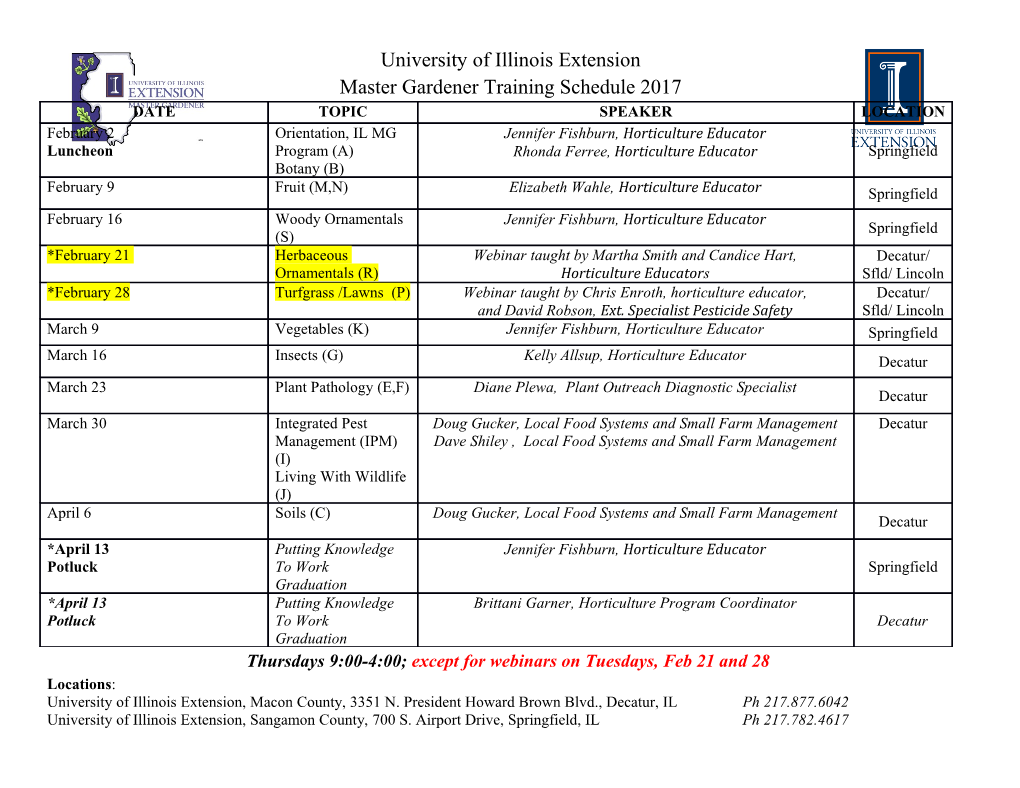
Iowa State University Capstones, Theses and Retrospective Theses and Dissertations Dissertations 1991 Edgeworth expansion and bootstrap approximation for M-estimators of linear regression parameters with increasing dimensions Muhammad Arif Tiro Iowa State University Follow this and additional works at: https://lib.dr.iastate.edu/rtd Part of the Statistics and Probability Commons Recommended Citation Tiro, Muhammad Arif, "Edgeworth expansion and bootstrap approximation for M-estimators of linear regression parameters with increasing dimensions " (1991). Retrospective Theses and Dissertations. 10077. https://lib.dr.iastate.edu/rtd/10077 This Dissertation is brought to you for free and open access by the Iowa State University Capstones, Theses and Dissertations at Iowa State University Digital Repository. It has been accepted for inclusion in Retrospective Theses and Dissertations by an authorized administrator of Iowa State University Digital Repository. For more information, please contact [email protected]. INFORMATION TO USERS This manuscript has been reproduced from the microfilm master. UMI films the text directly from the original or copy submitted. Thus, some thesis and dissertation copies are in typewriter face, while others may be from any type of computer printer. The quality of this reproduction is dependent upon the quality of the copy submitted. Broken or indistinct print, colored or poor quality illustrations and photographs, print bleedthrough, substandard margins, and improper alignment can adversely affect reproduction. In the unlikely event that the author did not send UMI a complete manuscript and there are missing pages, these will be noted. Also, if unauthorized copyright material had to be removed, a note will indicate the deletion. Oversize materials (e.g., maps, drawings, charts) are reproduced by sectioning the original, beginning at the upper left-hand corner and continuing from left to right in equal sections with small overlaps. Each original is also photographed in one exposure and is included in reduced form at the back of the book. Photographs included in the original manuscript have been reproduced xerographically in this copy. Higher quality 6" x 9" black and white photographic prints are available for any photographs or illustrations appearing in this copy for an additional charge. Contact UMI directly to order. University Microfilms International A Bell & Howell Information Company 300 North Zeeb Road, Ann Arbor, fvtl 48106-1346 USA 313/761-4700 800/521-0600 Order Number 9202398 Edgeworth expansion and bootstrap approximation for M-estimators of linear regression parameters with increasing dimensions Tiro, Muhammad Arif, Ph.D. Iowa State University, 1991 UMI 300N.ZeebRd. Ann Arbor, MI 48106 i Edgeworth expansion and bootstrap approximation for M-estimators of linear regression parameters with increasing dimensions by Muhammad Arif Tiro A Dissertation Submitted to the Graduate Faculty in Partial Fulfillment of the Requirements for the Degree of DOCTOR OF PHILOSOPHY Major: Statistics Approved: Members of the Committee: Signature was redacted for privacy. Signature was redacted for privacy. In Charge of Major Work Signature was redacted for privacy. Signature was redacted for privacy. For the Major Department Signature was redacted for privacy. For the Graduate College Iowa State University Ames, Iowa 1991 Copyright © Muhammad Arif Tiro, 1991. All rights reserved. ii TABLE OF CONTENTS ACKNOWLEDGEMENTS iv 1. INTRODUCTION 1 1.1 Preamble 1 1.2 Statements of the Problems 2 1.3 Basic Notations and Definitions 7 2. LITERATURE REVIEW 11 2.1 Edgeworth Expansion 11 2.2 Bootstrap Approximation ; 19 3. RESULTS ON LEAST SQUARES ESTIMATORS 26 3.1 Introduction 26 3.2 Edgeworth Expansion for Standardized Least Squares Estimators . 28 3.3 Edgeworth Expansion for Studentized Least Squares Estimators ... 29 3.4 Bootstrap Approximation for Studentized Least Squares Estimators . 33 3.5 Example 34 3.6 Proofs . 36 4. RESULTS ON GENERAL M-ESTIMATORS .59 4.1 Introduction .59 iii 4.2 Edgeworth Expansion for Standardized M-estimators 63 4.3 Edgeworth Expansion for Studentized M-estimators 64 4.4 Bootstrap Approximation for Studentized M-estimators 65 4.5 Example 68 4.6 Proofs 68 5. CONCLUDING REMARKS 82 BIBLIOGRAPHY 85 iv ACKNOWLEDGEMENTS I would like to express my deepest appreciation and sincere gratitude to my major professor Dr. S. N. Lahiri, for his guidance and advice during the course of my research, and to Dr. H. A. David, my major professor during my Master Program and co-major professor for my Ph.D. Program, for various ways he contributed to my education at Iowa State University. It has been a great pleasure having the opportunity to work with and learn from both scholars. Special thanks are due to Dr. V. A. Sposito, Dr. S. Sukhatme, Dr. E. H. Johnston