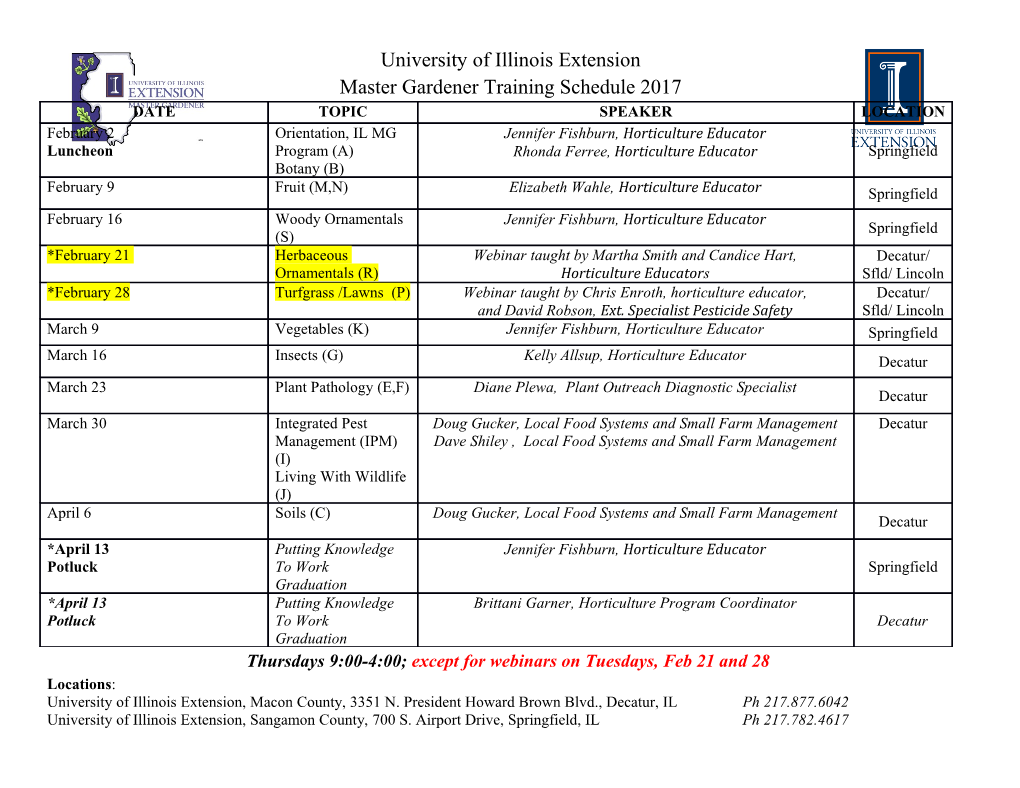
Estimation of Regional Business Cycle in Japan using Bayesian Panel Spatial Autoregressive Probit Model∗ Kazuhiko Kakamuy Hajime Wagoz Hisashi Tanizakix Abstract This paper considers the panel spatial autoregressive probit model from Bayesian point of view. The probit model is useful for qualitative (specifically, binary) data analyses. As it is well known, it is not easy to estimate the panel probit model by the maximum likelihood method. Therefore, in this paper, we consider the Markov chain Monte Carlo (MCMC) method to estimate the parameters as an alternative estima- tion method. Moreover, including the spatial autoregressive effect into the panel probit model we consider estimating the panel spatial autore- gressive probit model. Our model (i.e., the panel spatial autoregressive probit model) is illustrated with simulated data set and it is compared with the panel probit model. Furthermore, taking into account the ∗An earlier version of this paper was presented at the 2005 Japanese Joint Statistical Meeting at Hiroshima, the 2005 Fall meeting of Japanese Economic Association at Chuo University, MODSIM05 in Melbourne and ISBA08 in Hamilton Island. We would like to thank seminar/conference participants, especially Hideo Kozumi, Koji Miyawaki, Yasuhiro Omori, Mike K.P. So and Cathy W.S. Chen for their helpful comments and suggestions. However, responsibility for any errors remains entirely with us. This research was partially supported by the Ministry of Education, Science, Sports and Culture (Grant-in-Aid for Young Scientists (B) #20730144) for K. Kakamu and Japan Society for the Promotion of Science (Grant-in-Aid for Scientific Research (C) #18530158) for H. Tanizaki. yFaculty of Law and Economics, Chiba University, Inageku, Chiba 263-8522, Japan. Email: [email protected]. zDepartment of Economics, Kyoto Sangyo University, Kitaku, Kyoto 603-8555, Japan. Email: [email protected] xGraduate School of Economics, Kobe University, Nadaku, Kobe 657-8501, Japan. Email: [email protected] 1 spatial interaction, we explore the regional business cycle across 47 pre- fecture data in Japan, where the estimation period is from 1979 to 2003. Using Japanese regional data, we conclude from the empirical results that the spatial interaction plays an important role in the regional busi- ness cycle. Key Words: Business cycle; Markov chain Monte Carlo (MCMC); Panel spatial autoregressive probit model. JEL Classification: C11, C15, C23, R11. 1 Introduction The probit model has been widely used in qualitative data regression, espe- cially in microeconometrics or business cycle analysis, and it has been extended to the panel analysis. Maddala (1987) gives excellent surveys of the panel pro- bit model. Although the probit model is useful for qualitative data analysis, it is difficult to estimate the panel probit model by the maximum likelihood method, as pointed out by Bertschek and Lechner (1998). Therefore, alterna- tive estimation methods such as the generalized method of moments (GMM) are proposed (see Bertschek and Lechner, 1998; Greene, 2004 and so on) for estimation of the panel probit model. Since the seminal work by Anselin (1988), the spatial dependency becomes the concern of economic activity. Therefore, the probit