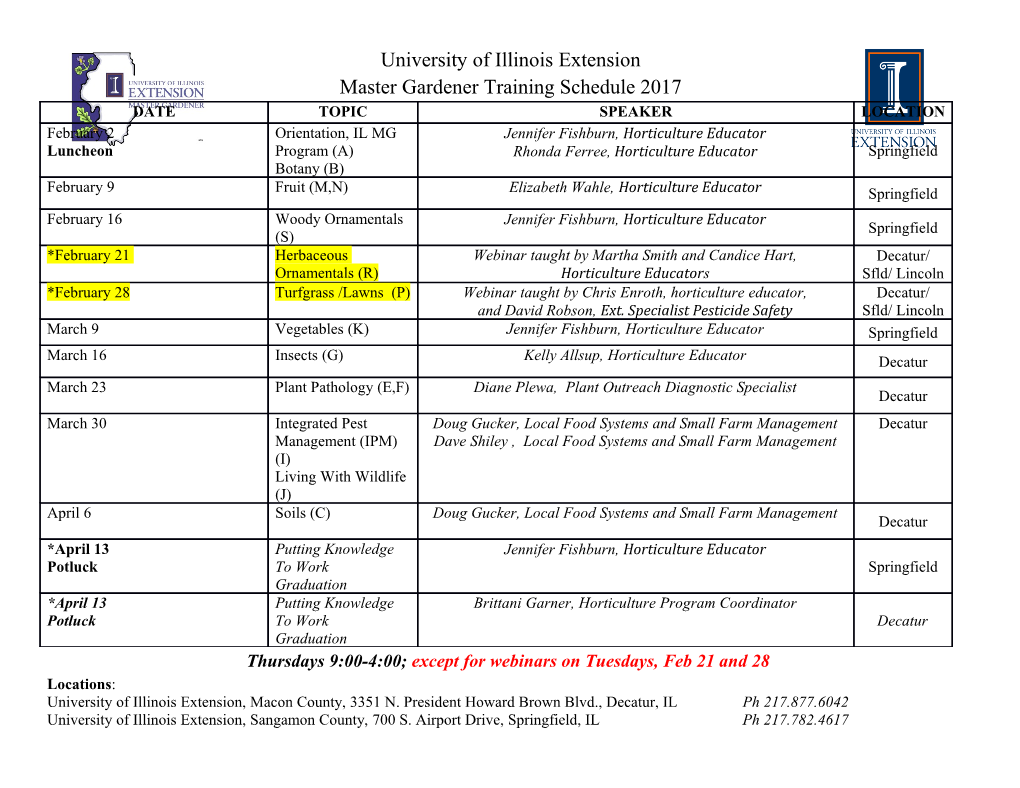
WHY MULTIVARIATE ANALYSIS? • Used for analysing complicated data sets • When there are many Independent Variables (IVs) and/or many Dependent Variables (DVs) • When IVs and DVs are correlated with one Dr. Azmi Mohd Tamil another to varying degrees Jabatan Kesihatan Masyarakat Fakulti Perubatan UKM • When need to come up with Prediction Model Based on lecture notes by Dr Azidah Hashim • Parallels greater complexity of contemporary research USING MULTIVARIATE ANALYSIS CHOICE OF APPROPRIATE STATISTICAL METHOD BASED ON: • WHICH STATISTICAL PROCEDURE TO USE? • Nature of IVs and DVs • HOW TO PERFORM CHOSEN PROCEDURE? • Investigator’s Experience • Personal Preferences • HOW TO INFER FROM RESULTS OBTAINED? • Ease of Comfort with Methods Used • Literature Review References • ANY OTHER ALTERNATIVE APPROACH? • Consultation with Statistician BASIC PREMISE I: BASIC PREMISE I: TERM USED:- Relationship between X Y X Y Independent Variable (IV) Dependent Variable (DV) Predictor Outcome Explanatory Response Eg . Smoking Lung Cancer Risk Factor Effect Age Hypertension Covariates (Continuous) Maternal ANC Birthweight Factor (Categorical) Control INDEPENDENT DEPENDENT VARIABLE VARIABLE Confounders RISK FACTOR OUTCOME Nuisance 1 BASIC PREMISE II: TERMINOLOGY: Univariate Analysis DATA • Analysis in which there is a single DV Bivariate Analysis Qualitative Quantitative • Analysis of two variables (categorical) • Wish to simply study the relationship between -Dichotomous -Continuous the variables - Polynomial -Discrete Multivariate Analysis • Simultaneously analyse multiple DVs and IVs Rough Guide to Multivariate BASIC PREMISE 111:VARIATIONS OF Methods (1) THE SAME THEME Name Xs Y THE GENERAL LINEAR MODEL: Regression and Continuous Continuous Correlation (eg. age) (eg. BP) Y = a + b1x1 + b2x2 + b3x3 …. + bixi Analysis of Variance Categorical Continuous Used in the following procedures: (ANOVA) (eg. SES) (eg. BP) ANOVA Analysis of Categorical Continuous ANCOVA Covariance and Continuous (eg BP) Multiple Linear Regression (ANCOVA) (eg age and SES) Multiple Logistic Regression Log Linear Regression Discriminant Function Rough Guide to Multivariate Methods (2) BASIC PREMISE IV: Name Xs Y Multiple Linear Continuous Continuous Regression (eg. Age, Ht, (eg. BP) Wt) TWO APPROACHES TO CHOOSING: Logistic Regression Continuous Categorical (eg. age) (eg. CHD) 1. Based on Type of Modelling Logistic Regression Categorical Categorical (eg. sex) (eg. CHD) 2. Based on Type of Research Question Discriminant Continuous Nominal / Function Analysis (eg. Age, Ordinal Income) (eg. Quality of Life) 2 MODELLING: TWO TYPES OF MODELLING TOOLS: Prior Knowledge Discovery 1. Theory Driven-Hypothesis Testing: Attempts to substantiate or disprove preconceived ideas Model 2. Data Driven: Building Validation Deployment Automatically creates model based on patterns found in data THEORY-DRIVEN MODELLING TOOLS: DATA-DRIVEN MODELLING TOOLS: CORRELATIONS CLUSTER ANALYSIS t-TESTS FACTOR ANALYSIS ANOVA DECISION TREES LINEAR REGRESSION DATA VISUALISATION LOGISTIC REGRESSION NEURAL NETWORKS DISCRIMINANT ANALYSIS FORECASTING METHODS BASIC PREMISE IV: RESEARCH QUESTION I: Degree of Relationship among Variables Types of Research