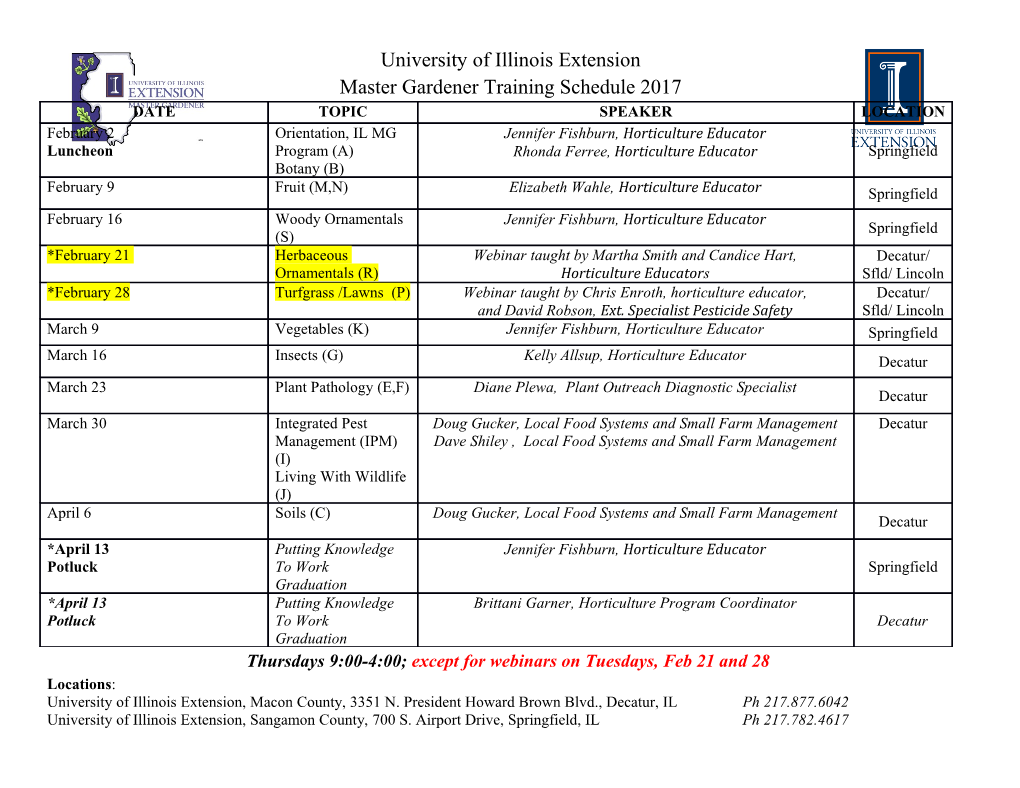
Networks beyond pairwise interactions: structure and dynamics Federico Battiston, Giulia Cencetti, Iacopo Iacopini, Vito Latora, Maxime Lucas, Alice Patania, Jean-Gabriel Young, Giovanni Petri To cite this version: Federico Battiston, Giulia Cencetti, Iacopo Iacopini, Vito Latora, Maxime Lucas, et al.. Networks beyond pairwise interactions: structure and dynamics. Physics Reports, Elsevier, 2020, 874, pp.1-92. 10.1016/j.physrep.2020.05.004. hal-03094211 HAL Id: hal-03094211 https://hal.archives-ouvertes.fr/hal-03094211 Submitted on 4 Jan 2021 HAL is a multi-disciplinary open access L’archive ouverte pluridisciplinaire HAL, est archive for the deposit and dissemination of sci- destinée au dépôt et à la diffusion de documents entific research documents, whether they are pub- scientifiques de niveau recherche, publiés ou non, lished or not. The documents may come from émanant des établissements d’enseignement et de teaching and research institutions in France or recherche français ou étrangers, des laboratoires abroad, or from public or private research centers. publics ou privés. Networks beyond pairwise interactions: structure and dynamics Federico Battiston∗ Department of Network and Data Science, Central European University, Budapest 1051, Hungary Giulia Cencetti Mobs Lab, Fondazione Bruno Kessler, Via Sommarive 18, 38123, Povo, TN, Italy Iacopo Iacopini School of Mathematical Sciences, Queen Mary University of London, London E1 4NS, United Kingdom and Centre for Advanced Spatial Analysis, University College London, London, W1T 4TJ, United Kingdom Vito Latora† School of Mathematical Sciences, Queen Mary University of London, London E1 4NS, United Kingdom Dipartimento di Fisica ed Astronomia, Universit`adi Catania and INFN, I-95123 Catania, Italy The Alan Turing Institute, The British Library, London NW1 2DB, United Kingdom and Complexity Science Hub Vienna (CSHV), Vienna, Austria Maxime Lucas Aix Marseille Univ, CNRS, CPT, Turing Center for Living Systems, Marseille, France Aix Marseille Univ, CNRS, IBDM, Turing Center for Living Systems, Marseille, France and Aix Marseille Univ, CNRS, Centrale Marseille, I2M, Turing Center for Living Systems, Marseille, France Alice Patania Network Science Institute, Indiana University, Bloomington, IN, USA Jean-Gabriel Young Center for the Study of Complex Systems, University of Michigan, Ann Arbor, MI, USA, 48109 Giovanni Petri‡ ISI Foundation, via Chisola 5, 10126 Turin, Italy and ISI Global Science Foundation, 33 W 42nd St, 10036 New York NY, USA (Dated: June 3, 2020) The complexity of many biological, social and technological systems stems from the richness of the interactions among their units. Over the past decades, a great variety of complex systems has been successfully described as networks whose interacting pairs of nodes are connected by links. Yet, in face-to-face human communication, chemical reactions and ecological systems, interactions can occur in groups of three or more nodes and cannot be simply described just in terms of simple dyads. Until recently, little attention has been devoted to the higher-order architecture of real complex systems. However, a mounting body of evidence is showing that taking the higher-order structure of these systems into account can greatly enhance our modeling capacities and help us to understand and predict their emerging dynamical behaviors. Here, we present a complete overview of the emerging field of networks beyond pairwise interactions. We first discuss the methods to represent higher-order interactions and give a unified presentation of the different frameworks used to describe higher-order systems, highlighting the links between the existing concepts and representations. We review both the measures designed to characterize the structure of these systems, and the models proposed in the literature to generate synthetic structures, such as random and growing simplicial complexes, bipartite graphs and hypergraphs. We then introduce and discuss the rapidly growing research on arXiv:2006.01764v1 [physics.soc-ph] 2 Jun 2020 higher-order dynamical systems and on dynamical topology. We focus on novel emergent phenomena characterizing landmark dynamical processes, such as diffusion, spreading, synchronization and games, when extended beyond pairwise interactions. We elucidate the relations between higher- order topology and dynamical properties, and conclude with a summary of empirical applications, providing an outlook on current modeling and conceptual frontiers. ∗ [email protected] † [email protected] ‡ [email protected] 2 CONTENTS I. Introduction 3 II. Higher-order representations of networks5 A. Elementary representations of higher-order interactions5 1. Low- versus high-order representations5 2. Graph-based representations5 3. Explicit higher-order representations7 B. Relations