and Dr. R. Hickman, as my committee members, for their valuable contributions to my education. I would like to thank the Indonesian government for providing me with a scholar­ ship through Indonesian Overseas Training Office - National Building Bureau, during the period of my study at Iowa State University. I am grateful to my mother, for the kind of love, encouragement, and the countless sacrifices she made for me and my family. She has been the foundation of my education, even though my father died before I was born. Finally, I am especially thankful to my wife, Bugiana, my daughters, Rina, Yaya, Ningsih, and Umairah for their patience, tolerance, love and never ending support of me during some difficult times we passed through. Thank you for helping me maintain my perspective and enthusiasm. 1 1. INTRODUCTION 1.1 Preamble The motivation of this study comes from an observation on the simplest kind of asymptotic theory, the one based on normal approximations. In this case we seem to be in paradise, pampered with maximally concentrated estimators, uniformly most powerful tests, and confidence sets which are intervals. There is but one disadvantage: in this paradise, one is rather far from reality. Normal approximation is an oversim­ plification. Moreover, the normal approximation does not allow one to discriminate between statistical procedures which are equivalent at this level of accuracy. Since the normal approximation reflects the actual performance of statistical procedures only to a certain degree, practical relevant differences may be hidden behind an identical asymptotic behavior. It appears that more refined asymptotic methods - using asymptotic expansions rather than normal approximations - lead to a general statistical theory which, though more complicated than the theory based on normal approximations, is still simple enough to achieve numerical results which are of sufficient accuracy for moderate sample sizes. We hope that asymptotic methods will finally be accepted as solutions to the problems mathematical statistics is faced with. The fact that, in most cases, no other solutions are feasible should support this process. 2 There are several general approaches to distribution approximations. The one to which we restrict attention is that of finding asymptotic expansions - in which the errors of approximation approach zero as some parameter, typically a sample size, approaches infinity. Essentially, the method consists of finding improvements to the large sample approximations used throughout statistics. The aim is not to obtain a better bound for the errors of the normal approximation, but to reduce the error by use of a better approximation. This preferable approach was developed by Edgeworth as an improvement to the central limit theorem. We will give special attention to this Edgeworth expansion. Furthermore, we also consider a resampling method called bootstrap. This method consists of approximating the distribution of a function of the observations and the underlying distribution, by what Efron (1979) calls the bootstrap distribution of this quantity. This distribution is obtained by replacing the unknown distribution by the empirical distribution of the data in the definition of the statistical function, and then resampling the data to obtain a Monte Carlo distribution for the resulting random variables. We study the two methods, Edgeworth expansion and bootstrap approximation, in the context of linear regression models. More details about the development of Edgeworth expansion and basic results establishing the specialty of bootstrap approximation will be given in the next chapter. 1.2 Statements of the Problems First of all, we consider a linear regression model Vj - x'-j3 + e^; ; = 1,2,... ,n (1.1) 3 where yi,y2i • • • iVn are observations; ej^,e2,...,£7^ are independent identically dis­ tributed random variables with common distribution function F, having mean zero, and finite variance cr^; xi^x2,- • • ,Xn are known, nonrandom p-vectors; and (3 is the pxl vector of parameters. The dimension p is allowed to increase with n. The problem is to find good approximations for the distributions of standardized as well as studentized linear combinations of least squares estimators of (3 without assuming normal errors. Also conditions on the Xj's should be investigated to get the good approximations. Note that the least squares estimates are optimal if the errors are independent and identically distributed normal, so we need a careful inspection of the pattern of the residuals. In fact, we never know the precise distribution of the errors; therefore we need robust alternatives to the method of least squares. A robust estimator is one that
Details
-
File Typepdf
-
Upload Time-
-
Content LanguagesEnglish
-
Upload UserAnonymous/Not logged-in
-
File Pages97 Page
-
File Size-