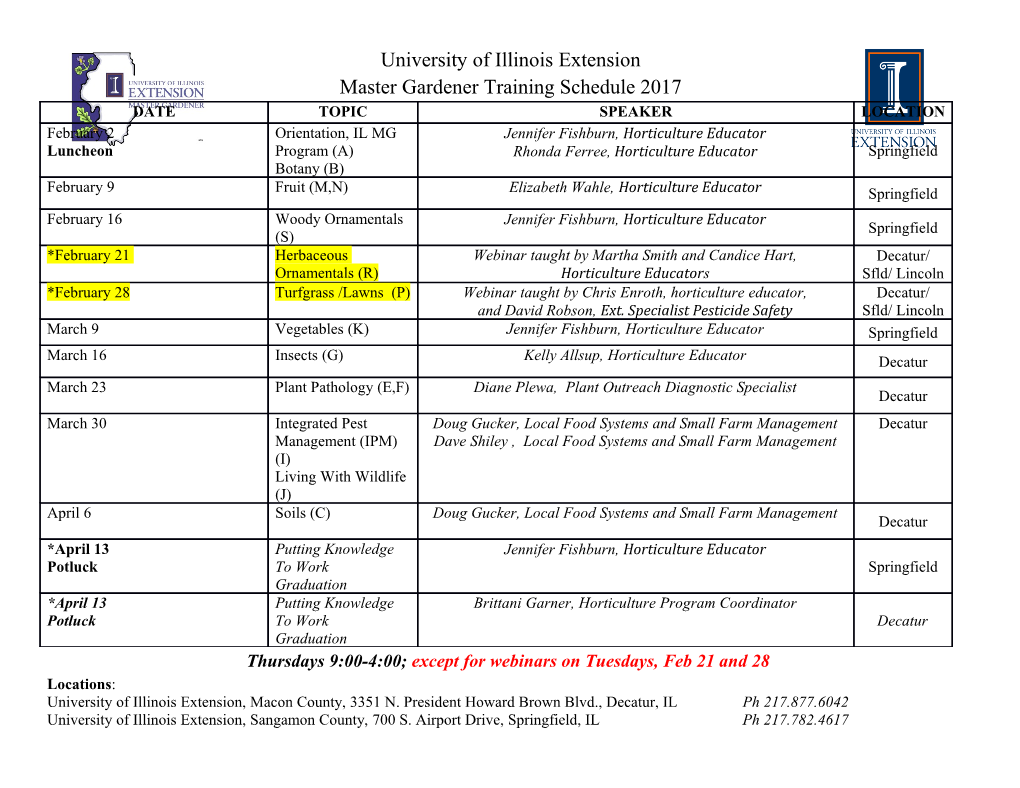
Journal of Traffic and Logistics Engineering Vol. 3, No. 2, December 2015 Clustering of Districts in Erzurum by Number of Injury Hümeyra Bolakar Department of Civil Engineering, Engineering Faculty, Aksaray University, Aksaray, Turkey E-mail: [email protected] Ahmet Tortum Department of Civil Engineering, Engineering Faculty, Ataturk University, Erzurum, Turkey E-mail: [email protected] Ahmet Atalay Narman Vocational High School, Ataturk University, Erzurum, Turkey E-mail: [email protected] Abstract—In this study, the number of injuries from road Clustering analysis is performed to determine the black traffic accidents for each district was identified in Erzurum, spots in traffic accident analysis in some studies [3], [6], Turkey during the years of 2012 and 2013. Clustering [8]. Moreover, clustering analysis is used to determine analysis was made according to these rates by using both similar districts or provinces in the literature [4]-[11]. classical k-means and fuzzy c-means technique. Districts In this study, clustering analysis was performed were divided into five clusters by analysis conducted with these two techniques. Districts with the highest injury risk according to the number of injuries from road traffic were determined, and the results obtained were compared. accidents (RTAs) occurred in Erzurum province for 2012 In this study, it was observed that the result of fuzzy c- and 2013. Clustering analysis was realized in two means technique is equal to the result of k-means technique. different forms. Firstly, traditional k-means method, and Moreover, it was determined that geographical information secondly fuzzy c-means method were applied. systems are advantageous to show and understand the Geographical Information System (GIS) software was results of the thematic maps. used to demonstrate the results of the clustering analysis. Thematic maps of the districts were drawn by using GIS Index Terms—clustering, k-means, fuzzy c-means, road software. Erzurum province has eighteen districts. traffic accident, injury The aim of this study is to group similar districts of Erzurum according to the number of injury in road traffic accidents. It is to compare the results of traditional I. INTRODUCTION clustering and fuzzy clustering methods. Traffic accidents and deaths, injuries and material damage caused by these accidents still occupy a large II. METHOD place as one of the most important problems in the world. In Turkey every year more than 500.000 traffic accidents A. Cluster Analysis happen and of these accidents 5.000 end up in death and Cluster Analysis is the group of methods that help to 160.000 results in injuries. In 2012 and 2013, 9861 traffic divide the units, variables, or units and variables that take accidents happened in Erzurum and 87 people died in place in the X data matrix and of which natural groupings these accidents while 5755 people were injured [1], [2]. are not certainly known in terms of sub-clusters similar to In recent years, clustering analysis was carried out by each other. researchers on traffic accidents [3]-[11]. The purpose of In clustering analysis, we used both traditional k- such cluster analyses was to determine the districts means and fuzzy c-means methods. showing similarities with each other in the light of data on traffic accidents. Upon determining similar districts, B. K-Means Clustering Method each group may be analysed separately and the measures K-means technique, the most commonly used of the to be taken for traffic accidents may be easily determined. non-hierarchy methods, was found by MacQueen and it Diminish in death and material loss will be achieved by aims to collect elements with values closest to each other means of special measures taken in each district group in in the same cluster in cases when the number of the addition to general precautions taken to prevent traffic clusters is known [12], [13]. accidents. In this method, individuals are divided into k clusters to make the sum of squares within the groups the smallest. According to the below stated formula individuals are Manuscript received February 1, 2015; revised April 12, 2015. ©2015 Journal of Traffic and Logistics Engineering 125 doi: 10.12720/jtle.3.2.125-128 Journal of Traffic and Logistics Engineering Vol. 3, No. 2, December 2015 classified into the cluster giving the smallest distance (the However the cluster centres should change at the same closest) when a1n, a2n, .... , akn every group is selected as time according to the following weighted average cluster centre for individuals in the same space while formula in (6): each observation