Questions Statistical technique: • Degree of Relationship among a. Bivariate r (Bivariate correlation and Variables Regression) • Significance of Group Differences b. Multiple R ( Multiple Correlation and Multiple Regression) • Prediction of Group Membership c. Sequential R • Structure d. Canonical R e. Multiway Frequency Analysis 3 RESEARCH QUESTION II: RESEARCH QUESTION III Significance of Group Differences Prediction of Group Membership Statistical Techniques: Statistical technique a. t-test b. One-way ANOVA c. Two-way ANOVA a. Discriminant Function d. Profile Analysis b. Multiway Frequency Analysis (Logit) c. Logistic Regression PRELIMINARY CHECK OF DATA RESEARCH QUESTION IV BEFORE MULTIVARIATE ANALYSIS Structure • Accuracy of Data File • Honest Correlation • Missing Data Statistical technique: •Outliers a. Principal Component Analysis • Normality, linearity and homoscedasticity Regression b. Factor Analysis • Multicollinearity and Singularity Diagnostics c. Structural Equation Modelling • Common Data Transformations Univariate outliers ACCURACY OF DATA FILE : 600 Inspect univariate descriptive statistics for accuracy of input 500 73 a. Out-of-range values 400 b. Plausible means and standard deviation 300 c. Coefficient of variation d. Univariate outliers 200 100 141198211181 0 N = 184 Pre-pregnancy weight 4 Inflated Correlation HONEST CORRELATIONS • If composite variables are to be used and • Inflated Correlation two or more composite variables have the • Deflated Correlation same raw data, correlation can be inflated. • Inaccurately Completed • i.e. correlation between BMI and weight Deflated Correlation Inaccurately Completed 1) the range of values for one variable is restricted; “relationship between annual average daily traffic count • Questionnaires inaccurately completed due (AADT) and accidents on rural highways and picks a remote region where all AADT's are less than 3000, then to lack of time, lack of concern, emotional if there is a good correlation, he is likely to bias will affect correlation. underestimate it with such a restricted range on one variable. 2) if an intervening variable mediates between two variables; “relationship between thickness of asphalt and chloride content, then picking only those bridges in the population which have a waterproofing membrane will likely push down the estimate. The waterproofing membrane intervenes, literally and statistically. ” MISSING DATA MISSING DATA How to handle missing data a. Deleting cases or variables Seriousness depends on Random b. Estimating missing data • Pattern of missing data Non-Random - use of prior knowledge • How much is missing - inserting mean values • Why is it missing - using regression c. Using a missing data correlation matrix d. Treating missing data as data e. Repeating analyses with and without missing data 5 OUTLIERS • Cases with such extreme values on one OUTLIERS : variable or a combination of variables that they distort statistics • Presence due to Measure of Impact : Leverage - incorrect data entry Discrepancy - failure to specify missing value codes Influence - outlier is not a member of target population - distribution for variable in population is more skewed than normal The relationship among leverage, discrepancy