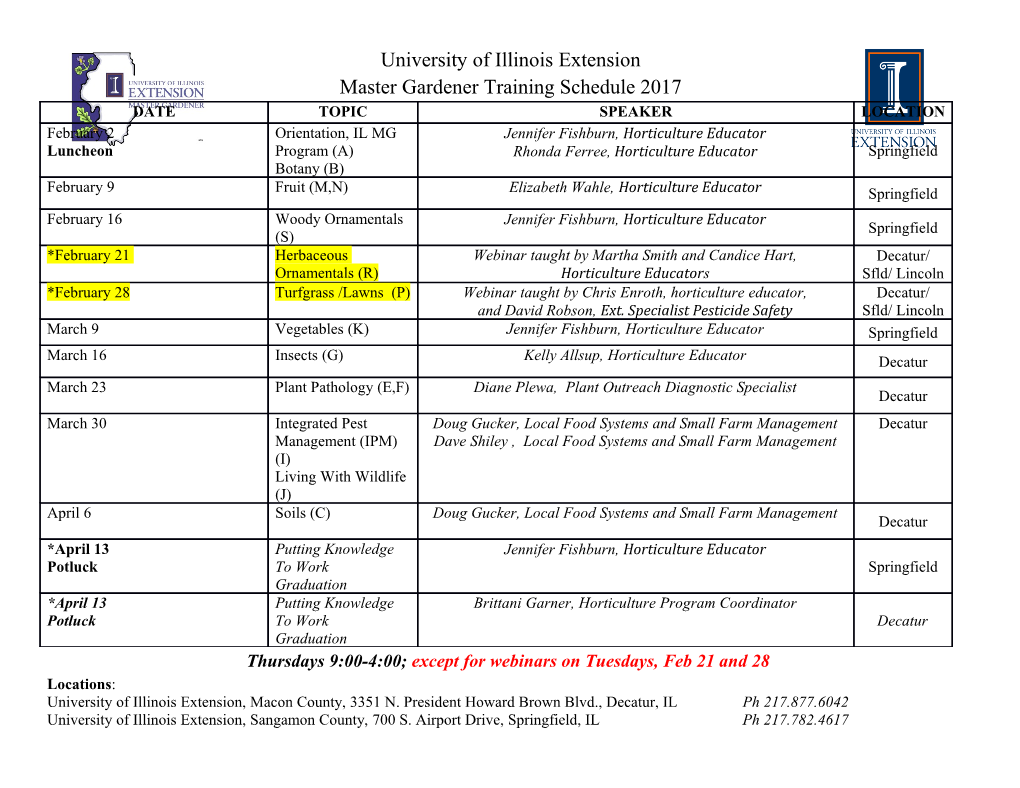
. Magnificent beasts of the Milky Way: Hunting down stars with unusual infrared properties using supervised machine learning Julia Ahlvind1 Supervisor: Erik Zackrisson1 Subject reader: Eric Stempels1 Examiner: Andreas Korn1 Degree project E in Physics { Astronomy, 30 ECTS 1Department of Physics and Astronomy { Uppsala University June 22, 2021 Contents 1 Background 2 1.1 Introduction................................................2 2 Theory: Machine Learning 2 2.1 Supervised machine learning.......................................3 2.2 Classification...............................................3 2.3 Various models..............................................3 2.3.1 k-nearest neighbour (kNN)...................................3 2.3.2 Decision tree...........................................4 2.3.3 Support Vector Machine (SVM)................................4 2.3.4 Discriminant analysis......................................5 2.3.5 Ensemble.............................................6 2.4 Hyperparameter tuning.........................................6 2.5 Evaluation.................................................6 2.5.1 Confusion matrix.........................................6 2.5.2 Precision and classification accuracy..............................7 3 Theory: Astronomy 7 3.1 Dyson spheres...............................................8 3.2 Dust-enshrouded stars..........................................8 3.3 Gray Dust.................................................9 3.4 M-dwarf.................................................. 10 3.5 post-AGB stars.............................................. 10 4 Data and program 10 4.1 Gaia.................................................... 11 4.2 AllWISE ................................................. 11 4.3 2MASS .................................................. 11 4.4 MATLAB................................................. 12 4.4.1 Decision trees........................................... 12 4.4.2 Discriminant analysis...................................... 13 4.4.3 Support Vector Machine..................................... 13 4.4.4 k-nearest neighbour....................................... 13 4.4.5 Ensemble............................................. 13 5 The general method 14 5.1 Forming datasets and building DS models............................... 14 5.1.1 Training set stars......................................... 14 5.1.2 Training set Dyson spheres................................... 14 5.1.3 Training set YSOs........................................ 16 5.2 Finding and identifying the DS candidates............................... 16 5.2.1 Manual analysis of DS candidates............................... 16 5.3 Testing set................................................ 17 5.4 Best fitted model............................................. 18 6 Process 18 6.1 limiting DS magnitudes......................................... 19 6.2 Introducing a third class......................................... 19 6.3 Coordinate dependence......................................... 20 6.4 Malmquist bias.............................................. 21 6.5 cc flag................................................... 22 6.6 Feature selection............................................. 