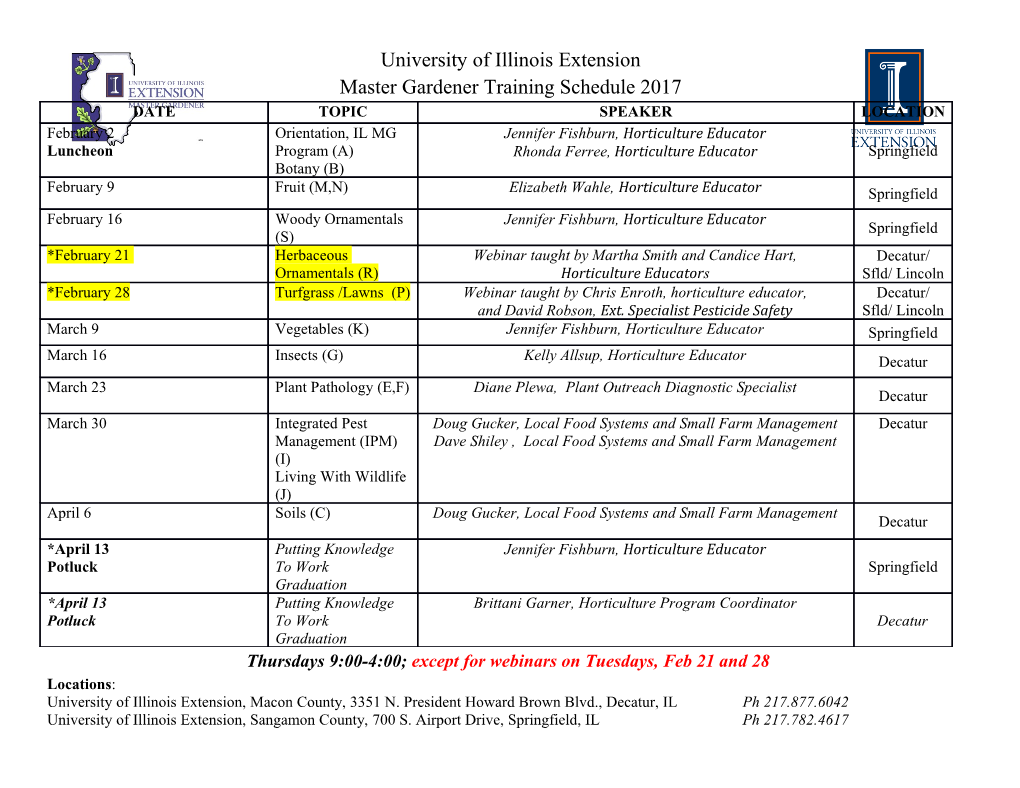
fo'oHkkjr @tdil 3. Development of OHWR System for Gurmukhi R.K. Sharma, Palki Sharma, Narpinder Sharma, Harjeet Singh, Karun Verma, Ravinder Kumar, Rajesh Kumar, School of Mathematics & Computer Applications, Thapar University, Patiala, Punjab (INDIA) Abstract Gurmukhi and Roman numerals. Handwritten character recognition is a Postprocessing of classes has also been complex task owing to various writing styles performed in this work in order to refine the of different individuals. A number of authors recognition process results. In this have worked on the problem of handwritten postprocessing, the sequence of classes is Gurmukhi OHWR character recognition. They have, in analyzed; overwritten strokes are identified general, used structural and statistical and resolved. A heuristic based algorithm features in their work. Handwritten has been developed to form Gurmukhi character recognition systems have also aksharas from the identified classes. been proposed for Gurmukhi script by some 1. Introduction authors. This work presents a system to This is a well-established fact that recognize online handwritten Gurmukhi handwritten character recognition is a characters, Gurmukhi numerals, Roman complex task. This is due to different numerals and special characters. A handwriting styles of individuals, and also the sufficiently large annotated database has cursiveness in their handwriting. Handwritten been created in this work for the strokes used character recognition is divided into two in writing these Gurmukhi symbols. The categories: offline and online. In offline recognition engines developed in this work handwritten character recognition, data are are based on 100 samples (Engine 1) and 22 scanned images taken from a prewritten text, samples (Engines 2, 3 and 4) of each class usually on a sheet of paper. In online collected from different writers. These handwriting recognition systems, data are samples have been preprocessed and captured while writing with the help of a annotated. LibSVM classifier has been used special pen and an electronic surface. These in this work for classification purpose. The systems generally include the phases of system presented in this work could attain the preprocessing, features extraction and highest recognition accuracy of 96.8% at 5- classification. Feature extraction is a very fold cross validation for Gurmukhi significant phase in a character recognition characters, of 90.0% at 4-fold cross system, which is used to decide for the validation for Gurmukhi numerals, of 90.2% relevant shape contained in the character. The at 5-fold cross validation for Gurmukhi performance of a character recognition numerals and Roman numerals, of 84.0% at system largely depends on the features, which 4-fold cross validation for special characters, have been extracted. 55.
Details
-
File Typepdf
-
Upload Time-
-
Content LanguagesEnglish
-
Upload UserAnonymous/Not logged-in
-
File Pages1 Page
-
File Size-