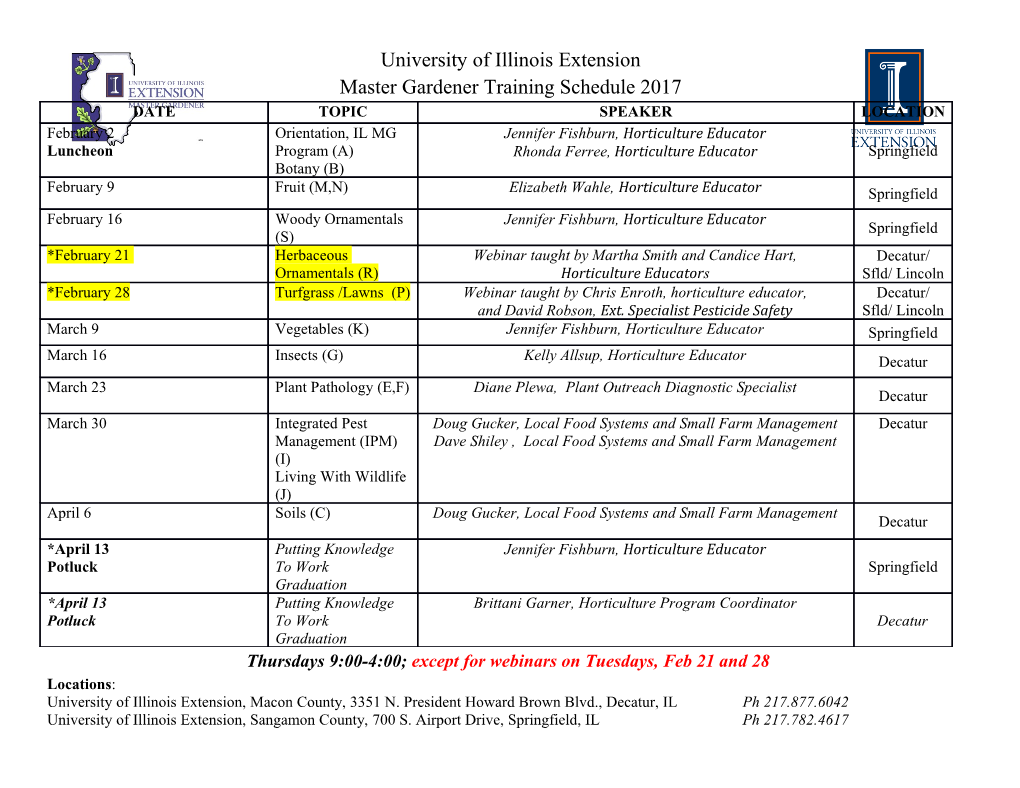
Automatic Transcription of Bass Guitar Tracks applied for Music Genre Classification and Sound Synthesis Dissertation zur Erlangung des akademischen Grades Doktoringenieur (Dr.-Ing.) vorlelegt der Fakultät für Elektrotechnik und Informationstechnik der Technischen Universität Ilmenau von Dipl.-Ing. Jakob Abeßer geboren am 3. Mai 1983 in Jena Gutachter: Prof. Dr.-Ing. Gerald Schuller Prof. Dr. Meinard Müller Dr. Tech. Anssi Klapuri Tag der Einreichung: 05.12.2013 Tag der wissenschaftlichen Aussprache: 18.09.2014 urn:nbn:de:gbv:ilm1-2014000294 ii Acknowledgments I am grateful to many people who supported me in the last five years during the preparation of this thesis. First of all, I would like to thank Prof. Dr.-Ing. Gerald Schuller for being my supervisor and for the inspiring discussions that improved my understanding of good scientific practice. My gratitude also goes to Prof. Dr. Meinard Müller and Dr. Anssi Klapuri for being available as reviewers. Thank you for the valuable comments that helped me to improve my thesis. I would like to thank my former and current colleagues and fellow PhD students at the Semantic Music Technologies Group at the Fraunhofer IDMT for the very pleasant and motivating working atmosphere. Thank you Christian, Holger, Sascha, Hanna, Patrick, Christof, Daniel, Anna, and especially Alex and Estefanía for all the tea-time conversations, discussions, last-minute proof readings, and assistance of any kind. Thank you Paul for providing your musicological expertise and perspective in the genre classification experiments. I also thank Prof. Petri Toiviainen, Dr. Olivier Lartillot, and all the collegues at the Finnish Centre of Excellence in Interdisciplinary Music Research at the University of Jyväskylä for a very inspiring research stay in 2010. Many experiments described in this thesis would not have been possible without the support of other researches in the Music Information Retrieval community. I want to thank Justin Salamon, Matti Ryynänen, and Anssi Klapuri for providing me with their algorithms’ transcription results and Cory McKay for publishing the jSymbolic software. Finally, my biggest thanks go to my family, thank you Harald, Monika, Michel, Wolf for your support and thank you Franziska for your love and patience. Where words fail, music speaks. (Hans Christian Andersen) iii iv Abstract Music recordings most often consist of multiple instrument signals, which overlap in time and frequency. In the field of Music Information Retrieval (MIR), existing algorithms for the automatic transcription and analysis of music recordings aim to extract semantic information from mixed audio signals. In the last years, it was frequently observed that the algorithm performance is limited due to the signal interference and the resulting loss of information. One common approach to solve this problem is to first apply source separation algorithms to isolate the present musical instrument signals before analyzing them individually. The performance of source separation algorithms strongly depends on the number of instruments as well as on the amount of spectral overlap. In this thesis, isolated instrumental tracks are analyzed in order to circumvent the