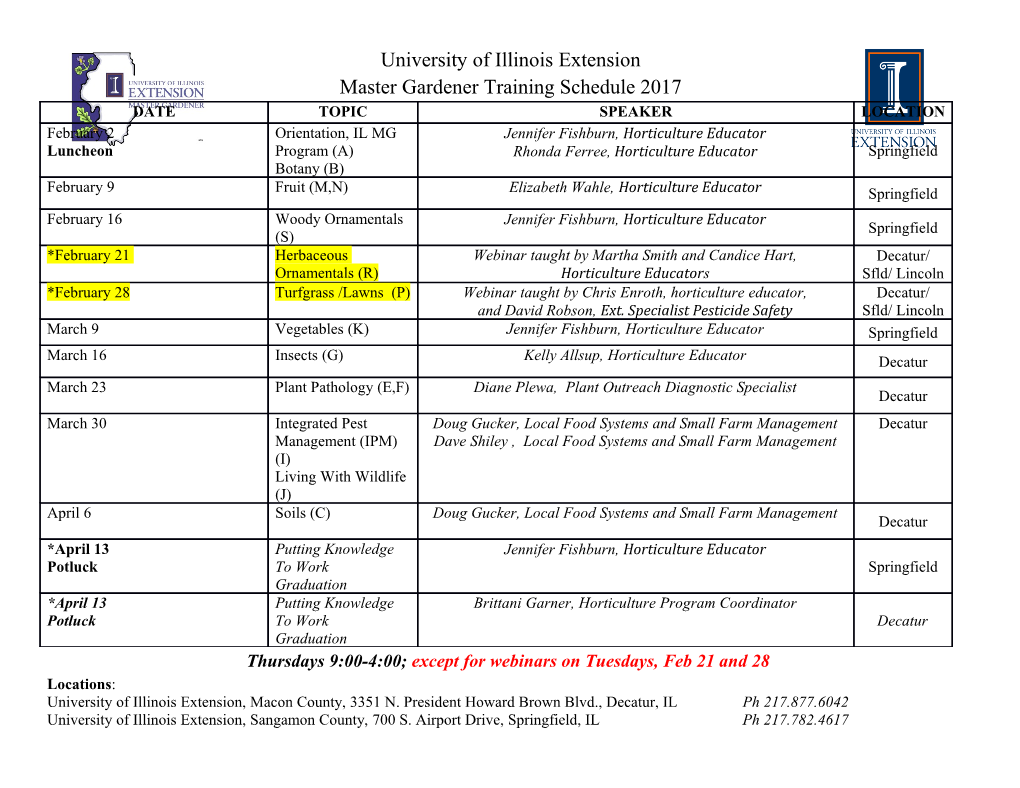
<p> Logistic Regression – Classification by MSE Minimization</p><p>Remember: Classification can be solved by MSE minimization methods (E[y|x] can be used to derive posteriors P(yCj|x)). Question: What functional form f(x;) can be an appropriate choice for representing posterior class probabilities?</p><p>M Option 1: What about linear model f(x;) = j x j ? The range of the function goes beyond 0-1, so it j0 is not a good choice.</p><p>Option 2: We can use sigmoid function to do squeeze the output of a linear model to the range between 0 M and 1: f(x;) = g( j x j ). If g(z) = 1/(1+ez), optimizing f(x;) is called logistic regression. j0</p><p>Solution: Logistic regression can be solved by minimizing MSE. Derivative MSE/j is MSE 2 N M (y f (x;θ))x g' x i1 ij k 0 k ik j N </p><p>Note: Solving MSE = 0 results in (M+1) nonlinear equations with (M+1) unknowns optimization can be done by using gradient descent algorithm. Maximum Likelihood (ML) Algorithm</p><p>Basic Idea: Given a data set D and a parametric model with parameters that describes the data generating process, the best solution * is the one that maximizes P(D|), i.e. </p><p>* = arg max P(D|)</p><p>P(D|) is called the likelihood, so the name of the algorithm that finds the optimal solution * is called the maximum likelihood algorithm. This idea can be applied for both unsupervised and supervised learning problems.</p><p>ML for Unsupervised Learning: Density Estimation</p><p>Given D = {xi, i=1, 2, …N}, and assuming the functional form p(x|) of the data generating process, the goal is to estimate the optimal parameters that maximize likelihood P(D|):</p><p>P(D|) = P(x1, x2, …, xN|)</p><p>By assuming that data points xi are independent and identically distributed (iid) N P(D|) = p(xi | θ) (p is the probability density function.) i1 Since log(x) is monotonically increasing function with x, maximization of P(D|) is equivalent to maximization of l = log(P(D|)). l is called the log-likelihood and is a popular choice when dealing with distributions from the exponential family (see section 2.4). So, N l logp(x | θ) i1 i</p><p>Example: Data set D = {xi, i=1, 2, …N} is drawn from a Gaussian distribution with mean and standard deviation , i.e., X ~ N(,2). Therefore, (, ) , and 2 (xi ) 2 N 1 (xi ) 2 1 2 2 l log p(xi | , ) e i1 2 2 2 2 </p><p>Values and that maximize the log-likelihood satisfy the necessary condition for local optimum: l 1 N l N 2 0 ˆ xi , 0 ˆ 1 x ˆ N i1 N i1 i</p><p>ML for Supervised Learning</p><p>Given D = {(xi,yi), i=1, 2, …N}, and assuming the functional form p(y|x,) of the data generating process, the goal is to estimate the optimal parameters that maximize likelihood P(D|):</p><p>P(D|) = P(y1, y2, …, yN|x1, x2, …, xN,) = /if data is iid N = py | x ,θ i 1 i i ML for Regression</p><p>Assume the data generating process corresponds to: y f (x,θ) e , where e ~ N(,2) Note: this is a relatively strong assumption! y ~ N( f (x,θ),2 )</p><p>(x f (x,θ))2 1 2 p(y | x,θ) e 2 2 N 1 (y f (x ,θ))2 l log P(D | θ) log i i 2 i1 2 2 N Since is a constant, maximization of l is equivalent to minimization of 1 y f (x ,θ)2 N i1 i i Important conclusion: Regression using ML under the assumption of DGP with additive Gaussian noise is equivalent to regression using MSE minimization!!</p><p>ML for Classification</p><p>Logistic Regression</p><p>The assumptions involved in logistic regression are similar to those involved with linear regression, namely the existence of a linear relationship between the inputs and the output. In the case of logistic regression, this assumption takes a somewhat different form: we assume that the posterior class probabilities can be estimated as a linear function of the inputs, passed through a sigmoidal function. Parameter estimates (coefficients of the inputs) are then calculated to minimize MSE. For simplicity, assume we are doing binary classification and that y {0,1} . Then the logistic regression model is 1 j x j P(y C1 | x) , where 1 e j</p><p>The likelihood function of the data D is given by</p><p>N N yi 1- yi p( D |Θ )=照 p ( yi | x i , Θ ) =m i (1 - m i ) i=1 i = 1</p><p> yi 1- yi where we denoted i P(yi C1 | xi ) . Note that the term mi(1- m i ) reduces to the posterior class probability of class 0, i P(yi C0 | xi ) , when yi C0 , and the posterior class probability of class 1, i P(yi C1 | xi ) , when yi C1 . In order to find the ML estimators of the parameters, we form the log-likelihood</p><p>N</p><p>ℓ =logp ( D |Θ ) = [ yi logm i + (1 - y i )log(1 - m i )] i=1</p><p>The ML estimators require us to solve �Θℓ 0 , which is a non-linear system of (M+1) equations in (M+1) unknowns, so we don’t expect a closed form solution. Hence we would, for instance, apply the gradient descent algorithm to get the parameter estimates for the classifier.</p>
Details
-
File Typepdf
-
Upload Time-
-
Content LanguagesEnglish
-
Upload UserAnonymous/Not logged-in
-
File Pages3 Page
-
File Size-