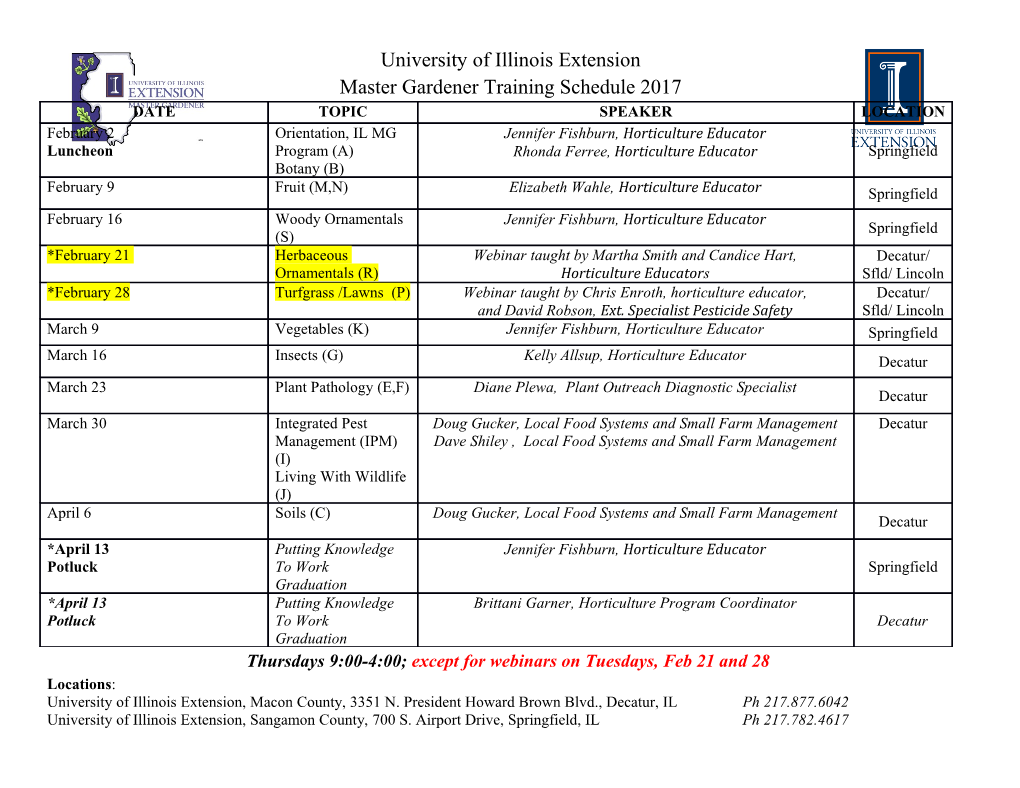
k Trim Size: 7in x 9.25in Oakes c19.tex V1 - 01/11/2017 7:26 P.M. Page 493 CHAPTER NINETEEN NATURAL EXPERIMENTS AND INSTRUMENTAL VARIABLES ANALYSES IN SOCIAL EPIDEMIOLOGY M. Maria Glymour, Stefan Walter, and Eric J. Tchetgen Tchetgen “Alternatives to the experiment will always be needed, and a key issue is to identify which kinds of observational studies are most likely to generate unbiased results.” k k COOK, SHADISH, ET AL. (2008) ocial epidemiology is of little use if it cannot guide us in how to improve Spopulation health. Such guidance depends on understanding the causal structures that harm the health of people exposed to social disadvantage. Although evaluating the effects of social conditions on health is a central task in social epidemiology, the core analytic tools of our discipline are often insuf- ficient; we may not be able to conclusively estimate the magnitude ofhealth effects or even to determine whether important social exposures have any health effects at all. Social epidemiology largely inherited the methodological techniques of the parent discipline of epidemiology, and the usual approach to estimating treatment effects from observational data involves assessing the observed association between the exposure and outcome of interest, adjusting or stratifying to account for likely confounders. Instrumental variables (IV) analyses provide an alternative to this approach when data from either researcher-randomized experiments or natu- ral experiments are available. IV analyses depend strongly on the assumption that the data were generated by a valid experiment, that is, that subjects were 493 k k Trim Size: 7in x 9.25in Oakes c19.tex V1 - 01/11/2017 7:26 P.M. Page 494 494 Methods in Social Epidemiology effectively randomized, even if the randomization process was not initiated by the researcher and adherence to random assignment was low. For many of the exposures of interest in social epidemiology, opportunities to intentionally randomize are few and far between, but natural experiments may be more common. Social determinants of health, such as schooling, poverty, employ- ment, and even marriage, fertility, and social connections, are influenced by administrative policies, state and local laws, and other forces external to the individual (Currie 1995; Angrist and Krueger 2001). These policies sometimes create natural or quasi-experiments in which an individual’s probability of exposure to a particular social condition is influenced by a policy that is uncorrelated with the individual’s personal characteristics or other factors that would affect the outcome of interest. Such natural experiments—in which a random process influences the probability of exposure but this random event is not instigated by researchers—provide a powerful approach to test causal claims. We have organized this chapter to give a broad intuition first and provide greater detail in subsequent sections. The first section discusses the motivations for IV analyses. We then provide a brief introduction to the assumptions required to interpret IV estimates as causal parameters, and k describe approaches to implementing IV analyses. The next section frames k natural experiments and IV estimates in terms of the general problem of causal inference, and walks through a simple numerical example comparing the IV estimate to other parameters. We briefly introduce applications with binary and survival outcomes. We then discuss a few examples of specific instruments that seem especially clever or potentially relevant to social epidemiology. The concluding sections address common points of confusion regarding IV analyses and reiterate the limitations of IV. Much of the descrip- tion below is informal, focusing on intuition. More formal introductions to IV are available in several excellent articles published elsewhere (Angrist et al. 1996; Greenland 2000; Angrist and Krueger 2001; Pearl 2009). IV is also discussed in standard econometrics textbooks (Kennedy 2008; Greene 2000; Wooldridge 2016). Motivations for Using Instrumental Variables in Social Epidemiology Research We recognize three related motivations for using IV approaches in social epidemiology. The first is to estimate the causal effect of treatment, rather than the intent to treat (ITT) effect (defined below). A second major k k Trim Size: 7in x 9.25in Oakes c19.tex V1 - 01/11/2017 7:26 P.M. Page 495 Natural Experiments and Instrumental Variables Analyses in Social Epidemiology 495 motivation for IV analyses is to circumvent the problem of unmeasured confounders when it seems unlikely, or at least uncertain, that all of the confounding bias between the exposure and outcome can be addressed using regression adjustment or related methods. A third motivation for using IV analyses in social epidemiology is that the IV effect estimate may correspond to a parameter of substantive interest that cannot be directly estimated in conventional models, such as the effect of treatment differences induced by changes in the IV. IV analyses, although often used in natural or quasi-experimental settings, are also relevant in researcher randomized trials, such as the Moving To