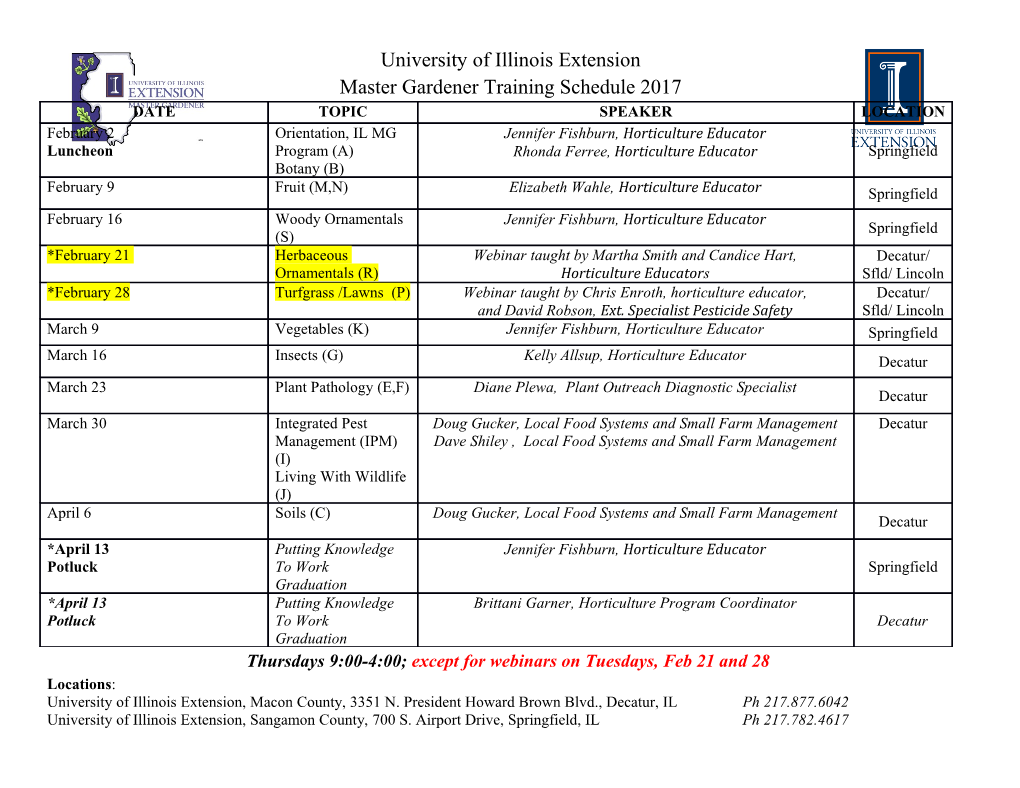
Title AdaptingName, the Wave Day Dataflow Month, Architecture 2019 to a Licensable AI IP Product Presented by Yuri Panchul, MIPS Open Technical Lead On SKOLKOVO Robotics & AI Conference. April 15-16, 2019 www.wavecomp.ai Wave + MIPS: A Powerful History of Innovation 2010 2012 2014 2016 2018 Wave founded by Dado Banatao as Developed Coarse Grain Delivered 11GHz test Announced Derek Meyer as CEO Wave acquires MIPS to deliver on its vision for of Wave Semiconductor, with a vision Reconfigurable Array (CGRA) chip at 28nm revolutionizing AI from the datacenter to the edge of ushering in a new era of AI semiconductor architecture Launched Early Access Program computing to enable data scientists to experiment Announced partnership with Broadcom and Samsung with neural networks to develop next-gen AI chip on 7nm node Launched MIPS Open initiative Closed Series D Round of funding at $86M, bringing total investment to $115M+ Expanded global footprint with offices in Beijing and Manila 2010 2011 2012 2013 2014 2015 2016 2017 2018 2019 2015 2011 2013 2017 2019 Renamed the company to Wave develops dataflow-based Wave expanded team to include MIPS business is sold by Created technology, providing higher Wave Computing to better Imagination Technologies to MIPS Open architecture, silicon and reflect focus on accelerating AI performance and scalability software expertise Tallwood Venture Capital as Advisory Board for AI applications with dataflow-based solutions Tallwood MIPS Inc. for $65M MIPS Technologies is sold to MIPS Powering 80% of MIPS Automotive MCUs MIPS introduces first Android-MIPS Imagination Technologies. MediaTek selects MIPS for LTE ADAS-enabled automobiles based Set top box at CES & ADAS engagements with modems MobilEye, Microchip & MStar Wave Joins Amazon, Facebook, Google selects MIPS architecture for use Google and Samsung to Support in its Android 3.0, "Honeycomb“ mobile Advanced AI Research at UC device Berkeley TALLWOOD Venture Capital Datacenter-to-Edge Needs Systems, Silicon and IP Compute Systems AI Processors AI Licensable IP “Deep learning is a fundamentally new software model. So we need a new computer platformTritonAI toTM run it...” Semiconductor Cloud Enterprise Partners AI Applications Software Autonomous Driving NLP Retail Smart Cities Wave Computing © 2019 3 What is Driving AI to the Edge? Market Drivers Networking Enterprise Mobile Industrial Autonomous IOT AI was born in AI Use Cases Datacenter“Deep learning is a fundamentally new software model. So we need a new computer platform to run it...” Privacy Security Isolated Low latency Cost Bandwidth Wave Computing © 2019 Storage Compute 4 Scalable AI Platform: TritonAI™ 64 Key Benefits: • Highly Scalable to address broad AI use cases MIPS64 + WaveFlowTM WaveTensorTM SIMD • Supports Inference and Training Multi-CPU High flexibility to High efficiency • High“Deep flexibility learning tois asupport fundamentally all AI algorithms new software model. RichSo weInstruction need a newsupport computer all AI platformfor key to CNNrun it...” algorithms AI algorithms Set Proven Tools • High efficiency for key AI CNN algorithms Mature Software • Configurable to support AI use cases • Mature Software