challenges of source separation. Instead, the focus is on the development of instrument-centered signal processing algorithms for music transcription, musical analysis, as well as sound synthesis. The electric bass guitar is chosen as an example instrument. Its sound production principles are closely investigated and considered in the algorithmic design. In the first part of this thesis, an automatic music transcription algorithm for electric bass guitar recordings will be presented. The audio signal is interpreted as a sequence of sound events, which are described by various parameters. In addition to the conventionally used score-level parameters note onset, duration, loudness, and pitch, instrument-specific parameters such as the applied instrument playing techniques and the geometric position on the instrument fretboard will be extracted. Different evaluation experiments confirmed that the proposed transcription algorithm outperformed three state-of-the-art bass transcription algorithms for the transcription of realistic bass guitar recordings. The estimation of the instrument-level parameters works with high accuracy, in particular for isolated note samples. In the second part of the thesis, it will be investigated, whether the sole analysis of the bassline of a music piece allows to automatically classify its music genre. Different score-based audio features will be proposed that allow to quantify tonal, rhythmic, and structural properties of basslines. Based on a novel data set of 520 bassline transcriptions from 13 different music genres, three approaches for music genre classification were compared. A rule-based classification system could achieve a mean class accuracy of 64.8 % by only taking features into account that were extracted from the bassline of a music piece. The re-synthesis of a bass guitar recordings using the previously extracted note parameters will be studied in the third part of this thesis. Based on the physical modeling of string instruments, a novel sound synthesis algorithm tailored to the electric bass guitar will be presented. The algorithm mimics different aspects of the instrument’s sound production mechanism such as string excitement, string damping, string-fret collision, and the influence of the electro-magnetic pickup. Furthermore, a parametric audio coding approach will be discussed that allows to encode and transmit bass guitar tracks with a significantly smaller bit rate than conventional audio v vi coding algorithms do. The results of different listening tests confirmed that a higher perceptual quality can be achieved if the original bass guitar recordings are encoded and re-synthesized using the proposed parametric audio codec instead of being encoded using conventional audio codecs at very low bit rate settings. Zusammenfassung Musiksignale bestehen in der Regel aus einer Überlagerung mehrerer Einzelinstrumente. Die meisten existierenden Algorithmen zur automatischen Transkription und Analyse von Musikauf- nahmen im Forschungsfeld des Music Information Retrieval (MIR) versuchen, semantische Infor- mation direkt aus diesen gemischten Signalen zu extrahieren. In den letzten Jahren wurde häufig beobachtet, dass die Leistungsfähigkeit dieser Algorithmen durch die Signalüberlagerungen