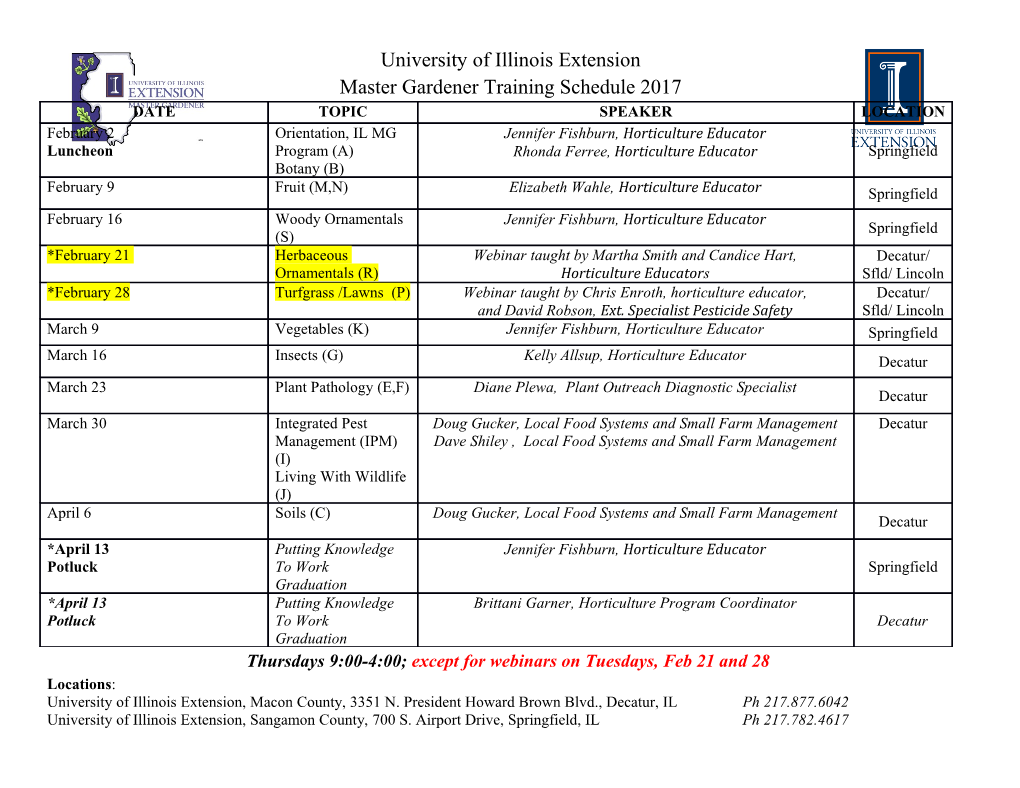
Entity Set Expansion using Topic information Kugatsu Sadamitsu, Kuniko Saito, Kenji Imamura and Genichiro Kikui∗ NTT Cyber Space Laboratories, NTT Corporation 1-1 Hikarinooka, Yokosuka-shi, Kanagawa, 239-0847, Japan sadamitsu.kugatsu, saito.kuniko, imamura.kenji @lab.ntt.co.jp { [email protected] } Abstract pointwise mutual information (PMI). Thelen and Riloff (2002), Ghahramani and Heller (2005) and This paper proposes three modules based on Sarmento et al. (2007) also proposed original score latent topics of documents for alleviating “se- functions with the goal of reducing semantic-drift. mantic drift” in bootstrapping entity set ex- Our purpose is also to reduce semantic drift. For pansion. These new modules are added to a achieving this goal, we use a discriminative method discriminative bootstrapping algorithm to re- alize topic feature generation, negative exam- instead of a scoring function and incorporate topic ple selection and entity candidate pruning. In information into it. Topic information means the this study, we model latent topics with LDA genre of each document as estimated by statisti- (Latent Dirichlet Allocation) in an unsuper- cal topic models. In this paper, we effectively uti- vised way. Experiments show that the accu- lize topic information in three modules: the first racy of the extracted entities is improved by generates the features of the discriminative mod- 6.7 to 28.2% depending on the domain. els; the second selects negative examples; the third prunes incorrect examples from candidate examples 1 Introduction for new entities. Our experiments show that the pro- posal improves the accuracy of the extracted entities. The task of this paper is entity set expansion in The remainder of this paper is organized as fol- which the lexicons are expanded from just a few lows. In Section 2, we illustrate discriminative boot- seed entities (Pantel et al., 2009). For example, strapping algorithms and describe their problems. the user inputs a few words “Apple”, “Google” and Our proposal is described in Section 3 and experi- “IBM” , and the system outputs “Microsoft”, “Face- mental results are shown in Section 4. Related works book” and “Intel”. are described in Section 5. Finally, Section 6 pro- Many set expansion algorithms are based on boot- vides our conclusion and describes future works. strapping algorithms, which iteratively acquire new entities. These algorithms suffer from the general 2 Problems of the previous Discriminative problem of “semantic drift”. Semantic drift moves Bootstrapping method the extraction criteria away from the initial criteria Some previous works introduced discriminative demanded by the user and so reduces the accuracy methods based on the logistic sigmoid classifier, of extraction. Pantel and Pennacchiotti (2006) pro- which can utilize arbitrary features for the relation posed Espresso, a relation extraction method based extraction task instead of a scoring function such as on the co-training bootstrapping algorithm with en- Espresso (Bellare et al., 2006; Mintz et al., 2009). tities and attributes. Espresso alleviates semantic- Bellare et al. reported that the discriminative ap- drift by a sophisticated scoring system based on proach achieves better accuracy than Espresso when ∗ Presently with Okayama Prefectural University the number of extracted pairs is increased because 726 Proceedings of the 49th Annual Meeting of the Association for Computational Linguistics:shortpapers, pages 726–731, Portland, Oregon, June 19-24, 2011. c 2011 Association for Computational Linguistics multiple features are used to support the evidence. However, three problems exist in their methods. First, they use only local context features. The dis- criminative approach is useful for using arbitrary features, however, they did not identify which fea- ture or features are effective for the methods. Al- though the context features and attributes partly re- duce entity word sense ambiguity, some ambiguous entities remain. For example, consider the domain broadcast program (PRG) and assume that PRG’s attribute is advertisement. A false example is shown here: “Android ’s advertisement employs Japanese Figure 1: The structure of our system. popular actors. The attractive smartphone begins to target new users who are ordinary people.” The en- tity Android belongs to the cell-phone domain, not sic adjustment numbers. The entity-attribute pairs PRG, but appears with positive attributes or contexts are obtained by taking the cross product of seed en- because many cell-phones are introduced in adver- tity lists and attribute lists. The pairs are used as tisements as same as broadcast program. By us- queries for retrieving the positive documents, which D ing topic, i.e. the genre of the document, we can include positive pairs. The document set e,a in- e, a distinguish “Android” from PRG and remove such cluding same entity-attribute pair is regarded E { } false examples even if the false entity appeared with as one example e,a to alleviate over-fitting for con- positive context strings or