Platform support Software Platform → Flexibility → Efficiency Wave Computing © 2019 5 Data Rates and New AI Networks Requirements Data Rates & Network Complexity Drive Data Compute Performance Demands (Inferences /sec) Data 100K Rates Aggregated Core Network Data GHz 10K Video to Radar Lidar 1K MHz Music to Imaging 100 KHz Data Compute Performance Compute Data Voice 10 Accelerometer Hz MIPS64+SIMD 1 0.01 W 0.1 W 1 W 10 W 100 W 1 KW **These curves represent the conceptional combination of these technologies, not actual independent performance.** Wave Computing © 2019 6 WaveTensor ™ Configurable Architecture Configurable Architecture for Tensor Processing Data 4x4 4x4 4x4 4x4 Fetch Tile Tile Tile Tiles Slice • Configurable MACs, Accumulation and Array Size Data 8x8 8x8 • Overlap of Communication & Computation Fetch Tile Tile N Tiles/Slice High Speed • Compatible datatypes with WaveFlow™ Core Buffer Data Confi Confi Confi Confi • Supports int8 for inferencing Fetch gTile gTile gTile gTile • Roadmap“Deep learning to bfloat16, is a fp32 fundamentally for training new software model. So we need a new computer platform to run it...” Data Confi Confi Confi Confi M Fetch gTile gTile gTile gTile Slices Configurable MAC units ResNet-50 Inferences/Sec* More Memory {4x4 | 8x8} Compute Density ~1K/mm2 MAX TOPs TOPs/Watt TOPs/mm2 Compute Efficiency ~500/watt 1024 8.3 10.1 • Core, Int8, 7nm Fin-FET nominal process/Vdd/temp • Core, Int8, 8x8 tile config, 7nm Fin-FET nominal • Batch=1, std model w/o pruning, performance process/Vdd/temp and power vary with array size/configuration Wave Computing © 2019 7 WaveFlow™ Reconfigurable Architecture dMEM dMEM dMEM dMEM dMEM dMEM dMEM dMEM • Configurable IMEM and DMEM Sizes PE-4 PE-5 PE-6 PE-7 PE-C PE-D PE-E PE-F iMEM iMEM iMEM iMEM iMEM iMEM iMEM • Overlap of communication & Computation iMEM i MAC MAC MAC MAC m MAC MAC MAC MAC • Compatible datatypes with WaveTensor™ e m • Integer (Int8, Int16, Int32) for inference PE-0 PE-1 PE-2 PE-3 PE-8 PE-9 PE-A PE-B iMEM iMEM iMEM iMEM iMEM iMEM iMEM • Roadmap (bfloat16, fp32) for training iMEM dMEM dMEM dMEM dMEM dMEM dMEM dMEM dMEM “Deep learning is a fundamentally new software model. So we need a new computer platform to run it...” Tile = (16 PE’s + 8 MACS) • Wide range of scalable solutions (2-1K tiles) • Future Proof all AI algorithms Tile Tile Tile Tile Tile • Flexible 2 dimensional tiling implementation AXI • Reconfigurable for dynamic networks Tile Tile Tile Tile Tile • Concurrent Network execution • Supports signal and vision processing WaveFlowTM = Wave Dataflow Array of Tiles Wave Computing © 2019 8 Future Proofing in an Rapidly Evolving Industry What is the likelihood that your DNN accelerator will run all these “yet to be invented” networks? “Deep learning is a fundamentally new software model. SoWave’s we need TritonAI a new computer™ 64 platform platform combines to run it...” a reconfigurable processor with an efficient neural network accelerator. Offers customers peace of mind and investment protection Wave Computing © 2019 9 Operations using WaveFlow™ Reconfigurable Processor Future-proof your Silicon CNN Layers Data Preprocessing • Sparse Matrix-Vector Processing • Scaling • Stochastic pooling • Aspect Ratio adjustment • Median pooling (illumination estimation & color correction) • Normalizing “Deep learning is a fundamentally new software model. So we need a new computer platform to run it...” Activation functions Other Functions • Leaky rectified linear unit (Leaky ReLU) (used in Yolo3) • Compression/Decompression • Parametric rectified linear unit (PReLU) • Encryption/Decryption • Randomized leaky rectified linear unit (RReLU) • Sorting Custom Operators (e.g.) • Novel Loss Function • New Softmax Implementation • Image resize nearest neighbor Wave Computing © 2019 10 MIPS-64 Processor MIPS-64: • MIPS64r6 ISA • 128-bit SIMD/FPU for int/SP/DP ops • Virtualization extensions • Superscalar 9-stage pipeline w/SMT • Caches (32KB-64KB), DSPRAM (0-64KB) • Advanced branch predict and MMU “Deep learning is a fundamentally new software model. So we need a new computer platform to run it...” Multi-Processor Cluster: • 1-6 cores • Integrated L2 cache (0-8MB, opt ECC) • Power mgmt. (F/V gating, per CPU) • Interrupt control with virtualization • 256b native AXI4 or ACE interface Wave Computing © 2019 11 Wave’s TritonAI™ 64 IP Software Platform Software Platform: • Mature IDE & Tools • Driver Layer for Technology Mapping • Linux Operating system support/updates • Abstract AI Framework calls via WaveRTTM API • Optimized AI Libraries for: • CPU/SIMD/WaveFlow/WaveTensor • TensorFlow-Lite Build support/updates • Extensible to Edge Training with Full TensorFlow Build “Deep learning is a fundamentally new software model. So TensorFlowwe need a Lite new I/F computer platformONNX to I/F run it...” Configurable Hardware Platform: WaveRT™ | API based Run time with Libraries • MIPS64r6 ISA Cluster Linux • 1- 6 cores Add-Ons to IDE: • 1-4 threads/core New/updated RT Libraries, Driver Layer • L1 I/D (32KB-64KB) New/updated Tech support • Unified L2 (256K to 8 Mbytes) MIPS IDE MIPS64 CPU+SIMD • WaveFlow Tile Array Compile, • 4 – N Tiles WaveFlow™ WaveTensor™ Build, Core Core MIPS64 CPU+SIMD Mem • WaveTensor Slice Array Debug, Coherent • 1 – N Slices Libraries MIPS64 CPU+SIMD Wave Computing © 2019 12 Federated Learning: The Next Frontier in Edge AI Wave Computing © 2019 13 Today’s Approach: Centralized Machine Learning Better ML comes at a cost of collecting data Most training done in the cloud. i.e. Send your data to the cloud. Diminished Privacy • Where is your data? “Deep learning is a fundamentally new software model.• WhoSo we has need access a new to computer your data? platform to run it...” Incompatible with Banks, Insurance, Military, Health sectors Latency Problems • Most access technologies are asymmetric High Communications Costs Wave Computing © 2019 14 Federated Learning: Edge AI with Data Privacy Federated learning uses training at the edge to refine the global model 1. Server selects a group of users Server Server model 2. Users receive copy of central model “Deep learning is a fundamentally new software model. So we need3. Users a new update computer model platform based to run on it...” local data (“Training at the Edge”) A The server selects K users B They receive the current model 4. Updates are
Details
-
File Typepdf
-
Upload Time-
-
Content LanguagesEnglish
-
Upload UserAnonymous/Not logged-in
-
File Pages56 Page
-
File Size-