und den daraus resultierenden Informationsverlust generell limitiert ist. Ein möglicher Lösungsansatz besteht darin, mittels Verfahren der Quellentrennung die beteiligten Instrumente vor der Anal- yse klanglich zu isolieren. Die Leistungsfähigkeit dieser Algorithmen ist zum aktuellen Stand der Technik jedoch nicht immer ausreichend, um eine sehr gute Trennung der Einzelquellen zu ermöglichen. In dieser Arbeit werden daher ausschließlich isolierte Instrumentalaufnahmen un- tersucht, die klanglich nicht von anderen Instrumenten überlagert sind. Exemplarisch werden anhand der elektrischen Bassgitarre auf die Klangerzeugung dieses Instrumentes hin spezialisierte Analyse- und Klangsynthesealgorithmen entwickelt und evaluiert. Im ersten Teil der vorliegenden Arbeit wird ein Algorithmus vorgestellt, der eine automa- tische Transkription von Bassgitarrenaufnahmen durchführt. Dabei wird das Audiosignal durch verschiedene Klangereignisse beschrieben, welche den gespielten Noten auf dem Instrument entsprechen. Neben den üblichen Notenparametern Anfang, Dauer, Lautstärke und Tonhöhe werden dabei auch instrumentenspezifische Parameter wie die verwendeten Spieltechniken sowie die Saiten- und Bundlage auf dem Instrument automatisch extrahiert. Evaluationsexperimente anhand zweier neu erstellter Audiodatensätze belegen, dass der vorgestellte Transkriptionsalgo- rithmus auf einem Datensatz von realistischen Bassgitarrenaufnahmen eine höhere Erkennungs- genauigkeit erreichen kann als drei existierende Algorithmen aus dem Stand der Technik. Die Schätzung der instrumentenspezifischen Parameter kann insbesondere für isolierte Einzelnoten mit einer hohen Güte durchgeführt werden. Im zweiten Teil der Arbeit wird untersucht, wie aus einer Notendarstellung typischer sich wieder- holender Basslinien auf das Musikgenre geschlossen werden kann. Dabei werden Audiomerkmale extrahiert, welche verschiedene tonale, rhythmische, und strukturelle Eigenschaften von Basslinien quantitativ beschreiben. Mit Hilfe eines neu erstellten Datensatzes von 520 typischen Basslin- ien aus 13 verschiedenen Musikgenres wurden drei verschiedene Ansätze für die automatische Genreklassifikation verglichen. Dabei zeigte sich, dass mit Hilfe eines regelbasierten Klassifika- tionsverfahrens nur Anhand der Analyse der Basslinie eines Musikstückes bereits eine mittlere Erkennungsrate von 64,8 % erreicht werden konnte. Die Re-synthese der originalen Bassspuren basierend auf den extrahierten Notenparametern wird im dritten Teil der Arbeit untersucht. Dabei wird ein neuer Audiosynthesealgorithmus vorgestellt, der basierend auf dem Prinzip des Physical Modeling verschiedene Aspekte der für die Bassgitarre charakteristische Klangerzeugung wie Saitenanregung, Dämpfung, Kollision zwischen Saite und Bund sowie dem Tonabnehmerverhalten nachbildet. Weiterhin wird ein parametrischer vii viii Audiokodierungsansatz diskutiert, der es erlaubt, Bassgitarrenspuren nur anhand der ermittel- ten notenweisen Parameter zu übertragen um sie auf Dekoderseite wieder zu resynthetisieren. Die Ergebnisse mehrerer Hötest belegen, dass der vorgeschlagene
Details
-
File Typepdf
-
Upload Time-
-
Content LanguagesEnglish
-
Upload UserAnonymous/Not logged-in
-
File Pages180 Page
-
File Size-