression model which is equivalent (see Agresti J. Wolfson et al. / Who’s good this year? Comparing the information content of games in the four major US sports 155 (2002)) to the standard Bradley-Terry model (Bradley where πˆ g andμ ˆ g are estimates derived from game and Terry, 1952): results in G, and |G| denotes the number of games in G. The BT and MOV superscripts denote the = − + logit πi,j βi βj α, (1) Bradley-Terry (i.e., win-loss) and margin of victory models respectively. The βi and βj terms are the team strength parameters BT MOV for teams i and j, respectively, and α is a home-field For a given season, we estimated I and I advantage parameter. for sets of game results corresponding to 12.5%, We fit a similar model when the actual game scores 25.0%, 37.5%, 50.0%, 62.5%, 75.0% and 87.5% of are considered.
Details
-
File Typepdf
-
Upload Time-
-
Content LanguagesEnglish
-
Upload UserAnonymous/Not logged-in
-
File Pages11 Page
-
File Size-