model with the spatial dependency (i.e., the spatial autoregressive probit model) has been examined both analytically and empirically. For example, Holloway et al. (2002) ap- ply the spatial probit model to high-yielding variety (HYV) adoption among Bangladeshi rice producers. Smith and LeSage (2004) also propose the spatial dependency with individuals in each location, and apply to the 1996 presi- dential election results for 3110 US counties. As it is difficult to estimate the spatial autoregressive probit model by the maximum likelihood method, all the past studies are estimated by the Markov chain Monte Carlo (MCMC) method (e.g., LeSage, 2000). In the business cycle analysis, it is important to capture the turning point. Several models are proposed, i.e., probit and logit model (Maddala, 1992); sequential probability recursion model (Neftci, 1982); Markov switching model (Hamilton, 1989); dynamic Markov-switching factor model (Watanabe, 2003). However, they do not take into account the regional business cycle with the 2 spatial dependency. In this paper, incorporating the spatial dependency into the panel probit model, we consider the panel spatial autoregressive probit model as a regional business cycle model. From Bayesian point of view, Kakamu and Wago (2008) examine the panel spatial autoregressive model. This paper considers the panel spatial autore- gressive probit model in Bayesian framework, by extending LeSage (2000) and Kakamu and Wago (2008). Our model (i.e., the panel spatial autoregressive probit model) is illustrated with simulated data set and it is compared with the panel probit model, where the spatial autoregressive effect is ignored. We find from the simulation results that the bias appears in the parameter esti- mates when the estimated model is the panel probit model and the true model is the panel spatial autoregressive model. In addition, the bias increases as the spatial autoregressive effect is large, which implies that the spatial interaction plays an important role. Furthermore, taking into account the spatial interaction, we also explore the regional business cycle in Japan, where 47 prefecture data during the periods from 1979 to 2003 are utilized. From the empirical results, (i) we confirm that the occurrence and collapse of the bubble economy is a common phenomena in Japan, i.e., the probability that the growth rate of regional IIP (Index of Industrial Production) is positive falls drastically in 1992-1993 and rises after 1993 (note that the bubble economy in Japan burst in 1991), (ii) the business cycle in Japan is very similar to the regional business cycle in each prefecture, and (iii) the posterior distributions of the spatial interaction do not include zero in the 95% credible intervals, when the business cycle phase (expansion or contraction) in most of the prefectures is similar to each other (i.e., 1979, 1984, 1988-1993, 1998 and 2001). Thus, we conclude from Japanese regional data that the spatial interaction plays an important role in the business cycle. The rest of this paper is organized as follows. In the next section, we summarize the panel spatial autoregressive probit model. Section 3 discusses computational strategy of the MCMC methods. In Section 4 our approach is illustrated with simulated data set. Section 5 presents the empirical results based on the business index records in Japan, where 47 prefectures data from 1979 to 2003 are examined. Section 6 summarizes the results with concluding remarks. 