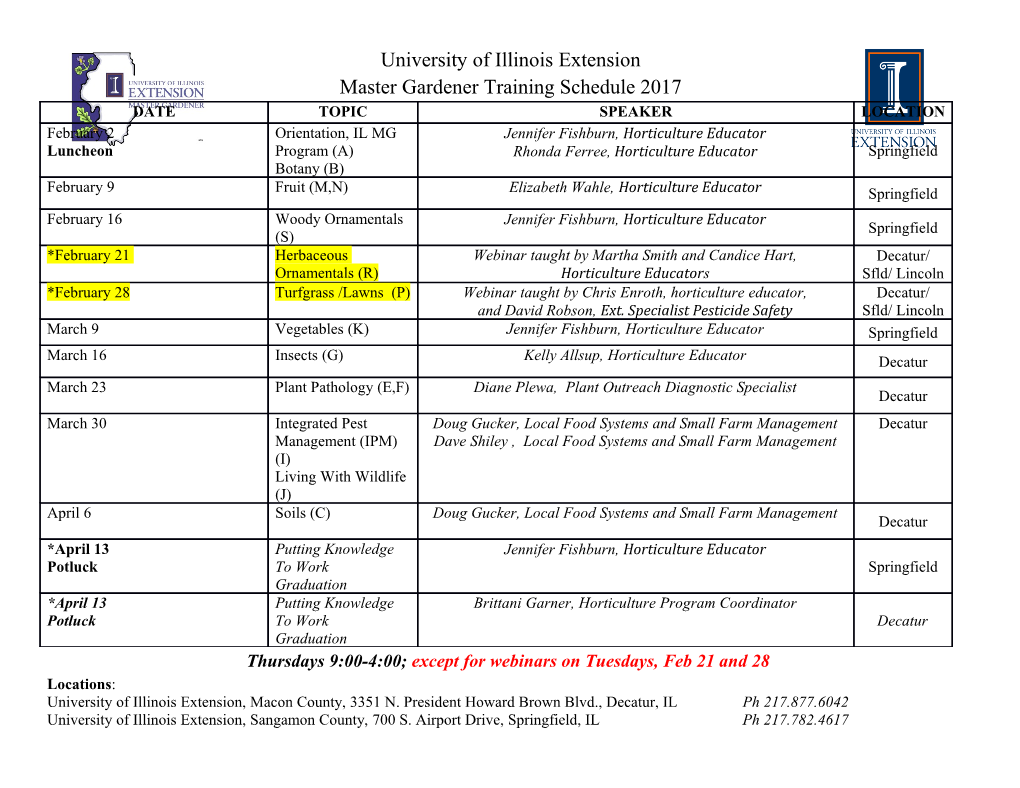
Toni Heittola Automatic Classi¯cation of Music Signals Master of Science Thesis The subject was approved by the Department of Information Technology on the 14th February 2003. Thesis supervisors: Professor Jaakko Astola (TUT) M.Sc. Anssi Klapuri (TUT) M.Sc. Antti Eronen (Nokia) Preface This work was carried out at the Institute of Signal Processing, Department of Information Technology, Tampere University of Technology, Finland. I wish to express my gratitude to my thesis advisors and examiners Mr. Anssi Klapuri and Mr. Antti Eronen, for they constant guidance, advice, and patience throughout this work. Further, I thank the examiner Professor Jaakko Astola for his advice and comments. I am grateful to Ms. Paula Keltto for her great e®ort to collect and thoroughly annotate all the pieces in the music database. I want also to thank Mr. Vesa Peltonen for his advice and for the database access tools, and Mr. Tuomas Virtanen for his sinusoids-plus-noise spectrum model used in this thesis. I am grateful to Mr. Juha Tuomi for all the valuable discussions during the development of the system. I want to thank the members of the Audio Research Group of Tampere University of Technology for their valuable knowledge and support and for providing a stimulating working atmosphere. I wish to thank my mother and grandparents for all understanding, support and endless love. Big thanks also to my friends for understanding my little time for them during this work. Tampere, December 2003 Toni Heittola i Contents Preface . i Contents . ii TiivistelmÄa . v Abstract . vi List of acronyms and symbols . vii 1 Introduction . 1 1.1 Musical genre recognition . 2 1.2 Instrument detection . 2 1.3 Organisation of the thesis . 3 2 Literature review . 4 2.1 Musical genre . 4 2.1.1 Genre taxonomy . 4 2.2 Human performance for genre classi¯cation . 5 2.3 Automatic musical genre recognition . 6 2.3.1 State-of-the-art . 6 2.3.2 Features . 9 2.3.3 Classi¯cation . 13 2.4 Related work . 15 2.4.1 Musical signal processing . 15 2.4.2 Speech/music discrimination and general audio classi¯cation . 16 2.4.3 Speaker recognition and veri¯cation . 16 3 Music database . 18 3.1 Collecting the database . 18 3.2 Format of acoustic data . 18 3.3 Annotations . 19 3.3.1 File format . 19 3.3.2 Annotation ¯elds . 19 3.4 Musical genre hierarchy . 22 3.4.1 Descriptions of musical genres . 24 3.5 Statistics of the database . 25 ii Contents 4 Listening experiment . 28 4.1 Experiment design . 28 4.1.1 Objectives and assumptions . 28 4.1.2 Method . 29 4.1.3 Stimuli . 29 4.1.4 Equipment and facilities . 30 4.1.5 Subjects . 31 4.2 Test procedure . 31 4.2.1 Preparation . 31 4.2.2 Familiarisation stage . 32 4.2.3 Main experiment . 33 4.2.4 User interface for data collection . 34 4.3 Results . 34 4.3.1 Analysis . 37 4.4 Discussion and conclusions . 38 5 System description . 40 5.1 General structure of pattern recognition system . 40 5.2 Extraction of spectral features . 40 5.2.1 Mel-frequency cepstral coe±cients . 41 5.2.2 Band Energy Ratio . 43 5.3 Rhythm feature extraction . 43 5.3.1 Preprocessing with sinusoidal modelling . 44 5.3.2 Periodicity detection . 44 5.4 Classi¯cation . 48 5.4.1 K-Nearest Neighbour classi¯er . 