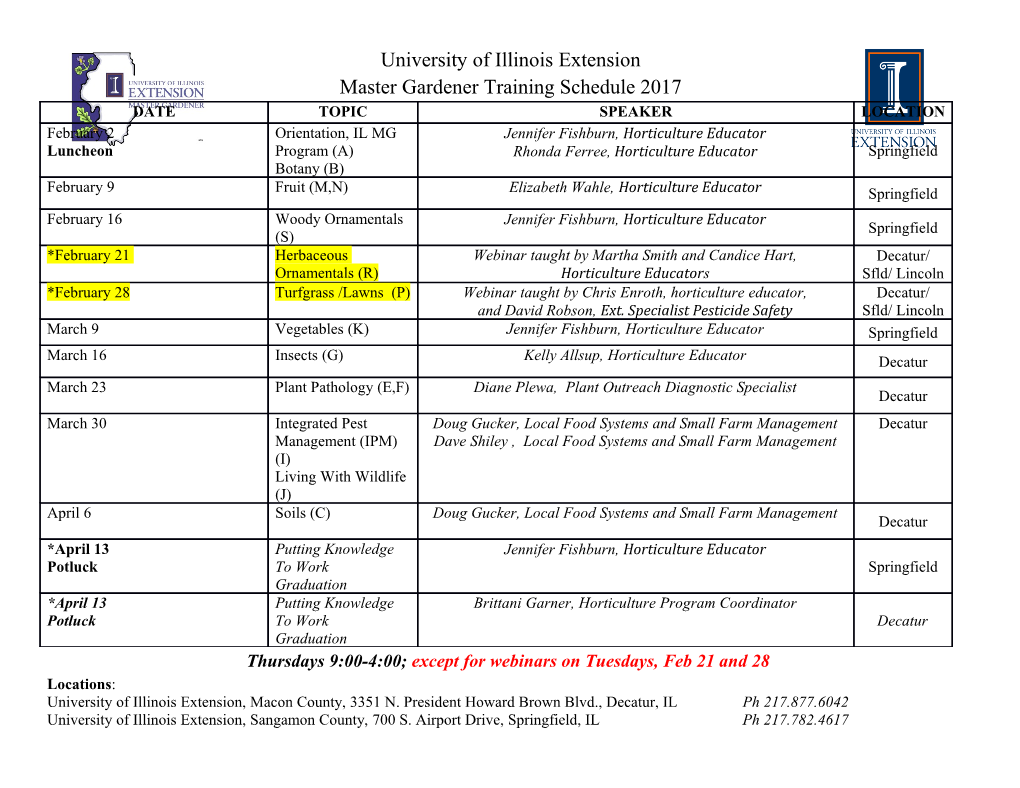
2009 10th International Conference on Document Analysis and Recognition Stochastic Model of Stroke Order Variation Yoshinori Katayama, Seiichi Uchida, and Hiroaki Sakoe Faculty of Information Science and Electrical Engineering, Kyushu University, 819-0395, Japan fyosinori,[email protected] Abstract “ ¡ ” under an unnatural stroke correspondence which max- imizes their similarity. Note that the correct stroke order of A stochastic model of stroke order variation is proposed “ ” is (“—” ! “j' ! “=” ! “n” ! “–”) and that of “ ¡ ” and applied to the stroke-order free on-line Kanji character is (“—” ! “–” ! “j' ! “=” ! “n” ). Thus if we allow any recognition. The proposed model is a hidden Markov model stroke order variation, those two characters become almost (HMM) with a special topology to represent all stroke order identical. variations. A sequence of state transitions from the initial One possible remedy to suppress the misrecognitions is state to the final state of the model represents one stroke to penalize unnatural i.e., rare stroke order on optimizing order and provides a probability of the stroke order. The the stroke correspondence. In fact, there are popular stroke distribution of the stroke order probability can be trained orders (including the standard stroke order) and there are automatically by using an EM algorithm from a training rare stroke orders. If we penalize the situation that “ ¡ ” is set of on-line character patterns. Experimental results on matched to an input pattern with its very rare stroke order large-scale test patterns showed that the proposed model of (“—” ! “j' ! “=” ! “n” ! “–”), we can avoid the could represent actual stroke order variations appropriately misrecognition of “ ” as “ ¡ .” and improve recognition accuracy by penalizing incorrect For this purpose, a stochastic model of stroke order vari- stroke orders. ations is proposed. The model is a hidden Markov model (HMM) with a special topology that represents all stroke 1. Introduction order variations. A sequence of state transitions from the initial state to the final state of the model represents one In on-line recognition of multi-stroke characters, such as stroke order. If a reasonable probability is assigned to each Japanese Kanji characters and Chinese characters, stroke state transition by a training scheme, it is possible to repre- order variation is one of the most serious hurdles. An in- sent the probability distribution of stroke order variations. put pattern written in a certain stroke order has a partial or This model is useful for online character recognition be- global difference from its standard pattern written in a dif- cause it can suppress rare stroke orders by a low probability. ferent stroke order 1 and therefore some special treatment In fact, as shown in this paper, the proposed model could is necessary to compensate the variation. improve recognition accuracy of Japanese Kanji characters, which were collected from the large Japanese Kanji charac- Several approaches have been proposed to deal with ter database called “HANDS-kuchibue d-97-06-10 [2].” stroke order variations [1]. One important approach is to optimize the stroke-to-stroke correspondence between input 2. Conventional stroke-order free methods and standard patterns. If the correct stroke correspondence is determined, the similarity under the correspondence will 2.1. Related works be constant regardless of the stroke order variation of an in- For stroke order-free on-line character recognition, there put pattern. are mainly three approaches to deal with stroke order vari- This approach, however, has a side effect; the similar- ations. The first approach is to prepare multiple standard ity between an input pattern and the standard pattern of patterns with popular stroke orders [3]. Although this ap- a “wrong” category is often over-estimated by unnatural proach is very simple, there is no well-established strategy stroke correspondence. For example, an input pattern “ ” of choosing “popular” stroke orders. In addition, it cannot written in the correct stroke order will be misrecognized as cope with unexpected stroke order variations at all. 1Readers who are not familiar with multi-stroke characters should pay The second approach [4, 5] converts on-line character attention to the interesting fact that each multi-stroke character has its own patterns into image patterns and recognizes them by off- “correct” (or standard) stroke order. Children learn the correct stroke order line character recognition techniques. Although this hybrid in their school age. Every standard pattern is often prepared in its correct approach is also promising and provides stroke order-free stroke order. On the other hand, an incorrect but major stroke order ex- ists as the local rule, and the rare stroke order is taken accidentally and recognition systems, it cannot fully utilize the dynamics, or intentionally, especially by beginners of learning multi-stroke characters. temporal information, in on-line character patterns. 