attributes. Second, they text features. These are called positive examples in did not solve the problem of negative example se- Figure 1. Once positive examples are constructed, lection. Because negative examples are necessary discriminative models can be trained by randomly for discriminative training, they used all remaining selecting negative examples. examples, other than positive examples, as negative Candidate entities are restricted to only the examples. Although this is the simplest technique, Named Entities that lie in the close proximity to the it is impossible to use all of the examples provided positive attributes. These candidates of documents, by a large-scale corpus for discriminative training. including Named Entity and positive attribute pairs, Third, their methods discriminate all candidates for are regarded as one example the same as the train- new entities. This principle increases the risk of gen- ing data. The discriminative models are used to cal- erating many false-positive examples and is ineffi- culate the discriminative positive score, s(e, a), of each candidate pair, e, a . Our system extracts Nn cient. We solve these three problems by using topic { } information. types of new entities with high scores at each iter- ation as defined by the summation of s(e, a) of all positive attributes (A ); s(e, a). Note that 3 Set expansion using Topic information P a AP we do not iteratively extract∈ new attributes because 3.1 Basic bootstrapping methods our purpose is entity set expansion.∑ In this section, we describe the basic method 3.2 Topic features and Topic models adopted from Bellare (Bellare et al., 2006). Our system’s configuration diagram is shown in Figure In previous studies, context information is only used 1. In Figure 1, arrows with solid lines indicate the as the features of discriminative models as we de- basic process described in this section. The other scribed in Section 2. Our method utilizes not only parts are described in the following sections. After context features but also topic features. By utiliz- Ns positive seed entities are manually given, every ing topic information, our method can disambiguate noun co-occurring with the seed entities is ranked the entity word sense and alleviate semantic drift. by PMI scores and then selected manually as Na In order to derive the topic information, we utilize positive attributes. Ns and Na are predefined ba- statistical topic models, which represent the relation 727 between documents and words through hidden top- the candidate set by positive attributes, however, this ics. The topic models can calculate the posterior is not enough as described in Section 2. Our candi- probability p(z d) of topic z in document d. For date pruning module, described below, uses the mea- example, the topic| models give high probability to sure of topic similarity to remove obviously incor- topic z =”cell-phone” in the above example sen- rect documents. tences 1. This posterior probability is useful as a This pruning module is similar to negative exam- global feature for discrimination. The topic feature ple selection described in the previous section. The value φt(z, e, a) is calculated as follows. positive topic score, PT , is used as a candidate con- straint. Taking all positive examples, we select the p(z d) d De,a positive topics, PZ, which including all topics z sat- φt(z, e, a) = ∈ | . p(z0 d) isfying the condition PT (z) > th. At least one z∑0 d De,a | ∈ topic with the largest score is chosen as a positive ∑ ∑ In this paper, we use Latent Dirichlet Allocation topic when PT (z) th about all topics. After se- (LDA) as the topic models (Blei et al., 2003). LDA lecting this positive≤ topic, the documents including represents the latent topics of the documents and the entity candidates are removed if the posterior prob- co-occurrence between each topic. ability satisfy p(z d) th for all topics z. In this In Figure 1, shaded part and the arrows with bro- paper, we set the threshold| ≤ to th = 0.2. This con- ken lines indicate our proposed method with its use straint means that the topic of the document matches of topic information including the following sec- that of the positive entities and can be regarded as a tions. hard constraint for topic features. 3.3 Negative example selection 4 Experiments If we choose negative examples randomly, such ex- amples are harmful for discrimination because some 4.1 Experimental Settings examples include the same contexts or topics as the We use 30M Japanese blog articles crawled in May positive examples. By contrast, negative examples 2008. The documents were tokenized by JTAG belonging to broad genres are needed to alleviate se- (Fuchi and Takagi, 1998), chunked, and labeled with mantic drift. We use topic information to efficiently IREX 8 Named Entity types by CRFs using Mini- select such negative examples. mum Classification Error rate (Suzuki et al., 2006), In our method, the negative examples are cho- and transformed into features.
Details
-
File Typepdf
-
Upload Time-
-
Content LanguagesEnglish
-
Upload UserAnonymous/Not logged-in
-
File Pages6 Page
-
File Size-