Opportunity (MTO) experiment (Ludwig et al. 2011). Estimating the Effect of Being Treated Instead of the Effect of Being Randomized Randomized trials are considered to provide the most convincing evidence for causal effects (Byar et al. 1976; Abel and Koch 1999; DeMets 2002). ITT analyses—in which the outcomes of those assigned to a treatment group are contrasted with the outcomes of those assigned to a comparison group—are the standard analytical method for data from randomized trials. Because of k non-compliance, the ITT estimates do not estimate the effect of receiving the k treatment (versus the comparison) on the outcome. Instead, ITT analysis estimates the effect of being assigned to receive the treatment on the subjects’ health. If the ITT effect estimate in a valid RCT is significantly positive (benefi- cial), this indicates that the treatment improves outcomes for at least some individuals in the population, but it does not reveal the magnitude of that relationship. It is often critical to learn whether the treatment effect is large or small. For example, the MTO trial randomized families residing in public housing developments to either receive a housing voucher to subsidize rental of a private market apartment or to receive no such voucher (and remain in public housing). Of those offered a voucher, only about half took advantage of the offer and moved to a private market rental. At 4–7 years after random- ization, girls aged 12–17 in families who were randomized to receive a voucher were 12 percentage points less likely to meet criterion for psychological distress (measured with the Kessler 6 inventory). Accounting for non-adherence with an IV estimate indicated the impact of moving to a private market apartment for girls was a 23 percentage point reduction in the probability of psychological distress (Osypuk et al. 2012). Several alternatives to the ITT effect estimate rely on defining the exposure variable in the analysis to be the treatment each participant received k k Trim Size: 7in x 9.25in Oakes c19.tex V1 - 01/11/2017 7:26 P.M. Page 496 496 Methods in Social Epidemiology (regardless of their randomly assigned treatment). These approaches reintroduce confounding if there are common causes of treatment take-up and the outcome. For example, directly comparing the treated to the untreated (sometimes called as-treated analysis) or comparing compliers in the treatment arm to compliers in the control arm (per-protocol analysis) are well-known and long-rejected options (Hennekens and Buring 1987; Weiss 1998). IV analyses, however, can, under certain assumptions, provide an unconfounded estimate of the effect of treatment even if the association between treatment taken and the outcome is subject to unmeasured con- founding. IV analyses do not directly compare treated to untreated people. IV analyses therefore avoid conditioning on post-randomization information to define the exposure variable. A caveat, explained in more detail below,is that if the effect of treatment is not the same for everyone in the population, IV analyses may not provide the average treatment effect for the whole population. Avoiding Bias Due to Confounding in Observational Data Analyses In the absence of randomized trial data, epidemiologists typically attempt k to draw causal inferences from observational data by adjusting for common k causes (confounders) of the exposure and the outcome. Such adjustment may take the form of regression adjustment (i.e., conditioning on the con- founder in a regression model), standardization, stratification, restriction, or propensity score matching. These approaches have a common goal: identify an observed set of covariates such that, within strata of the covariates, the statistical association between treatment and outcome can be entirely attributed to the causal effect of the former on the latter (Greenland et al. 1999). The principal limitation of this approach is that such a set of covariates is rarely fully observed. Even if all common causes of the exposure and the outcome are known at a conceptual level, have they been well measured? Is the regression model incorporating such confounders correctly specified? If not, residual confounding could remain a threat to validity. Although recent meta-analyses suggest observational designs evaluating clinical interventions are not systematically biased compared to RCTs (Anglemyer et al. 2014), findings from observational studies often differ substantially from whatis achieved with RCTs (McCarrey and Resnick 2015), and the problem may be especially acute in social epidemiology (Berkman 2009). With a valid instrument, IV analyses can sometimes be used to estimate the effect of the treatment even if important confounders of the treatment-outcome relation are not accounted for. k k Trim Size: 7in x 9.25in Oakes c19.tex V1 - 01/11/2017 7:26 P.M. Page 497 Natural Experiments and Instrumental Variables Analyses in Social Epidemiology 497 Estimating Complier-Specific Treatment Effects The health effects of most exposures vary across individuals.
Details
-
File Typepdf
-
Upload Time-
-
Content LanguagesEnglish
-
Upload UserAnonymous/Not logged-in
-
File Pages46 Page
-
File Size-