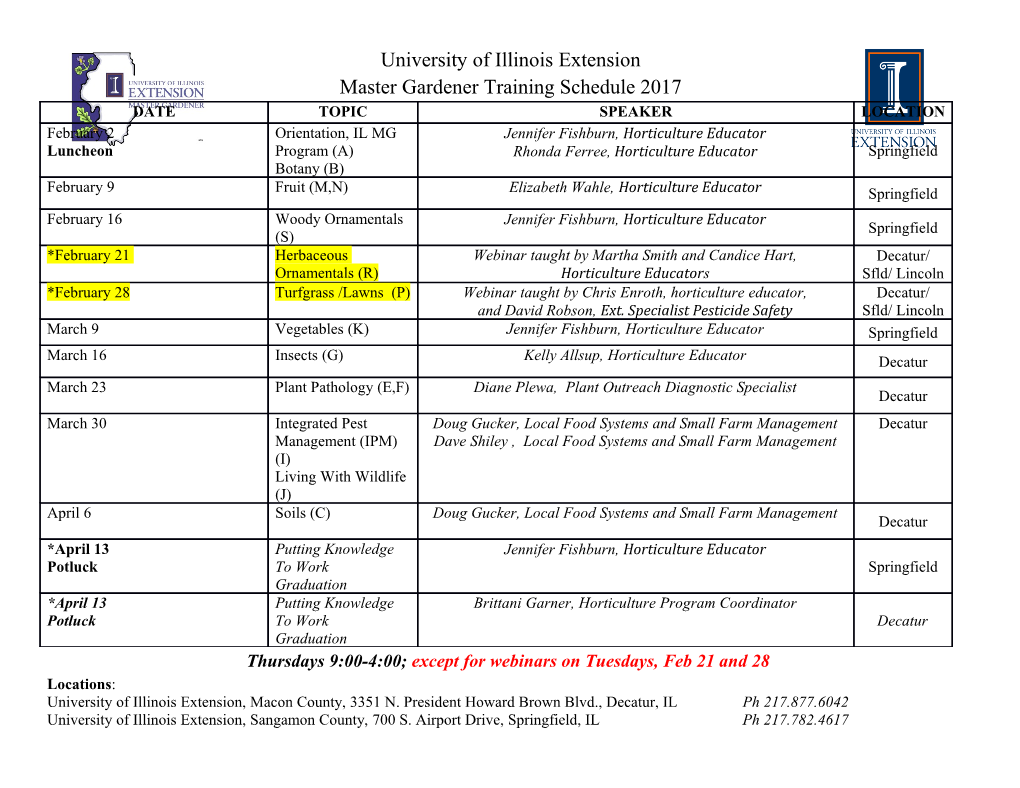
Adversarial Atacks on Graph Neural Networks via Node Injections: A Hierarchical Reinforcement Learning Approach Yiwei Sun Suhang Wang∗ Xianfeng Tang The Pennsylvania State University The Pennsylvania State University The Pennsylvania State University University Park, PA, USA University Park, PA, USA University Park, PA, USA [email protected] [email protected] [email protected] Tsung-Yu Hsieh Vasant Honavar The Pennsylvania State University The Pennsylvania State University University Park, PA, USA University Park, PA, USA [email protected] [email protected] ABSTRACT ACM Reference Format: Graph Neural Networks (GNN) ofer the powerful approach to node Yiwei Sun, Suhang Wang, Xianfeng Tang, Tsung-Yu Hsieh, and Vasant classifcation in complex networks across many domains including Honavar. 2020. Adversarial Attacks on Graph Neural Networks via Node Injections: A Hierarchical Reinforcement Learning Approach. In Proceedings social media, E-commerce, and FinTech. However, recent studies of The Web Conference 2020 (WWW ’20), April 20–24, 2020, Taipei, Taiwan. show that GNNs are vulnerable to attacks aimed at adversely im- ACM, New York, NY, USA, 11 pages. https://doi.org/10.1145/3366423.3380149 pacting their node classifcation performance. Existing studies of adversarial attacks on GNN focus primarily on manipulating the 1 INTRODUCTION connectivity between existing nodes, a task that requires greater Graphs, where nodes and their attributes denote real-world entities efort on the part of the attacker in real-world applications. In con- (e.g., individuals) and links encode relationships (e.g., friendship) trast, it is much more expedient on the part of the attacker to inject between entities, are ubiquitous in many application domains, in- adversarial nodes, e.g., fake profles with forged links, into existing cluding social media [1, 21, 35, 49, 50], e-commerce[16, 47], and graphs so as to reduce the performance of the GNN in classifying FinTech [24, 33]. Many real-wold applications are involve classify- existing nodes. ing the nodes in graph data based on the attributes of the nodes, and Hence, we consider a novel form of node injection poisoning their connectivity and attributes of the nodes that are connected to attacks on graph data. We model the key steps of a node injec- them in the graph. Thus, revealing a user’s level of risk in fnancial tion attack, e.g., establishing links between the injected adversarial platform such as AliPay1 can be formulated as a node classifcation nodes and other nodes, choosing the label of an injected node, etc. problem [24, 41]. Graph Neural Networks (GNNs) [13, 23, 26], cur- by a Markov Decision Process. We propose a novel reinforcement rently ofer the state-of-the art approach to node classifcation in learning method for Node Injection Poisoning Attacks (NIPA), to graph-structured data. sequentially modify the labels and links of the injected nodes, with- However, recent studies [12, 38, 44, 51] show that GNNs are vul- out changing the connectivity between existing nodes. Specifcally, nerable to poisoning attacks which add perturbation to the training we introduce a hierarchical Q-learning network to manipulate the graph. Since GNNs are trained based on node attributes and the link labels of the adversarial nodes and their links with other nodes in structure in the graph, an adversary can attack the GNNs by poi- the graph, and design an appropriate reward function to guide the soning the graph data used for training. For example, Nettack [51] reinforcement learning agent to reduce the node classifcation per- shows that by adding the adversarial perturbations on the node’s formance of GNN. The results of the experiments show that NIPA is attributes and the graph structure, classifcation accuracy of graph consistently more efective than the baseline node injection attack convolution network signifcantly drops. However, the success of methods for poisoning graph data on three benchmark datasets. such attack strategy requires that the adversary is able to control these nodes and manipulate its connectivity. In other words, poi- KEYWORDS soning the real-world graphs such as Facebook and twitter require Adversarial Attack; Graph Poisoning; Reinforcement learning; breaching the security of the database that stores the graph data, or manipulating the requisite members into adding or deleting their ∗ links to other selected members. Consequently, such attack strategy Corresponding Author is expensive and usually requires more budgets for the adversary to execute without being caught. This paper is published under the Creative Commons Attribution 4.0 International Thus, we need a more efcient way to poison the graphs to in- (CC-BY 4.0) license. Authors reserve their rights to disseminate the work on their crease the node misclassifcation rate of GNNs without changing the personal and corporate Web sites with the appropriate attribution. link structure between the existing nodes in the graph. Injecting fake WWW ’20, April 20–24, 2020, Taipei, Taiwan © 2020 IW3C2 (International World Wide Web Conference Committee), published nodes (users) to social networks with carefully crafted node labels under Creative Commons CC-BY 4.0 License. and connecting them to carefully chosen existing nodes ofers a ACM ISBN 978-1-4503-7023-3/20/04. https://doi.org/10.1145/3366423.3380149 1https://intl.alipay.com/ 673 WWW ’20, April 20–24, 2020, Taipei, Taiwan Yiwei Sun, Suhang Wang, Xianfeng Tang, Tsung-Yu Hsieh, and Vasant Honavar promising approach to accomplishing this objective. For example, !