3 2 Panel Spatial Autoregressive Probit Model Let yit be a binary data and xit be a 1 × k vector of exogenous variables, where i indicates the region (i = 1; 2; ··· ; n) and t represents time period (t = 1; 2; ··· ;T ). Let wij denote the spatial weight of the jth region in the ith region, which is given by: (i) wij = 0 for all i = j and (ii) wij = 1=mi when the jth region is contiguous with the ith region and wij = 0 otherwise, where mi denotes the number of regions which is contiguous with the ith region. Pn Note that we have j=1 wij = 1 for all i. In the panel model, we consider that the unobservable component which is specific to the ith region affects the dependent variable (e.g., Kakamu and Wago, 2008). αi is denoted by the unobservable component. Then, the panel spatial autoregressive probit model with parameters ρt, αi and β is written as follows: ( 1; if zit ≥ 0; yit = 0; if zit < 0; Xn zit = ρt wijzjt + αi + xitβ + it; it ∼ N (0; 1); (1) j=1 where zit is a latent variable (see Tanner and Wang, 1987). In (1), ρt represents the spatial interaction at time t. We may assume ρt = ρ. In the model above, however, it might be more plausible to consider that the spatial interaction varies over time. Let us define: 0 0 ρ = (ρ1; ρ2; ··· ; ρT ) ; α = (α1; α2; ··· ; αn) ; 0 0 0 0 0 zt = (z1t; z2t; ··· ; znt) ; z = (z1; z2; ··· ; zT ) ; 0 0 0 0 0 yt = (y1t; y2t; ··· ; ynt) ; y = (y1; y2; ··· ; yT ) ; 0 0 0 0 0 0 0 0 Xt = (x1t; x2t; ··· ; xnt) ; X = (X1; X2; ··· ; XT ) : W is called the spatial weight matrix (see, e.g., Anselin, 1988), where the element of W in row i and column j is denoted by wij, defined above. Then, the likelihood function of the model (1) is given by: YT L(yjρ; α; β; z; X; W ) = f(ytjρt; α; β; zt; Xt; W ); (2) t=1 where ! 0 − n etet f(y jρ ; α; β; z ; X ; W ) = (2π) 2 jI − ρ W j exp − t t t t n t 2 4 Yn YT n o × yit1[0;1)(zit) + (1 − yit)1(−∞;0](zit) : i=1 t=1 In indicates the n × n unit matrix, et is given by et = zt − ρtW zt − Xtβ − α, and 1(a;b)(x) denotes the indicator function, which takes 1 when x lies on the interval between a and b. 3 Posterior Analysis 3.1 Joint Posterior Distribution Since we utilize Bayesian method for estimation, we adopt the following hier- archical prior: ( )( ) YT Yn 2 2 2 π(ρ; α; β; µ, ξ ) = π(ρt) π(αijµ, ξ ) π(β)π(µ)π(ξ ); t=1 i=1 which is used in Kakamu and Wago (2008), where µ and ξ2 indicate the mean and variance of αi, respectively. Given the prior density π(ρ; α; β; µ, ξ2) and the likelihood function (2), the joint posterior distribution can be expressed as: π(ρ; α; β; µ, ξ2jz; y; X; W ) YT 2 / π(ρ; α; β; µ, ξ ) f(ytjρt; α; β; zt; Xt; W ): (3) t=1 2 2 We assume the prior distributions, i.e., π(ρt), π(αijµ, ξ ), π(β), π(µ) and π(ξ ), as follows: 2 2 ρt ∼ U(−1; 1); αijµ, ξ ∼ N (µ, ξ ); β ∼ N (β0; Σ0); ν λ µ ∼ N (µ ; ξ2); ξ2 ∼ IG( 0 ; 0 ); 0 0 2 2 where IG(a; b) denotes the inverse gamma distribution with scale parameter a and shape parameter b.
Details
-
File Typepdf
-
Upload Time-
-
Content LanguagesEnglish
-
Upload UserAnonymous/Not logged-in
-
File Pages22 Page
-
File Size-