22 6.7 Proportions of the training sets..................................... 23 7 Result 23 7.1 Frequent sources of reference...................................... 24 7.1.1 Marton et al.(2016)....................................... 24 7.1.2 Marton et al.(2019)....................................... 24 7.1.3 Stassun et al.(2018)....................................... 24 7.1.4 Stassun et al.(2019)....................................... 25 7.2 A selection of intriguing targets..................................... 25 7.2.1 J18212449-2536350 (Nr.1).................................... 26 7.2.2 J04243606+1310150 (Nr.8)................................... 27 7.2.3 J18242978-2946492 (Nr.10)................................... 28 7.2.4 J18170389+6433549 (Nr.22)................................... 29 7.2.5 J14492607-6515421 (Nr.26)................................... 30 7.2.6 J06110354-4711294 (Nr.30)................................... 31 7.2.7 J05261975-0623574 (Nr.37)................................... 32 7.2.8 J21173917+6855097 (Nr.46)................................... 33 7.3 Summary of the results......................................... 34 8 Discussion 34 8.1 Evaluation of the approach....................................... 35 8.1.1 The influence of various algorithms............................... 35 8.1.2 Training sets........................................... 36 8.2 Challenges................................................. 37 8.3 Follow-up observations.......................................... 37 8.4 Grid search................................................ 38 8.5 Future prospects and improvements.................................. 39 9 Conclusion 40 A Appendix i A.1 Candidates................................................i A.2 Uncertainty derivations......................................... iii A.3 Results of various models........................................ iv A.4 Algorithm.................................................v A.4.1 linear SVM............................................v A.4.2 quadratic SVM.......................................... vii Abstract The significant increase of astronomical data necessitates new strategies and developments to analyse a large amount of information, which no longer is efficient if done by hand. Supervised machine learning is an example of one such modern strategy. In this work, we apply the classification technique on Gaia+2MASS+WISE data to explore the usage of supervised machine learning on large astronomical archives. The idea is to create an algorithm that recognises entries with unusual infrared properties which could be interesting for follow-up observations. The programming is executed in MATLAB and the training of the algorithms in the classification learner application of MATLAB. Each catalogue; Gaia+2MASS+WISE contains „ 109, 5ˆ108 and 7ˆ108 (The European Space Agency 2019, Skrutskie et al. 2006, R. M. Cutri IPAC/Caltech) entries respectively. The algorithms searches through a sample from these archives consisting of 765266 entries, corresponding to objects within a ă 500 pc range. The project resulted in a list of 57 entries with unusual infrared properties, out of which 8 targets showed none of the four common