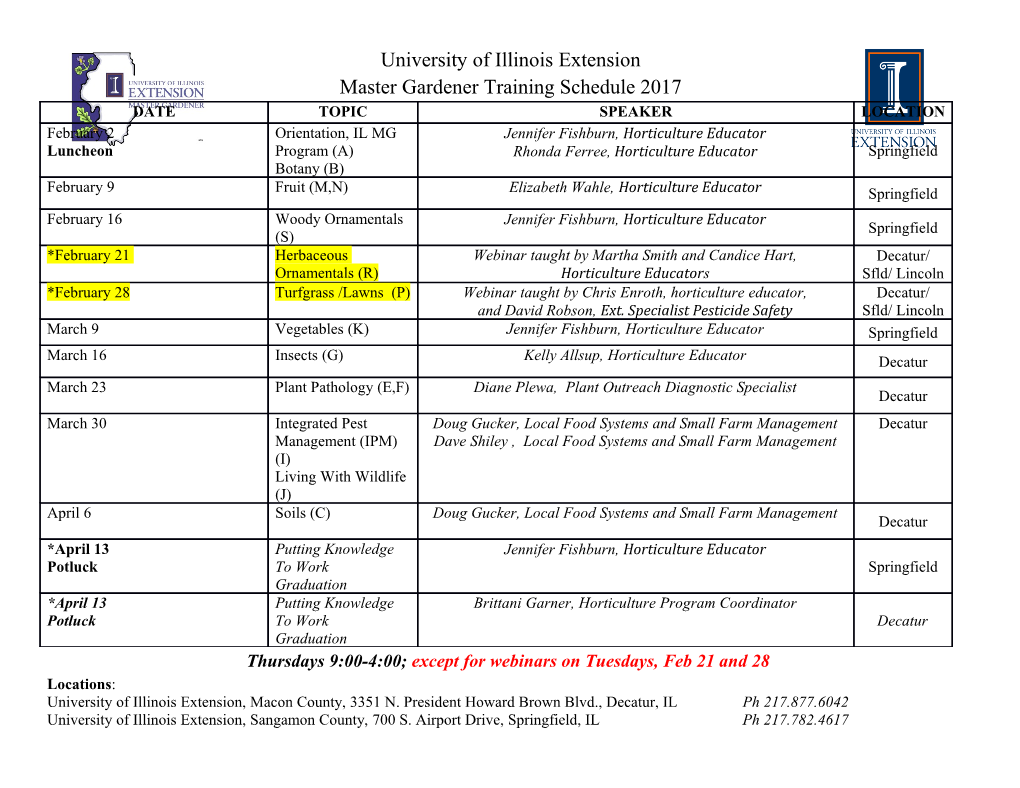
Wofford and Lindahl BMC Systems Biology (2019) 13:23 https://doi.org/10.1186/s12918-019-0702-2 RESEARCH ARTICLE Open Access A mathematical model of iron import and trafficking in wild-type and Mrs3/4ΔΔ yeast cells Joshua D. Wofford1 and Paul A. Lindahl1,2* Abstract Background: Iron plays crucial roles in the metabolism of eukaryotic cells. Much iron is trafficked into mitochondria where it is used for iron-sulfur cluster assembly and heme biosynthesis. A yeast strain in which Mrs3/4, the high- affinity iron importers on the mitochondrial inner membrane, are deleted exhibits a slow-growth phenotype when grown under iron-deficient conditions. However, these cells grow at WT rates under iron-sufficient conditions. The object of this study was to develop a mathematical model that could explain this recovery on the molecular level. Results: A multi-tiered strategy was used to solve an ordinary-differential-equations-based mathematical model of iron import, trafficking, and regulation in growing Saccharomyces cerevisiae cells. At the simplest level of modeling, all iron in the cell was presumed to be a single species and the cell was considered to be a single homogeneous volume. Optimized parameters associated with the rate of iron import and the rate of dilution due to cell growth were determined. At the next level of complexity, the cell was divided into three regions, including cytosol, mitochondria, and vacuoles, each of which was presumed to contain a single form of iron. Optimized parameters associated with import into these regions were determined. At the final level of complexity, nine components were assumed within the same three cellular regions. Parameters obtained at simpler levels of complexity were used to help solve the more complex versions of the model; this wasadvantageousbecausethedatausedfor solving the simpler model variants were more reliable and complete relative to those required for the more complex variants. The optimized full-complexity model simulated the observed phenotype of WT and Mrs3/4ΔΔ cells with acceptable fidelity, and the model exhibited some predictive power. Conclusions: The developed model highlights the importance of an FeII mitochondrial pool and the necessary exclusion of O2 in the mitochondrial matrix for eukaryotic iron-sulfur cluster metabolism. Similar multi-tiered strategies could be used for any micronutrient in which concentrations and metabolic forms have been determined in different organelles within a growing eukaryotic cell. Keywords: Ordinary differential equations, Nanoparticles, Iron-sulfur clusters, Mitochondria, Vacuoles, Cytosol, Kinetics, Oxygen Background kinetic models [1–3]. In principle, such models can reveal The complexity of biochemical processes in growing on a quantitative basis whether observed phenotypic be- eukaryotic cells is enormous, often rendering the corre- havior could emerge from a proposed system of reacting sponding genetic phenotypes difficult to understand at the chemical players using a particular set of kinetic and chemical level. One means of analyzing such systems is to thermodynamic parameters. This is a huge advantage rela- develop ordinary-differential-equation (ODE1)-based tive to the common practice of describing complex bio- chemical processes as a cartoon or scheme. Another * Correspondence: [email protected] advantage of math-based kinetic models is that all as- 1Texas A&M University, Department of Chemistry, College Station, TX sumptions are explicit and available for public inspection 77843-3255, USA 2Texas A&M University, Department of Biochemistry & Biophysics, College whereas cartoons and schemes generally include hidden Station 77843-3255, USA assumptions. The major disadvantage of such kinetic © The Author(s). 2019 Open Access This article is distributed under the terms of the Creative Commons Attribution 4.0 International License (http://creativecommons.org/licenses/by/4.0/), which permits unrestricted use, distribution, and reproduction in any medium, provided you give appropriate credit to the original author(s) and the source, provide a link to the Creative Commons license, and indicate if changes were made. The Creative Commons Public Domain Dedication waiver (http://creativecommons.org/publicdomain/zero/1.0/) applies to the data made available in this article, unless otherwise stated. Wofford and Lindahl BMC Systems Biology (2019) 13:23 Page 2 of 16 models is that a complete and accurate dataset, including developed an ODE-based model for iron dysregulation rate-law expressions, rate-constants, and reactant concen- in cancer cells in which the roles of the IRP-based regu- trations, are required to solve them and to endow them lation, the iron storage protein ferritin, the iron export with predictive power. Rarely is all such information avail- protein