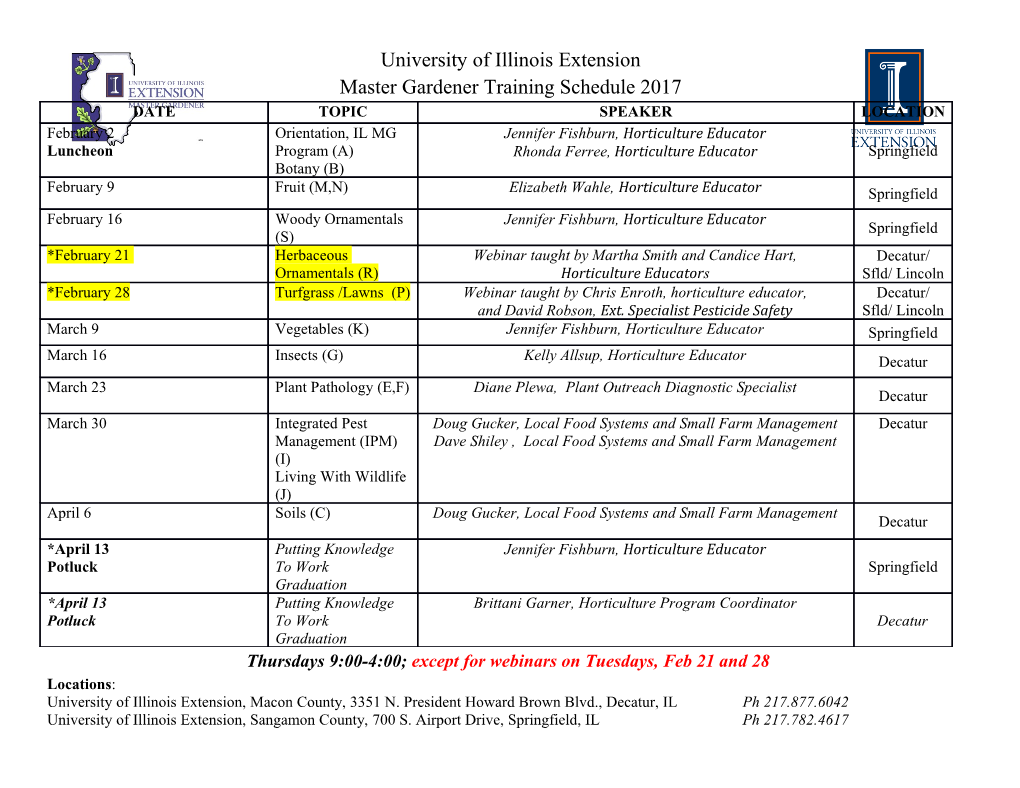
Patron: Her Majesty The Queen Rothamsted Research Harpenden, Herts, AL5 2JQ Telephone: +44 (0)1582 763133 WeB: http://www.rothamsted.ac.uk/ Rothamsted Repository Download A - Papers appearing in refereed journals Sinhuber, M., Van der Vaart, K., Yenchia, F., Reynolds, A. M. and Ouellette, N.T. 2021. An equation of state for insect swarms. Scientific Reports. 11 (3773). https://doi.org/10.1038/s41598-021-83303-z The publisher's version can be accessed at: • https://doi.org/10.1038/s41598-021-83303-z The output can be accessed at: https://repository.rothamsted.ac.uk/item/9832x/an- equation-of-state-for-insect-swarms. © 12 February 2021, Please contact [email protected] for copyright queries. 14/02/2021 15:41 repository.rothamsted.ac.uk [email protected] Rothamsted Research is a Company Limited by Guarantee Registered Office: as above. Registered in England No. 2393175. Registered Charity No. 802038. VAT No. 197 4201 51. Founded in 1843 by John Bennet Lawes. 1 An equation of state for insect swarms 2 Michael Sinhuber1,#,†, Kasper van der Vaart1,#, Yenchia Feng1, Andrew M. Reynolds2, and 3 Nicholas T. Ouellette1,* 4 5 1Department of Civil and Environmental Engineering, Stanford University, Stanford, CA 94305, 6 USA. 7 2Rothamsted Research, Harpenden, Hertfordshire, AL5 2JQ, United Kingdom. 8 9 #These authors contributed equally to this work. 10 †Present address: Carl von Ossietzky Universität Oldenburg, 26129 Oldenburg, Germany. 11 *Correspondence: Nicholas T. Ouellette (email: [email protected]) 12 13 Collective behaviour in flocks, crowds, and swarms occurs throughout the biological world. 14 Animal groups are generally assumed to be evolutionarily adapted to robustly achieve 15 particular functions, so there is widespread interest in exploiting collective behaviour for 16 bio-inspired engineering. However, this requires understanding the precise properties and 17 function of groups, which remains a challenge. Here, we demonstrate that collective groups 18 can be described in a thermodynamic framework. We define an appropriate set of state 19 variables and extract an equation of state for laboratory midge swarms. We then drive 20 swarms through “thermodynamic” cycles via external stimuli, and show that our equation 21 of state holds throughout. Our findings demonstrate a new way of precisely quantifying the 22 nature of collective groups and provide a cornerstone for potential future engineering design. 23 24 Introduction 25 Organisms on every size scale, from single-celled1 to highly complex2, regularly come together in 26 groups. In many cases, such aggregations are collective, in that the group as a whole displays 27 properties and functionality distinct from those of its individual members or simply their linear 28 sum3,4. It is generally assumed that since evolution has led so many different kinds of animals to 29 behave collectively, the performance of collective groups at whatever task they seek to achieve 30 ought to be well beyond the capabilities of a single individual5, while also being robust to uncertain 31 natural environments6,7 and operating without the need for top-down control8. For these reasons, 32 there has been significant interest both in understanding how collectivity conveys these 33 advantages9 and how to exploit it in engineered systems10,11. 34 35 Taking advantage of evolutionary adaptation for the design of such a bio-inspired artificial 36 collective system requires both determining the interaction rules used by real animals and properly 37 understanding the function of the group. Both of these tasks remain a challenge. Extracting 38 interaction rules by observing group behaviour is a highly nontrivial inverse problem12 that can 39 typically only be solved by assuming a modelling framework a priori13,14. Appropriate model 40 selection is made more difficult given that interactions may change in different contexts7,8,15. Even 41 less work has been done to precisely determine the tasks optimized by collective behaviour. 42 Assumptions about the purpose of group behaviour typically come from ecological reasoning16 43 rather than quantitative empirical evidence8—and in some cases, such as hypothesized 44 aerodynamic benefits conveyed to flocking birds, such reasoning has proved to be incorrect17,18. 45 46 We argue that the essential nature of the group functionality is encoded in its properties—and 47 therefore that understanding these properties both allows one to quantify the purpose of the 48 collective behaviour and to predict the response of the group to environmental changes. As recent 49 work has demonstrated19-21, a powerful way to characterize these properties is to borrow ideas 50 from other areas of physics. For groups on the move such as human crowds, hydrodynamics is a 51 natural choice, and empirically measured constitutive laws have allowed the formulation of 52 equations of motion that accurately predict how crowds flow20. But for stationary groups such as 53 insect swarms, where the group as a whole does not move even though its constituent individuals 54 are continuously rearranging, thermodynamics is a more natural framework, as it allows one to 55 precisely describe the state of the system irrespective of its net motion22. The most fundamental 56 relationship for doing so is the equation of state, which links the state variables that describe the 57 macroscopic properties of the system and encodes how they co-vary in response to environmental 58 changes. 