48 5.4.2 Hidden Markov Models . 48 6 System evaluation . 58 6.1 Musical genre recognition . 58 6.1.1 Method of evaluation . 58 6.1.2 Results . 59 6.1.3 Comparison with human abilities . 69 6.1.4 Discussion . 72 6.2 Instrument detection . 72 6.2.1 Detection . 73 6.2.2 Method of evaluation . 74 6.2.3 Results . 74 6.2.4 Discussion . 75 6.3 Locating segments with drums . 76 6.3.1 Test setup . 76 6.3.2 Results . 77 6.3.3 Discussion . 81 7 Conclusions . 83 Bibliography . 84 iii Contents A Musical genre hierarchy . 90 B Pieces in the music database . 95 iv TiivistelmÄa TAMPEREEN TEKNILLINEN YLIOPISTO Tietotekniikan osasto SignaalinkÄasittelyn laitos HEITTOLA, TONI: Musiikkisignaalien automaattinen luokittelu DiplomityÄo, 100 s. Tarkastajat: Prof. Jaakko Astola, DI Anssi Klapuri, DI Antti Eronen Rahoittajat: Tampereen teknillinen yliopisto, SignaalinkÄasittelyn laitos, Nokia Re- search Center. Joulukuu 2003 Avainsanat: musiikin sisÄaltÄoanalyysi, musiikin luokittelu, automaattinen musiikki- tyylin tunnistus, kuuntelukoe, soitinten havaitseminen. Digitaaliset musiikkikokoelmat ovat yleistyneet viime vuosina. Samalla kun nÄamÄa kokoelmat laajenevat tulee digitaalisen sisÄallÄon hallinta yhÄa tÄarkeÄammÄaksi. TÄas- sÄa diplomityÄossÄa tutkitaan sisÄaltÄopohjaista akustisten musiikkisignaalien luokitte- lua musiikkityylin (esim. klassinen, rock) sekÄa kÄaytettyjen soitinten pohjalta. Ihmi- sen kykyÄa tunnistaa musiikkityylejÄa tutkittiin kuuntelukokeella. TÄamÄa diplomityÄo kattaa kirjallisuustutkimuksen ihmisen musiikkityylin tunnistuksesta, nykyaikaisista musiikkityylin tunnistusjÄarjestelmistÄa sekÄa muista aiheeseen liittyvistÄa aloista. Li- sÄaksi esitellÄaÄan monikÄayttÄoinen musiikkitietokanta joka koostuu ÄaÄanityksistÄa sekÄa niiden manuaalisesti tehdyistÄa annotaatioista. MyÄos tyÄossÄa kÄaytettyjen piirteiden sekÄa luokittimien teoria selostetaan ja tehtyjen simulaatioiden tulokset esitellÄaÄan. Kehitetty musiikkityylin tunnistusjÄarjestelmÄa kÄayttÄaÄa mel-taajuus kepstrikertoimia esittÄamÄaÄan musiikkisignaalin aikamuuttuvaa magnitudispektriÄa. Musiikkityylikoh- taiset piirrejakaumat mallinnetaan piilotetuilla Markov malleilla. Soittimen havait- semista musiikista tutkitaan vastaavalla jÄarjestelmÄallÄa. LisÄaksi tÄassÄa tyÄossÄa esitetÄaÄan menetelmÄa rumpusoitinten havaitsemiseen. Rumpusoitinten lÄasnÄaolo musiikissa tun- nistetaan havainnoimalla jaksollisuutta signaalin alikaistojen amplitudiverhokÄayris- sÄa. Suoritettu kuuntelukoe osoittaa, ettÄa musiikkityylin tunnistus ei ole yksiselitteis- tÄa edes ihmiselle. Ihmiset pystyvÄat tunnistamaan oikean musiikkityylin keskimÄaÄarin 75 % tarkuudella (viiden sekunnin nÄaytteillÄa). LisÄaksi tulokset osoittavat, ettÄa ih- miset pystyvÄat tunnistamaan musiikkityylin melko tarkasti ilman pitkÄan aikavÄalin temporaalisia piirteitÄa, kuten rytmi. Kuudelle musiikkityylille kehitetty automaat- tinen musiikkityylin tunnistusjÄarjestelmÄa saavutti noin 60 % tarkkuuden, joka on vertailukelpoinen muiden vastaavien tunnistusjÄarjestelmien kanssa. Rumpusoitinten havainnoimisessa saavutettiin 81 % tarkkuus kÄayttÄaen esitettyÄa menetelmÄaÄa. v Abstract TAMPERE UNIVERSITY OF TECHNOLOGY Department of Information Technology Institute of Signal Processing HEITTOLA, TONI: Automatic