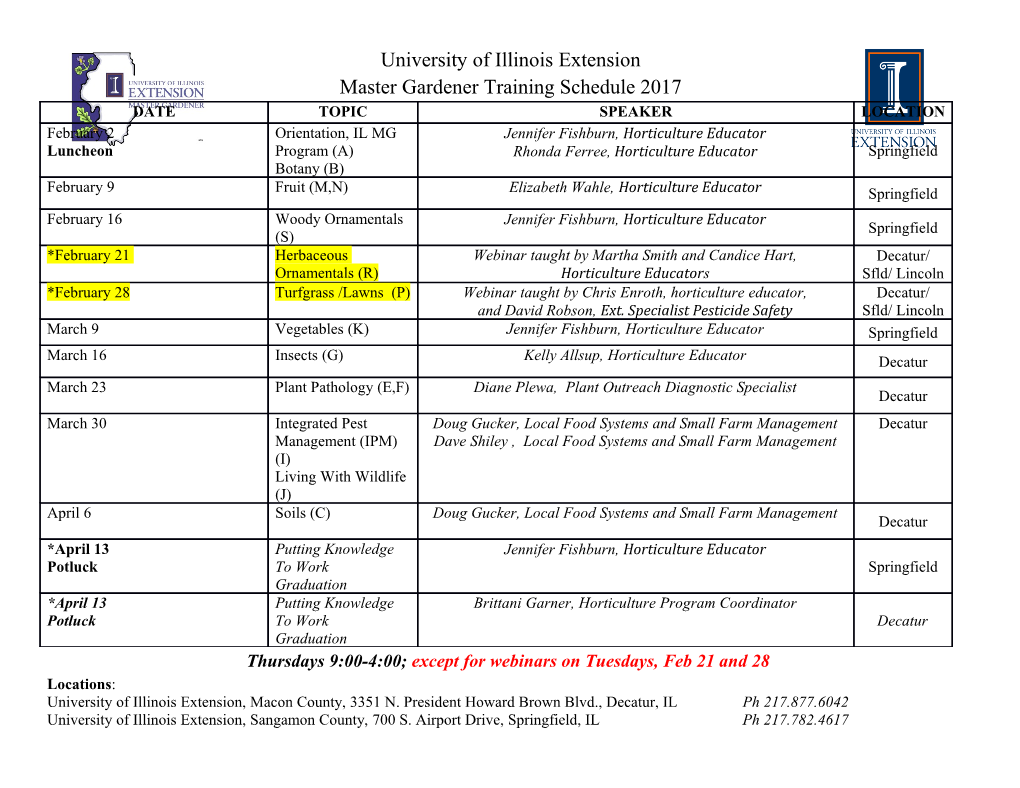
Connectivity analysis of single-cell RNA- seq derived transcriptional signatures A dissertation submitted to the Graduate School of the University of Cincinnati in partial fulfillment of the requirement for the degree of Doctor of Philosophy by Naim Al Mahi M.Sc. In Statistics, Ball State University, IN, 2014 B.Sc. In Statistics, University of Dhaka, Bangladesh, 2011 Committee Members: Mario Medvedovic, PhD (Chair) Jaroslaw Meller, PhD Siva Sivaganesan, PhD Jane Yu, PhD November 7, 2020 Division of Biostatistics and Bioinformatics Department of Environmental and Public Health Sciences University of Cincinnati College of Medicine Cincinnati, OH Abstract With the recent progress in high-throughput sequencing technologies, unprecedented amount of data from multiple omics modalities including genomics, transcriptomics, proteomics, and small-molecule data have become available. Accessibility and reusability of these biomedical big data provide immense opportunities and challenges as well. For example, integrating and connecting multiple data modalities such as, connecting single-cell transcriptomics data to small-molecule perturbational data can enhance the understanding of complex mechanisms underlie a disease and identify potential therapeutics. Recently released LINCS (Library of Integrated Network-based Cellular Signatures)-L1000 transcriptional signatures of chemical perturbations has opened new avenues to study cellular responses to existing drugs and new bioactive compounds. Connecting transcriptional signature of a disease to these chemical perturbation signatures to identify bioactive chemicals that can “revert” the disease signatures can lead to novel drug discovery and drug repurposing. Although, considerable research has been devoted to utilize bulk assay transcriptional data to study the relationship between diseases, genes, and drugs, considerably less attention has been paid to study the connection at the single cell level. Lately, single-cell RNA-seq (scRNA-seq) has emerged as a powerful tool to study gene expression of individual cells, providing a better understanding of complex disease mechanisms at single-cell resolution. In this thesis, we developed analytical methods to construct scRNA-seq derived transcriptional signatures and connected these signatures to LINCS-L1000 perturbational signatures. Utilizing the developed methods, we analyzed scRNA-seq data from Lymphangioleiomyomatosis (LAM) which is a rare pulmonary disease affecting primarily women of childbearing age. Our connectivity analysis identified MTOR inhibitors as candidates for reverting the LAM signature while the corresponding standard bulk analysis did not. This indicates the importance of using single cell analysis in constructing disease signatures instead of bulk tissue analysis. Furthermore, with the overall goal of removing technical roadblocks for reusing public domain ii transcriptomics data (both bulk and single-cell), we developed a web-application GREIN (GEO RNA-seq Experiments Interactive Navigator). The front-end user interfaces provide a wealth of user-analytics options including sub-setting and downloading processed data, interactive visualization, statistical power analyses, construction of differential gene expression signatures and their comprehensive functional characterization, and connectivity analysis with LINCS L1000 data. iii iv Acknowledgements I would like to take this opportunity to express my sincere gratitude to all the people who supported me with their advice, suggestion, and encouragement over the course of my doctoral study. First of all, I would like to thank my advisor, Mario Medvedovic, PhD, who was consistently supportive, extremely insightful, and one of the best advisors I have ever worked with. Without his invariable guidance, patience, and motivation throughout my time at the University of Cincinnati, I would be adrift. I would like to extend my gratitude to my thesis committee members, Jane Yu, PhD, Jaroslaw Meller, PhD, and Siva Sivaganesan, PhD, for their invaluable inputs, suggestions, and feedbacks to fine- tune this dissertation. I am gratefully indebted to Dr. Yu for her guidance, time, and support in LAM research, especially in understanding the biological background of LAM. I am thankful to Dr. Meller for his instrumental comments and ideas for improving this work. I would also like to thank Dr. Sivaganesan for his support and helpful advice. Thanks to all my past and present easy-going lab mates for helping me in daily lab activities and making my time in the lab memorable. I would also like to acknowledge our collaborators in the LINCS project who have helped me not only in the research activities but to improve my transferable skills as well. I would like to further express my gratitude to the faculties and administration of