59 60 Here, we formulate such an equation of state for laboratory swarms of the non-biting midge 61 Chironomus riparius (Fig. 1a). We define appropriate state variables, and empirically deduce their 62 relationship by analysing a large data set of measured swarms23. Then, by applying a suitable 63 sequence of external perturbations to the swarms, we show that we can drive them through a 64 thermodynamic cycle in pressure–volume space throughout which our empirical equation of state 65 holds. 66 67 68 69 Results 70 State variables. The first step in describing the macroscopic properties of the swarm is to define 71 a set of state variables that fully characterizes the state of the system. The equation of state then 72 links these state variables in a functional relation. In classical thermodynamics, a complete set of 73 state variables is given by the conjugate pairs of pressure P and volume V, temperature T and 74 entropy S, and, if the number of particles is not fixed, chemical potential µ and number of particles 75N. We use an analogous set of state variables here to characterize swarms. The most 76 straightforward state variable to define is the number of individuals N, which is given simply by 77 the number of midges that are participating in the swarm at a given time (note that midges that are 78 not swarming simply sit on the walls or floor of the laboratory enclosure). The volume V of the 79 swarm can be straightforwardly defined and computed as the volume of the convex hull enclosing 80 all the midges. Note that, while N and V are not independently controllable quantities, the ratio 81N/V is empirically approximately constant in large swarms 25, meaning that the “thermodynamic” 82 limit (that is, and with N ) is approached in our swarms33. In typical swarming N→∞ V→∞ V→ ρ 83 events, N changes on a time scale that is very slow compared to the swarm dynamics; thus, a 84 chemical potential is not needed to describe the instantaneous state of the swarm. Note, though, 85 that since the number of midges varies between measurements that may be separated by many 86 days, N remains a relevant state variable for capturing swarm-to-swarm variability. 87 88 The remaining three state variables are somewhat more subtle, but can be defined by building on 89 previous work. It has been explicitly shown24 that a virial relation based on the kinetic energy and 90 an effective potential energy holds for laboratory swarms of Chironomus riparius. For particles 91 moving in a potential, this virial relation can be used to define a pressure24. As we have shown 92 previously, swarming midges behave as if they are trapped in a harmonic potential well that binds 93 them to the swarm, with a spring constant k(N) that depends on the swarm size24,25 (Fig. 1b). The 94 difference between the kinetic energy and this harmonic potential energy thus allows us to compute 95 a pressure4,24,26, which is conceptually similar to the swim pressure defined in other active 96 systems27. The virial theorem thus provides a link between kinetic energy, potential energy, and a 97 field that plays the role of a pressure, when coupled with the observation that individual midges to 98 a good approximation behave as if they are moving in a harmonic potential24,25. We can write this 99 virial pressure P (per unit mass, assuming a constant mass per midge) as N 1 2 1 2 100 P = vi - 〈k〈ri , 3NV ∑ ( 2 ) i = 1 101 where N is the number of midges in the swarm, V is the swarm volume, vi is the velocity of midge 102i, r i is its distance from the swarm centre of mass, and 〈k〈 = 〈 -ai ∙ ri / ri〈 is the effective 103 spring constant of the emergent potential well that binds midges to the swarm. In this expression, 104a i is the acceleration of midge i, ri is the unit vector pointing from a midge towards the centre of 105 mass of the swarm (defined as given by N ) and averages are taken over the individuals 1/N∑i = 1ri 106 in the swarm. This spring constant depends on the swarm size N (Fig. 1b). We note that we have 107 previously simply used the directly computed potential energy - 〈ai ∙ ri〈 to define the 108 pressure4,26; here, we instead average the potential terms and fit them to a power law in N (Fig.
Details
-
File Typepdf
-
Upload Time-
-
Content LanguagesEnglish
-
Upload UserAnonymous/Not logged-in
-
File Pages23 Page
-
File Size-