$# !"# !%# in the fnancial platform, there is signifcant fnancial incentives for adversaries to attack the GNNs and manipulate the risks level of the real users. However, it is impossible for an attacker to breach the database. In contrast, an attacker could easily sign up fake ac- counts, create the social identity of the profles and send friendship requests to the real members. And as the social users always want to have the social infuence[9, 31], they tend to accept the friendship %/,$0 1-$23 &'(() $**$%+,- .($-* $**$%+,- requests from the others. With some of the real users accept the friendship from the attacker, the fake accounts are connected to the real users and thus such social network is poisoned. Once the Figure 1: (a) is the toy graph where the color of a node rep- GNNs are trained on the corrupted graph, the propagation of the resents its label; (b) shows the node injection poisoning at- fake information will misclassify the predicted level of risks on real tack performed by a naive attacker; (c) shows the node injec- users. Such node injection poisoning attacks are easier and less tion poisoning attack performed by a smart attacker using expensive to execute compared to those that require manipulating a smart strategy. The injected nodes are circled with dashed the links between existing nodes in the graph. Though promising, line. the work on such attacks are limited. Therefore, in this paper, we investigate a novel problem of graph The rest of the paper is organized as follows: Section 2 reviews poisoning attack by node injection. In essence, we are faced with the related work on adversarial attacks and reinforcement learning two challenges: (i) How to mathematically model and efectively on graph data; Section 3 formally defnes the non-target-specifc establish links between an injected adversarial (fake) node to ex- node injection poisoning attack problem. Section 4 presents NIPA, isting nodes in the original graph or to other injected adversarial our proposed solution; Section 5 describes our experimental results; nodes. As shown in Figure 1, both the attackers in (b) and (c) want section 6 concludes with a summary and an outline of promising to inject two fake nodes into the clean graph in (a). Obviously, directions for future work. the "smart attacker" who carefully designs the links and labels of the dashed line injected nodes could better poison the clean graph 2 RELATED WORK than the "dummy attack" who establish the links and generate the labels at random; and (ii) How to efciently solve the optimiza- Our study falls in the general area of data poisoning attacks on tion problem as the graph is discrete and highly-nonlinear. In an machine learning [4], that aim to corrupt the data so as to adversely attempt to solve these two challenges, we propose a novel frame- impact the performance of the predictive model that is trained on work named NIPA, to perform the Node Injection Poisoning Attack. the data. Such attacks have been extensively studied in the case As sequentially adding the adversarial connections and designing of non graph-structured data in supervised [3, 29] and reinforce- adversarial labels of the injected fake nodes could be naturally ment [18, 22, 25] learning. Specifcally, recent work has shown that formulated as the Markov decision process (MDP), NIPA adopts Q- deep neural networks are particularly vulnerable to data poison- learning algorithms, which have shown great successes for solving ing attacks [8, 19, 20, 37]. However, little attention has been given such problems [7, 36, 43]. The adopt of Q-learning also naturally to understanding how to poisoning the graph structured data. In solves the challenge of discrete optimization as now we concert the this paper, our focus is on such attacks on classifers trained on discrete edge adding process as actions in reinforcement learning graph-structured data. framework. To reduce the searching space, NIPA adopt a hierarchi- cal Q-learning network to decompose the actions. To cope with the 2.1 Adversarial Attacks on GNN graph highly-nonlinearity, NIPA comprises of deep Q network and The previous works [19, 37] have shown the intriguing properties GNN based state representation method. These components could of neural networks as they are "vulnerable to adversarial examples" learn the semantic structure of the graph and convert the discrete in computer vision domain. For example, in [19], the authors show graph structure to latent representations.
Details
-
File Typepdf
-
Upload Time-
-
Content LanguagesEnglish
-
Upload UserAnonymous/Not logged-in
-
File Pages11 Page
-
File Size-