and influence x OUTLIERS : x Dealing with Outliers High leverage, low discrepancy, High leverage, high discrepancy, • Find and remedy errors in data entry moderate influence high influence • Find and remedy missing values specification • Deletion • Retention with alteration x Low leverage, high discrepancy, moderate influence NORMALITY, LINEARITY AND OUTLIERS : HOMOSCEDASCITY Detecting Outliers Need for Multivariate Normality Univariate outliers: - assumption that each variable and all linear - inspection of z-scores combinations of the variables are normally distributed - graphical methods e.g. histograms, box plots, normal probability plots - robustness to violation of assumption still inconclusive Multivariate outliers: - computation of Mahalanobis distance 6 NORMALITY Normal - assessed via statistical or graphical methods - 2 components: skewness and kurtosis - if non-normal, consider transformation Positive skewness Negative skewness Positive kurtosis Negative kurtosis Predicted Y’ Predicted Y’ Curvilinear Relationship ) s r Assumptions o r Failure of r e Met ( Normality s l a u d i s e R (a) (b) Number of symptoms of Number Number of symptoms of Number ) Low Moderate High Low Moderate High s r o r r DOSAGE DOSAGE e Heteroscedasticity Non- ( s Linearity l a (a) Curvilinear (a) Curvilinear + linear u d i s e R (c) (d) HOMOSCEDASTICITY LINEARITY - assumption that the variability in scores for one continuous variable is roughly the - assumption of a straight line relationship same at all values of another continuous between 2 variables variable - Nonlinearity is diagnosed from -failure due to • residuals plots or • non normality of one of the variables or • bivariate scatterplots • one variable is related to some transformation of the other 7 COLLINEARITY PRESENCE OF COLLINEARITY CAUSES - concerns the relationship of the IVs to one another and does not directly - Unstable regression coefficient estimates involve the response variable - Large estimates of coefficient variances eg. - Wide 95% Confidence Limits Physical Activity Blood -Large p-values Collinearity Pressure - Large Standard errors Age MULTICOLLINEARITY AND PROBLEMS WITH MULTICOLLINEARITY SINGULARITY AND SINGULARITY (related to a Correlation Matrix) Multicollinearity : Variables are too highly correlated (> 0.90) - inflate size of error - weaken analysis Singularity : Variables are redundant; Matrix cannot be inversed the variables are perfectly correlated. expose the redundancy of variables and the need to remove variables from the analysis. TYPES OF TRANSFORMATIONS COMMON DATA TRANSFORMATION Square root Logarithm Inverse • recommended as a remedy for outliers and for failures of normality, linearity and homoscedasticity Reflect and square Reflect and logarithm Reflect and inverse root 8 MULTIVARIATE RELATIONSHIP APPLICATIONS OF MULTIVARIATE ANALYSIS X a) The primary purpose is to study the effect on eg. age variable Y of changes in a particular single variable Y X1, but it is recognised that Y may be affected by several other variables X,X, … The effect on Y of eg. CHD X simultaneous changes in
Details
-
File Typepdf
-
Upload Time-
-
Content LanguagesEnglish
-
Upload UserAnonymous/Not logged-in
-
File Pages17 Page
-
File Size-