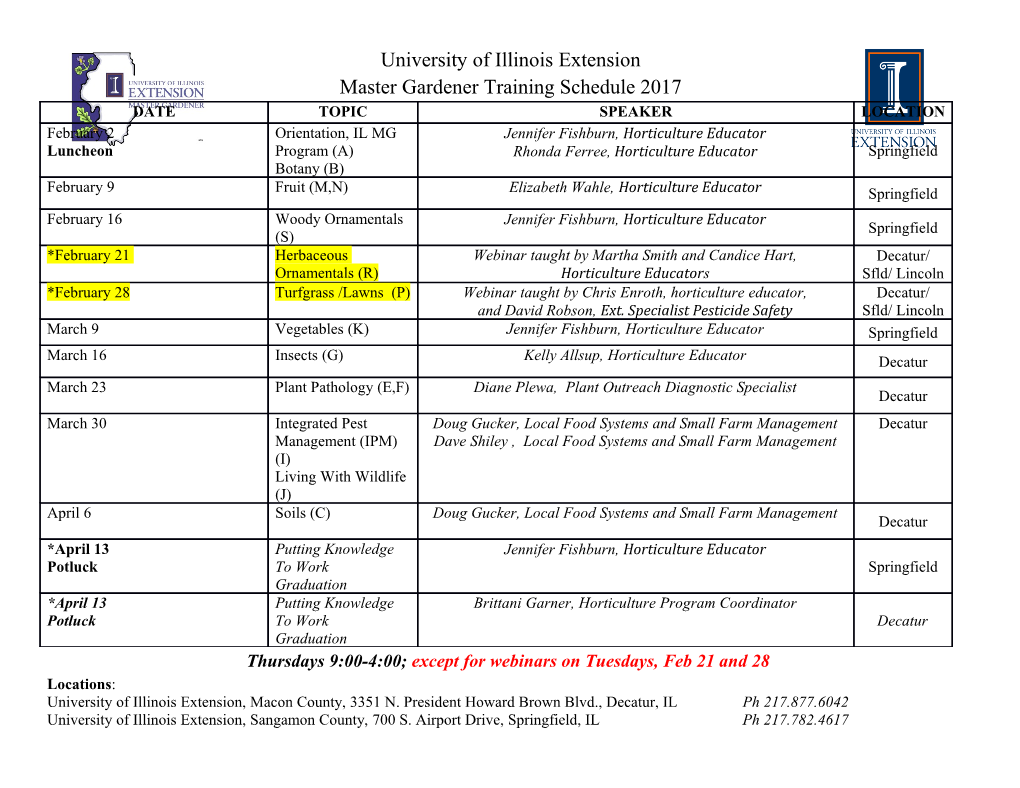
Evaluation of the Space Heating and Cooling Energy Savings of Smart Thermostats in a Hot-Humid Climate using Long-term Data FSEC-RR-647-16 August 2016 Authors Danny Parker, Karen Sutherland, and Dave Chasar Florida Solar Energy Center Presented at 2016 ACEEE Summer Study on Energy Efficiency in Buildings Copyright © 2016 American Council for an Energy Efficient Economy All rights reserved. No part of this work may be reproduced without consent of the Publisher: ACEEE 529 14th Street, N.W., Suite 600, Washington, D.C. 20045 phone: 202.507.4000 • fax: 202.429.2248 • e-mail: [email protected] • web: www.aceee.org Disclaimer The Florida Solar Energy Center/University of Central Florida nor any agency thereof, nor any of their employees, makes any warranty, express or implied, or assumes any legal liability or responsibility for the accuracy, completeness, or usefulness of any information, apparatus, product, or process disclosed, or represents that its use would not infringe privately owned rights. Reference herein to any specific commercial product, process, or service by trade name, trademark, manufacturer, or otherwise does not necessarily constitute or imply its endorsement, recommendation, or favoring by the Florida Solar Energy Center/University of Central Florida or any agency thereof. The views and opinions of authors expressed herein do not necessarily state or reflect those of the Florida Solar Energy Center/University of Central Florida or any agency thereof. Evaluation of the Space Heating and Cooling Energy Savings of Smart Thermostats in a Hot-Humid Climate using Long-term Data D. Parker, K. Sutherland, D. Chasar Florida Solar Energy Center ABSTRACT “Smart” thermostats regulate the home temperature by self-programming using heuristic evaluation of user habits and occupancy. Within the Phased Deep Retrofit (PDR) Project in Florida, a total of 26 NEST thermostats and two Lyric thermostats were installed in participating homes. Unlike previous evaluations, a full year of sub-metered hourly temperature and heating and cooling system operation data was available prior to the install of the smart thermostat allowing detailed evaluation of temperature-related changes. Overall measured heating and cooling energy savings averaged 9.5%. Unrealized Potential of Programmable Thermostats As thermostats are the central switch that control operation of heating and cooling systems—commonly the largest energy end use in homes—understanding how the occupants and thermostat interact is key to controlling energy use. However, this potential is complex, made up of the control hardware and how homeowners use it (behavior). That energy setup/setback has potential for energy savings has been demonstrated repeatedly in well- controlled measurements. For example, experimental work by Levins at Oak Ridge National Laboratory (Levins 1988) showed 20% measured heating savings from thermostat setback in the highly instrumented and unoccupied test homes. More recently, detailed National Research Council Canada test homes in Canada (Manning et al. 2007) showed that both thermostat setback (winter) and setup (summer) reliably produce savings of 13% and 11% respectively. Until the advent of smart thermostats, however, such savings