Classi¯cation of Music Signals Master of Science Thesis, 100 pages. Examiners: Prof. Jaakko Astola, M.Sc. Anssi Klapuri, M.Sc. Antti Eronen Funding: Tampere University of Technology, Institute of Signal Processing, Nokia Research Center. December 2003 Keywords: music content analysis, music classi¯cation, automatic musical genre recognition, listening experiment, musical instrument detection. Collections of digital music have become increasingly common over the recent years. As the amount of data increases, digital content management is becoming more important. In this thesis, we are studying content-based classi¯cation of acoustic musical signals according to their musical genre (e.g., classical, rock) and the instru- ments used. A listening experiment is conducted to study human abilities to recog- nise musical genres. This thesis covers a literature review on human musical genre recognition, state-of-the-art musical genre recognition systems, and related ¯elds of research. In addition, a general-purpose music database consisting of recordings and their manual annotations is introduced. The theory behind the used features and classi¯ers is reviewed and the results from the simulations are presented. The developed musical genre recognition system uses mel-frequency cepstral coef- ¯cients to represent the time-varying magnitude spectrum of a music signal. The class-conditional feature densities are modelled with hidden Markov models. Mu- sical instrument detection for a few pitched instruments from music signals is also studied using the same structure. Furthermore, this thesis proposes a method for the detection of drum instruments. The presence of drums is determined based on the periodicity of the amplitude envelopes of the signal at subbands. The conducted listening experiment shows that the recognition of musical genres is not a trivial task even for humans. On the average, humans are able to recognise the correct genre in 75 % of cases (given ¯ve-second samples). Results also indicate that humans can do rather accurate musical genre recognition without long-term temporal features, such as rhythm. For the developed automatic recognition system, the obtained recognition accuracy for six musical genres was around 60 %, which is comparable to the state-of-the-art systems. Detection accuracy of 81 % was obtained with the proposed drum instrument detection method. vi List of acronyms and symbols ACF Autocorrelation Function BER Band Energy Ratio DCT Discrete Cosine Transform DFT Discrete Fourier Transform EER Equal Error Rate ESACF Enhanced Summary Autocorrelation Function FA False Acceptance FR False Rejection GMM Gaussian Mixture Model HMM Hidden Mixture Model k-NN k-Nearest Neighbour MFCC Mel-Frequency Cepstral Coe±cients MIR Music Information Retrieval ML Maximum Likelihood MMI Maximum Mutual Information PCA Principal Component Analysis ROC Receiver Operation Characteristic SACF Summary Autocorrelation Function x vector x A0 transpose of matrix A A¡1 inverse of matrix A ¸ parameter set of an HMM th th ¹i;m mean vector for the m mixture component of the i state
Details
-
File Typepdf
-
Upload Time-
-
Content LanguagesEnglish
-
Upload UserAnonymous/Not logged-in
-
File Pages108 Page
-
File Size-