features that provide a natural physical explanation to the unconventional energy distribution. After more comprehensive studies of the aforementioned targets, we deem it necessary for further studies and observations on 2 out of the 8 targets (Nr.1 and Nr.8 in table3) to establish their true nature. The results demonstrate the applicability of machine learning in astronomy as well as suggesting a sample of intriguing targets for further studies. Sammanfattning Inom astronomi samlas stora m¨angderdata in kontinuerligt och dess tillv¨axt¨okar snabbt f¨orvarje ˚ar.Detta medf¨oratt manuella analyser av datan blir mindre och mindre l¨onsamaoch kr¨aver ist¨allet nya strategier och metoder d¨arstora datam¨angdersnabbare kan analyseras. Ett exempel p˚aen s˚adan strategi ¨arv¨agleddmaskininl¨arning. I detta arbete utnyttjar vi en v¨agledmaskininl¨arningsteknik kallad klassificering. Vi anv¨anderklassificerings tekniken p˚adata fr˚ande tre stora astronomiska katalogerna Gaia+2MASS+WISE f¨oratt unders¨oka anv¨andningenav denna teknik p˚ajust stora astronomiska arkiv. Id´en¨aratt skapa en algorithm som identifierar objekt med okontroversiella infrar¨odaegenskaper som kan vara intressanta f¨orvidare observationer och analyser. Dessa ovanliga objekt ¨arf¨orv¨antade att ha en l¨agreemission i det optiska v˚agl¨angdsomr˚adetoch en h¨ogreemission i det infrar¨oda¨anvad vanligtvis ¨arobserverad f¨oren stj¨arna.Programmeringen sker i MATLAB och tr¨aningsprocessen av algoritmerna i MATLABs applikation classification learner. Algoritmerna s¨oker igenom en samling data best˚aende av 765266 objekt, fr˚ankatalogerna Gaia+2MASS+WISE. Dessa kataloger inneh˚allertotalt „ 109, 5ˆ108 och 7ˆ108 (The European Space Agency 2019, Skrutskie et al. 2006, R. M. Cutri IPAC/Caltech) objekt vardera. Det begr¨ansadedataset som algoritmerna s¨oker igenom motsvarar objekt inom en radie av ă 500 pc. M˚angaav de objekt som algoritmerna identifierade som "ovanliga" tycks i sj¨alva verket vara nebul¨osaobjekt. Den naturliga f¨orklaringenf¨or dess infrar¨oda¨overskott ¨ardet omslutande stoft som ger upphov till v¨armestr˚alningi det infrar¨oda. F¨oratt eliminera denna typ av objekt och fokusera s¨okningenp˚amer okonventionella objekt gjordes modifieringar av programmen. En av de huvudsakliga ¨andringarnavar att introducera en tredje klass best˚aendeav stj¨arnorinneslutna av stoft som vi kallar "YSO"-klassen. Ytterligare en ¨andringsom medf¨ordef¨orb¨attraderesultat var att introducera koordninaterna i tr¨aningensamt vid den slutgiltiga klassificeringen och p˚as˚avis, identifiering av intressanta kandidater. Dessa justeringar resulterade i en minskad andelen nebul¨osaobjekt i klassen av "ovanliga" objekt som algoritmerna identifierade. Projektet resulterade i en lista av 57 objekt med ovanliga infrar¨odaegenskaper. 8 av dessa objekt p˚avisadeingen av det fyra vanligt f¨orekommande egenskaperna som kan ge en naturlig f¨orklaring p˚adess ¨overfl¨odav infrar¨odstr˚alning.Dessa egenskaper ¨ar;nebul¨osomgivning eller p˚avisadstoft, variabilitet, Hα emission eller maser str˚alning. Efter vidare unders¨okningav de 8 tidigare
Details
-
File Typepdf
-
Upload Time-
-
Content LanguagesEnglish
-
Upload UserAnonymous/Not logged-in
-
File Pages58 Page
-
File Size-