the Division of Biostatistics and Bioinformatics for their continuous support and resources to survive the grad school. Above all, completing my doctoral study would not be possible without continuous support of my family and friends. There are hardly any words to express my gratitude. To my mother and sister, who, despite the difficultly in their being 8,000 miles away, kept inspiring, motivating, and praying for me in every possible way. To my beloved wife Sawsan, my best friend and my support system, without whose unconditional support and obviously good food, I would not be able to come this far. I am indebted to my in-laws for their continuous encouragement throughout this journey. v Lastly, I would like to dedicate this work to my father, who had the dream to see me holding the doctoral degree and motivated me to go for graduate school. I wish he could see me fulfilling his dream in his lifetime, but I know he is watching me from the heaven. vi Table of Contents Abstract .................................................................................................................................................... ii Acknowledgements ................................................................................................................................. v List of Figures ......................................................................................................................................... ix List of Supplementary Figures............................................................................................................... x List of Tables .......................................................................................................................................... xi List of Supplementary Tables ............................................................................................................... xi Chapter 1 Introduction ............................................................................................................................... 12 Background ........................................................................................................................................... 12 Objective and Hypothesis ..................................................................................................................... 14 Chapter 2 Methods for single cell transcriptional signature connectivity analyses ................................... 15 Signature construction .......................................................................................................................... 15 Connectivity analysis ............................................................................................................................ 19 Chapter 3 Single-cell RNA-seq Data Analysis .............................................................................................. 23 Abstract .................................................................................................................................................. 24 Introduction ........................................................................................................................................... 25 Results .................................................................................................................................................... 27 Overview of scRNA-seq connectivity analysis ................................................................................ 27 Signature construction and connectivity analysis of naïve LAM ................................................. 27 Cluster analysis of naïve LAM and wild-type samples .................................................................. 28 Construction of cluster annotating signatures ............................................................................... 28 Construction of disease characterizing signatures ......................................................................... 30 Connectivity analysis ........................................................................................................................ 32 Signature construction and connectivity analysis of sirolimus treated LAM .............................. 35 Discussion .............................................................................................................................................. 39 Methods .................................................................................................................................................. 41 Single-cell RNA-seq and LINCS-L1000 data ................................................................................. 41 Single-cell RNA-seq data pre-processing and clustering
Details
-
File Typepdf
-
Upload Time-
-
Content LanguagesEnglish
-
Upload UserAnonymous/Not logged-in
-
File Pages109 Page
-
File Size-