vector of x1, x2, x3, ...., xn variables with n p variables expresses a point in the multi-dimensional x- m (üik ) xk space in (1) [9], [13]-[15]. v k1 (1 i c) (6) n i n 1 2 W min x a (1) (üik ) N n i in i1 k1 For the data to be partitioned to clusters by this method, C. Fuzzy c-Means Clustering Method following procedures must be completed step by step. Fuzzy c-means algorithm is the best-known and widely Step 1: Dates are a date series or pattern series X= {x1, used method of fuzzy partitioning clustering techniques. x2, x3,…, xn},in general, c is identified, (2<c<n-1), This algorithm was put forth by Dunn in 1973 and it was Step 2: Just any 1 repeat components of c mean vector, developed by Bezdec in 1981 [16]. Unlike in traditional n m clusters, each data point in fuzzy clusters may belong to ü()l x more than one sub-cluster in different degrees. However, ki k ()l k1 the sum of degrees of the membership of the same data v n m (7) point in different clusters coming one after the other ()l üki should be equal to 1. This means that if the degree of the k 1 membership of belonging of a data i to a cluster j is üi,j, (1ic ) then m being the number of clusters: m Step 3: Membership degree in step 1 according to the following expression, üi, j 1 (2) j1 (11) 1 üik (1 i c; 1 k n) On the other hand, the sum of membership degrees of 2 c m1 (8) the set of data in the same cluster j must be smaller than n, xk vi x v which is the number of data. In an extreme case, if all j1 k j data are in one cluster, then the sum of membership Step 4: In this step, it is controlled that calculations are degrees must be equal to n. This case is theoretical and it close to each other, it is either repeated or stopped [17]. is not meaningful in practice. However, the following may be written for all discussions, III. RESULTS AND DISCUSSION n (3) The aim of this study was to determine the cities üi, j n i1 similar to each other in terms of injury rates in traffic Solution is expected in extreme cases of (2) and (3) accidents happening in the districts. given the degrees of membership for real clustering. Here, In this study, clustering analysis was conducted using all data are common to each cluster with a certain degree the number of injury from RTAs in Erzurum for 2012 and of membership as mentioned above. For assigning the 2013. In this study, both k-means and fuzzy c-means points to different clusters, the idea of taking the gross clustering methods were used. The number of clusters average of the distance between points and given cluster was identified as five in both methods. centres will be used. The function to express this kind of Cluster centres obtained by classical k-means weight is defined as: clustering method were given in Table I. In the cluster analysis, researcher can entitle clusters n c 2 f (ü,v) (ü )m x v (4) [9], [12], [13], [15]. In this study, according to cluster ik k i centres, we entitled the clusters as the highest, more than k1 i1 medium, medium, less than medium and the least. The Here, 0<m<∞ exponent of membership degrees is title shows the number of injuries in a district. taken as weight. Vector v in (4) represents the coordinates of cluster centres. For clustering, this TABLE I. CENTRES OF CLUSTERS BY K-MEANS METHOD function must be minimized in the space of change. For degrees of membership, after this process of minimizing CLUSTER NUMBER which may be solved by taking derivatives according to unknown of which the mathematical details will not be 1 2 3 4 5 given here, the following is obtained in (5): Injuries during 2012 1002 104 8,14 16,86 53,50 1 üik 2 Injuries during 2013 991 117 4,86 27,71 43 c m1 xk vi (5) x v j1 k j According to cluster centres in k-means clustering (1 i c; 1 k n) method, clusters were entitled in Fig. 1. According to k- ©2015 Journal of Traffic and Logistics Engineering 126 Journal of Traffic and Logistics Engineering Vol. 3, No. 2, December 2015 means clustering, thematic map of districts was identified TABLE II. CENTRES OF CLUSTERS BY FUZZY C-MEANS in Fig. 1. CLUSTER NUMBER 1 2 3 4 5 Injuries 103,9394 1002 6,835842 16,4014 51,82501 during 2012 Injuries 116,9276 991 4,373043 26,87263 42,67219 during 2013 According to cluster centres in fuzzy c-means method, clusters were entitled in Fig. 2. According to the result of fuzzy c-means clustering, thematic map of districts was identified in Fig. 2. ArcGis program, the geographic information system software, was prepared and used to show the results in a visual way on the map of Erzurum (Fig.
Details
-
File Typepdf
-
Upload Time-
-
Content LanguagesEnglish
-
Upload UserAnonymous/Not logged-in
-
File Pages4 Page
-
File Size-