ferroportin, the labile iron pool, reactive oxygen able, and available information is often less quantitative species, and the cancer-associated Ras protein were em- than desired. phasized [13], as well as a logical-rule-based mathemat- A common approach to circumventing this problem is ical model of iron homeostasis in healthy mammalian to employ simple models (in terms of numbers of com- cells [14]. Mitchell and Mendes used ODE’s to model ponents and reactions) that nevertheless remain capable iron metabolism and regulation in a liver cell and its of generating observed cellular behavior and of explain- interaction with blood plasma [15]. They emphasized ing genetic phenotypes. Designing such models involves the role of iron-regulating hormone hepcidin and the deciding which species and reactions to include, which regulatory and storage systems mentioned above, and to leave out, and which to combine into groups. Such they simulated the effects of iron-overload disease. Their decisions often boil-down to whether including an add- model was complex - involving 66 adjustable parameters itional component or reaction is “worth” (in terms of many of which were not experimentally determined. generating the desired behavior) an additional adjustable None of the above models included iron-sulfur cluster parameter. Simple models with few adjustable parame- (ISC) synthesis, the role of mitochondria (or other or- ters simplify reality but they can also provide fundamen- ganelles), and none modeled growing cells. In terms of tal insights into reality - by penetrating through the biological emphasis, the model of Achcar et al. [16]is entangled and bewildering complexity of a highly com- most relevant to the current study. They developed a plex system. model of iron metabolism and oxidative stress in yeast Iron is critical for all eukaryotic cells [4, 5]. It is cells using a Boolean approach using weighted reactions. present in many forms including heme centers, Their model included ISC assembly, as well as organelles iron-sulfur clusters (ISCs), nonheme mononuclear spe- such as mitochondria, vacuoles, cytosol, and nucleus. cies, and iron-oxo dimeric centers. Such centers are However, their model was exceedingly complicated (642 commonly found in the active-sites of metalloenzymes. components and 1007 reactions) and was not ODE Iron plays a major role in energy metabolism; e.g. there based [16]. They modeled the development of FeIII are iron-rich respiratory complexes located on the inner (phosphate) oxyhydroxide nanoparticles in mitochondria membrane of mitochondria. Mitochondria are the pri- of mutant cells lacking ISC assembly proteins (e.g. Yfh1, mary site in the cell where ISCs are assembled, and the the yeast frataxin homolog), similar to the emphasis of only site where iron is installed into porphyrins during our previous model [17]. They included a reaction in heme biosynthesis. For these reasons, mitochondria are which an unidentified species X converted nanoparticles a major ‘hub’ for iron trafficking. into free iron, and hypothesized that X might be gluta- The cytosol also plays an important role in iron traf- thione. In contrast, our model emphasized the role of ficking, in that nutrient iron enters this region prior to oxygen in controlling nanoparticle formation. being distributed to the organelles. Most of the iron that The iron content of yeast cells and the major organ- enters the cytosol is probably in the FeII state, but nei- elles involved in iron trafficking have been analyzed ther the oxidation state nor the concentration of cyto- using Mössbauer (MB) spectroscopy, the most powerful solic Fe has been established [6]. The vacuoles are spectroscopic tool for interrogating the iron content of another trafficking ‘hub’ in yeast, as much of the iron biological samples [18]. If the absolute iron concentra- imported into these cells (when grown on iron-sufficient tion of 57Fe-enriched cells and organelles are known, the media) is stored in these acidic organelles [7, 8]. Vacu- absolute concentrations of major groups of olar iron is predominately found as a mononuclear non- iron-containing species in such cells can be calculated heme high spin (NHHS) FeIII species, probably using percentages obtained by MB. Such data is used coordinated to polyphosphate ions [9]. here to develop an advanced mathematical model of iron Iron is tightly regulated in cells, and some insightful import and trafficking in eukaryotic cells. mathematical models involving iron metabolism, traf- In WT cells, much iron enters mitochondria through ficking and regulation have been developed. Twenty Mrs3 and Mrs4, paralogous inner-membrane proteins years ago, Omholt et al. designed and analyzed
Details
-
File Typepdf
-
Upload Time-
-
Content LanguagesEnglish
-
Upload UserAnonymous/Not logged-in
-
File Pages16 Page
-
File Size-