978-0-7695-3725-2/09 $25.00 © 2009 IEEE 803 DOI 10.1109/ICDAR.2009.146 m p n S(2) m,n Pre(m ) Post(m ) 0011 m l 2 0011 3 n 0001 0111 0001 0111 0101 0101 1 4 0010 1011 0010 1011 0 0110 0110 0000 1111 0000 1111 1001 0100 1001 1101 0100 1101 1010 1010 1000 1110 1000 1110 1100 1100 k=0 1 2 3 4 k=0 1 2 3 4 Figure 2. Sets of states, S(k), Pre(m), and Figure 1. Cube graph representing all stroke Post(m). order variations of “ ¢ . ” timal path problem of the cube graph. The optimal path is The third and more essential approach is to optimize defined as the path with the maximum sum of stroke simi- stroke correspondence between input and standard pat- larities. Dynamic programming (i.e., Viterbi algorithm) was terns [6, 7, 8, 9, 10]. Stroke correspondence between input used to find the optimal path in [6]. and standard patterns is optimized so as to maximize to- tal stroke similarities. Cube search [6] introduced below is 3. Cube HMM also a method of this approach. In principle, this approach is robust enough to cope with any stroke order. This ro- In this section, a stochastic model of stroke order varia- bustness, however, often over-estimates similarity between tions, called cube HMM, is newly introduced. Cube HMM patterns of different categories and leads misrecognitions. is a left-to-right (and no self-state transition) HMM whose In order to suppress those misrecognitions, we newly intro- topology is defined as the cube graph. In cube HMM, each duce a stochastic model of stroke order variations, which is node of the cube graph is treated as a state. The stroke c a pioneering attempt to the best of authors' knowledge. similarity qm;n is treated as an “symbol” output probabil- ity, where the symbol is a stroke. For example, qc 2.2. Cube search [6] 0001;0101 is the output probability of I2 and designed to become large c Cube search is a method to optimize stroke order corre- (small) if the stroke I2 is similar (dissimilar) to J3 . The c spondence. Its key idea is to represent all stroke order vari- probability qm;n will be detailed in 3.1. ations of a K-stroke character as a K-dimensional hyper The main difference between the cube search of 2.2 c cubic graph. The “cube” name derives from this structure. and cube HMM is that a state transition probability pm;n c c c c Figure 1 shows the cube graph G for the category c =“ ( n pm;n = 1) is assigned to em;n, along with qm;n. The P c ¢ ”, which is a four-stroke Kanji character. All stroke order state transition probability pm;n represents the probability c variations are represented by paths from the start node to the of a specific stroke order. For example, p0001;0101 repre- end node. The four-digit binary number in each node indi- sents the probability that the third standard stroke is written cates the strokes matched already. Let Ik be the kth stroke as the second stroke in the category c. Thus, if we train c pc appropriately, cube HMM can represent the probabil- of the input pattern and Jl be the lth stroke of the standard m;n pattern of the category c. Then, “0101” means that I1 and ity of stroke order variations of c. c c c c The pc for the K-stroke character spends memory of I2 have already been matched to J1 and J3 , or J3 and J1 . m;n c c K−1 Each edge em;n on the cube graph G represents a spe- O(K · 2 ), it is hard to describe the large number of c cific stroke correspondence. For example, the edge em;n stroke character in a cube HMM. However such character between m = 0001 and n = 0101 represents the corre- consists of character components and radicals, it is useful c spondence between I2 and J3 . (This is because the third to describe the character as cube HMMs describing radical. bit becomes 1 at the second transition.) A stroke similarity c c c 3.1. Training algorithm qm;n between I2 and J3 is assigned to the edge em;n. In [6], the stroke similarity is evaluated by stroke shape and Like popular HMMs, the state transition probability position, just like other methods [7, 8, 9, 10]. Then, the pm;n of cube HMM can be trained by the EM algorithm. optimal stroke correspondence problem between the input For the notational simplicity, we will drop the superscript c, pattern and the standard pattern of c is considered as the op- whenever there is no confusion. 804 Table 1. Statistics of dataset. The parenthe- ◦ Forward probability sized number shows the number of samples α0 = 1:0; with regular stroke order.
Details
-
File Typepdf
-
Upload Time-
-
Content LanguagesEnglish
-
Upload UserAnonymous/Not logged-in
-
File Pages5 Page
-
File Size-