and links between representations8 III. Measures 9 A. Matrix representations of higher-order systems 10 1. Incidence matrix 10 2. Adjacency matrix 10 B. Walks, paths and centrality measures 11 1. Degree centralities 11 2. Paths and path-based centralities 13 3. Eigenvector centralities 14 C. Triadic closure and clustering coefficient 15 D. Simplicial homology 16 1. Boundary operators and homology groups 16 2. Evolving simplicial complexes 16 3. Other measures of shape in simplicial complexes 17 E. Higher-order Laplacian operators 17 1. Hypergraph Laplacians 18 2. Combinatorial Laplacians 18 IV. Models 20 A. Equilibrium models 21 1. Bipartite models 21 2. Motifs models 24 3. Stochastic set models 25 4. Hypergraphs models 27 5. Simplicial complexes models 29 B. Out-of-equilibrium models 31 1. Bipartite models 32 2. Stochastic set models 33 3. Hypergraphs models 33 4. Simplicial complexes models 34 V. Diffusion 36 A. Higher-order diffusion 37 1. Edge-flows 38 B. Higher-order random walks 39 1. Random walks on simplicial complexes 39 2. Random walks on hypergraphs 40 VI. Synchronization 43 A. Phase oscillators 43 1. Higher-order Kuramoto model 43 2. Higher-order interactions from phase reduction 50 B. Nonlinear oscillators 52 1. Chaotic oscillators 52 2. Neuron models 53 C. Inference of nonpairwise interactions in coupled oscillators 55 VII. Spreading and social dynamics 57 A. Spreading in higher-order networks 57 3 1. Spreading on simplicial complexes 58 2. Spreading on hypergraphs 59 B. Opinion and cultural dynamics beyond pairwise interactions 61 1. Voter model 61 2. Majority models 63 3. Continuous models of opinion dynamics 64 4. Cultural dynamics 65 VIII. Evolutionary games 65 A. Multiplayer games on networks 67 1. Public goods game 67 2. Other multiplayer games 69 B. Games with higher-order interactions 69 1. Public goods game on bipartite networks 69 2. Public goods game on hypergraphs 70 IX. Applications 71 A. Social systems 72 B. Neuroscience and brain networks 75 C. Ecology 78 D. Other biological systems 83 X. Outlook and conclusions 85 Acknowledgments 87 References 88 I. INTRODUCTION Any significant understanding of a complex system must rely on system level descriptions. Consider the following exercise: take an ecosystem, and break it into its pieces. No matter how good or accurate our knowledge at the level of the individual species is, chances are that our understanding of population dynamics (e.g. how the abundances of the different species change in time) will be slim at best. The same holds true when we attempt to explain epileptic seizures starting from the individual neurons of the human brain; or viral rumors spreading across societies from individual human psychology. All these approaches fail because they are missing a fundamental ingredient of any complex system, that is the rich pattern of nonlinear interactions between the system components. After many years of reductionism, science has abandoned the idea that the collective behaviors of a complex system can be simply understood and predicted by considering the units of the system in isolation [1], and now more than ever is embracing the idea of complexity as one of the principles governing the world we live in. Within this paradigm, networks have emerged as a reference modeling tool for complex systems [2]. Networks are the maps that define the physical or virtual space where interactions take place. Add competitive and cooperative relationships to an ecosystem, synaptic connections to the human brain, and human interactions to rumor spreading, and readily the self-organizing patterns and the collective behavior we observe in nature begin to unravel and look less obscure. Building on earlier work in mathematics, social network analysis and ecology, a handful of breakthrough papers at the turn of the millennium has attracted the interest of the scientific community, triggering thousands of contributions over the last twenty years and leading to the formation of the new multidisciplinary field of Network Science. This new research community has developed an unusual mixture of graph theory and statistical mechanics into a flourishing discipline, with applications spanning the full range of science, from fundamental physics all the way to the social sciences. The boundaries and potential applications are yet to be fully realized [2]. Still, the richness in scope and tools has already made the field of networks an independent discipline, often referred to as a science of its own. The growth of network science was also strengthened by the progressively wider availability of large datasets with detailed information on social, technological and biological interactions, which provided the raw material
Details
-
File Typepdf
-
Upload Time-
-
Content LanguagesEnglish
-
Upload UserAnonymous/Not logged-in
-
File Pages110 Page
-
File Size-