levels have depended on the willingness of occupants to manage their thermostats and make effective control decisions. For example, Blasnik as cited by Bailes (2012) found heating savings of 5%-8% in multiple studies in many occupied homes in the northeastern United States from 1998–2008- less than half of identified potentials. Also, Roberts and Lay (2013) also showed that in 20 homes in New York, the measured interior nighttime temperatures were only about 3°F lower than midday temperatures and, in a similar sample of Florida homes for cooling, the often unoccupied daytime setup was only about 2°F. Thus, achieved temperature setback/ set-ups appear much lower than the potentials, given existing thermostat controls and associated behavior. From 1999–2001, a large monitoring project in central Florida for Florida Power Corporation evaluated 150 sub-metered homes and found that homes with programmable thermostats actually used more space cooling than those with manual slide thermostats because homeowners were more likely to change the daily settings on the manual thermostats due to the nuisance of programming (Nevius 2000). Verifying this finding, the influence of thermostats and load controls was evaluated in Florida homes by utilities desiring to enhance load control. These findings from utilities also showed that programmable thermostats led to increased cooling consumption (Lopes and Agnew 2010). The problems were not confined to Florida, as data from ©2016 ACEEE Summer Study on Energy Efficiency in Buildings 8-1 Minnesota showed much the same contradictory result from programmable thermostats (Nevius and Pigg 2000). Other efforts (Vastamaki, Sinkkonen, and Leinonen 2005) (Meier et al. 2011) indicated that much of the problem stems from an overly complex user interface for programmable thermostat, with only one in four households programming them. Figure 1. The Nest learning thermostat installed at one of the PDR sites showing portable logger recording temperature/humidity right by original thermostat & smart replacement. Smart Thermostats Newer “smart” thermostats get around these problems by self-programming depending on heuristic or machine learning evaluation of user control habits as well as sensed occupancy. Such smart thermostats include Nest (see Figure 1), Lyric, and Ecobee. These modern devices use a combination of data on occupancy, weather, and thermostat-setting preference to help consumers with automated setback/setup schedules. These devices have also been shown in other studies in other regions to produce cooling energy savings. For example, the Nest thermostats have been shown to provide savings of 1.16 kWh/day or 11.3% in a very large sample of homes in Southern California (Nest 2014). However, there are reasons to believe savings may differ in Florida, with different demographics, construction practices, and intense cooling consumption. Thus, this paper aims to evaluate the energy savings and peak demand impacts of smart thermostats in a highly metered field project in Florida. Installation Campaign The Phased Deep Retrofit project (PDR) in Florida installed a series of energy efficiency improvements in 56 homes over a three year period to estimate individual and combined technology impacts. Study sites for Nest or Lyric “smart” thermostat were chosen based on homeowner acceptance, compatibility, and the proviso that no confounding measures be installed in the home over the two year evaluation period Among the 38 smart thermostats installed in the PDR project, nine sites received Nests within the deep retrofits, 22 received a Nest or Lyric in 2014, and seven sites a Nest in 2015. Within the analysis, we carefully reduced confounding influences by removing sites from analysis with issues that would bias results. Three sites had the evaluation time periods limited due to a change out of the air conditioning equipment (very visible within the sub-metered data). 8-2 ©2016 ACEEE Summer Study on Energy Efficiency in Buildings The nine homes that had Nest thermostats installed in the summer of 2013 as part of the deep retrofits are also not included in this analysis because the thermostats were installed as a part of a much larger group of retrofit measures. This left 25 Nest sites and two Lyric sites for the final evaluation. A subset of three Nest installs installed in 2015 in homes with supplemental mini- split heat pumps operating and controlled independently were evaluated, but are not considered part of the main sample. Site characteristics for the installations are summarized in Table 1; HVAC characteristics are provided in Table 2. Table 1. Smart thermostat general site characteristics Yea Liv- House Year r ing No. Wall Air- of AC Site Bui Area of Ceiling Cons- tightness AHU/ Size AC # City lt (ft2) Occu. Stories Insulation truction (ACH50) Comp (tons) SEER 4 Melbourne 1971 1,166 2 1 R-19 CMU 11.5 2000 2.5 14.0 6 Palm Bay 1981 1,542 2 1 R-25 CMU 8.9 2006 3.0 13.0 1998/ 11 Cocoa Beach 1958 1,672 3 1 R-6 CMU 10.9 3.0 < 12 2002 12 Port Orange 1984 1,594 3 1 R-6 CMU 12.0 2000 3.0 < 12 16 Indialantic 1982 2,231 3 1 R38 Frame 12.7 2002 4.0 13.5 Melbourne 15 1975 1,359 2 1 R-15 CMU 8.2 1997 3.0 13.5 Beach 17 Indialantic 1964 1,456 2 1 R-30 CMU 8.4 2002 3.0 19.0 18 Cocoa 1995 1,802 2 1 R-21 CMU 6.2 2008 3.0 14.0 21 Cocoa Beach 1981 1,628 2 1 R-30 CMU 6.9 2013 3.5 13.0 22 Cocoa Beach 1955 1,743 2 1 R-19 CMU 11.0 2001 2.5 12.0 24 Cocoa 1986 1,978 3 2 R-25 Frame 9.5 2010 3.5 15.0 27 Palm Bay 1995 2,050 2 1 R-30 Frame 8.0 2008 5.0 12.0 28 Merritt Island 1966 2,622 2 1 R-16 CMU 8.9 1999 5.0 10.0 29 Cocoa 1985 1,215 2 1 R-30 Frame 10.2 1985 2.5 < 10 Pembroke 34 1978 1,651 2 1 R-8 CMU 9.3 2011 3.0 15.0 Pines CMU/ 1993/ 35 Plantation 1993 1,625 2 2 R-19 6.6 3.5 < 10 Frame 1998 42 Naples 2001 1,666 3 2 R-30 Frame 6.1 2002 3.0 10.0 45 Davie 1987 1,299 2 1 R-19 CMU 9.1 2006 2.5 13.0 1999/ 47 Fort Myers 1990 1,088 4 1 R-15 Frame 5.5 2.5 < 10 2004 48 Naples 1973 1,436 4 1 R-38 CMU 13.2 2006 3.0 13.0 50 Melbourne 1958 2,168 4 1 R-30 CMU 5.5 2005 4.0 14.0 52 Cocoa 2000 1,696 2 1 R-30 Frame 7.0 2012 3.0 13.0 56 Merritt Island 1963 1,000 3 1 R-19 CMU 13.5 2005 2.5 10.0 58 Rockledge 1979 2,020 2 1 R13 CMU 13.3 2003 3.5 13.0 Melbourne 59 1985 2,298 2 1 R-19 Frame 7.1 2005 4.0 14.0 Beach 43 Fort Myers 2000 1,383 2 1 R-25 CMU 6.5 1999 2.5 10.0 44 Naples 1998 1,627 2 1 R-19 CMU 4.7 1998 4.0 10.0 ©2016 ACEEE Summer Study on Energy Efficiency in Buildings 8-3 Table 2.
Details
-
File Typepdf
-
Upload Time-
-
Content LanguagesEnglish
-
Upload UserAnonymous/Not logged-in
-
File Pages17 Page
-
File Size-