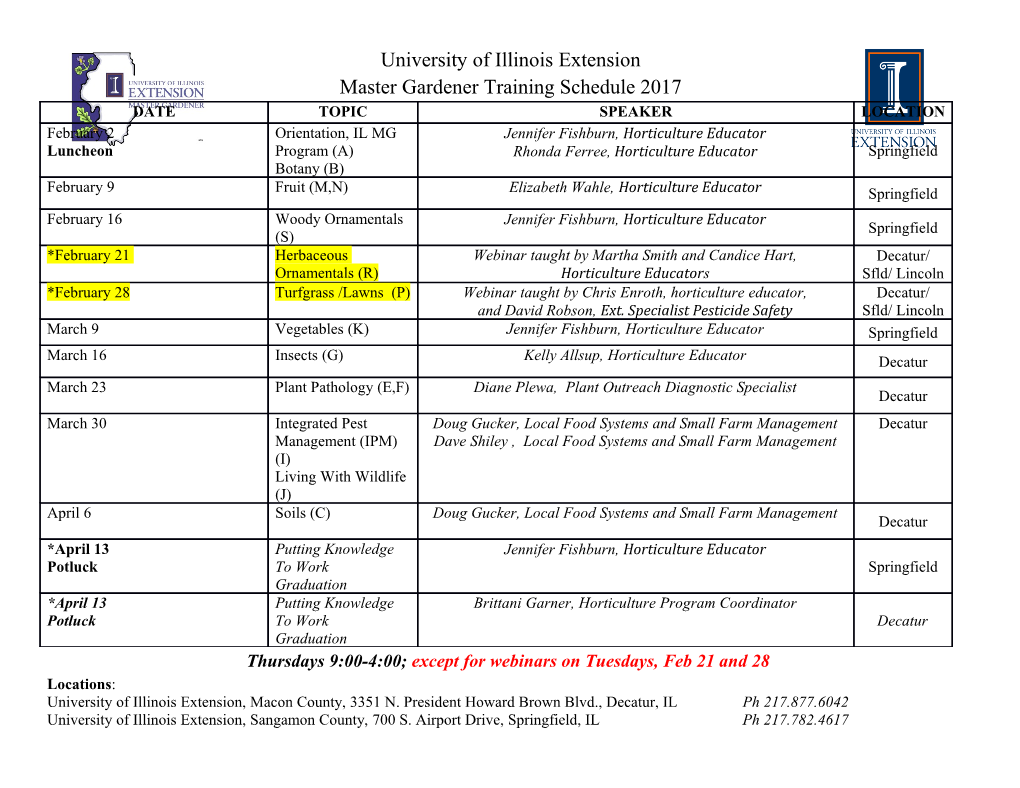
Module 5: Interval Estimation Statistics (OA3102) Professor Ron Fricker Naval Postgraduate School Monterey, California Reading assignment: WM&S chapter 8.5-8.9 Revision: 1-12 1 Goals for this Module • Interval estimation – i.e., confidence intervals – Terminology – Pivotal method for creating confidence intervals • Types of intervals – Large-sample confidence intervals – One-sided vs. two-sided intervals – Small-sample confidence intervals for the mean, differences in two means – Confidence interval for the variance • Sample size calculations Revision: 1-12 2 Interval Estimation • Instead of estimating a parameter with a single number, estimate it with an interval • Ideally, interval will have two properties: – It will contain the target parameter q – It will be relatively narrow • But, as we will see, since interval endpoints are a function of the data, – They will be variable – So we cannot be sure q will fall in the interval Revision: 1-12 3 Objective for Interval Estimation • So, we can’t be sure that the interval contains q, but we will be able to calculate the probability the interval contains q • Interval estimation objective: Find an interval estimator capable of generating narrow intervals with a high probability of enclosing q Revision: 1-12 4 Why Interval Estimation? • As before, we want to use a sample to infer something about a larger population • However, samples are variable – We’d get different values with each new sample – So our point estimates are variable • Point estimates do not give any information about how far off we might be (precision) • Interval estimation helps us do inference in such a way that: – We can know how precise our estimates are, and – We can define the probability we are right Revision: 1-12 5 Terminology • Interval estimators are commonly called confidence intervals • Interval endpoints are called the upper and lower confidence limits • The probability the interval will enclose q is called the confidence coefficient or confidence level – Notation: 1-a or 100(1-a)% – Usually referred to as “100(1-a)” percent CIs Revision: 1-12 6 Confidence Intervals: The Main Idea • Via the CLT, we know that Y is within 2 std errors ( Y n ) of m 95% of the time • So, m must be within 2 SEs of 95% of the time (Unobserved) sampling distribution of the mean y 95% confidence (Unobserved) mY interval for mY (Unobserved) population distribution (pdf of Y) 7 mYY 2 n In General • A two-sided confidence interval: Lower confidence Upper confidence limit limit ˆˆ PrqLU q q 1 a Target Confidence parameter coefficient • A lower one-sided confidence interval: ˆ PrqL q 1 a • An upper one-sided confidence interval: ˆ Prq qU 1 a Revision: 1-12 8 Pivotal Method: A Strategy for Constructing CIs • Pivotal method approach – Find a “pivotal quantity” that has following two characteristics: • It is a function of the sample data and q, where q is the only unknown quantity • Probability distribution of pivotal quantity does not depend on q (and you know what it is) • Now, write down an appropriate probability statement for the pivotal quantity and then rearrange terms… Revision: 1-12 9 Example: Constructing a 95% CI for m, known (1) • Let Y1, Y2, …, Yn be a random sample from a normal population with unknown mean mY and known standard deviation Y • Create a CI for mY based on the sampling distribution of the mean: 2 Y~ NmYY , / n • To start, we know that (via standardizing): Y m Y ~N (0,1) Y / n Revision: 1-12 10 Example: Constructing a 95% CI for m, known (2) • Now for Z ~ N(0,1) we know Pr( 1.96 Z 1.96) 0.95 – That is, there is a 95% probability that the random variable Z lies in this fixed interval • Thus Y -mY Pr -1.96 1.96 0.95 Y / n • So, let’s derive a 95% confidence interval… Revision: 1-12 11 Example: Constructing a 95% CI for m, known (3) Y -mY Pr -1.96 1.96 0.95 Y / n Revision: 1-12 12 Example: Constructing a 95% CI for m, known (4) • So, If Y1 = y1, Y2 = y2, …, Yn = yn are observed values of a random sample from a N m , 2 with known, then Y y m1.96 is a 95% confidence interval for Y n • We can be 95% confident that the interval covers the population mean – Interpretation: In the long run, 19 times out of 20 the interval will cover the true mean and 1 time out of 20 it will not Revision: 1-12 13 Calculating a Specific CI • Consider an experiment with sample size n=40, y 5.426 and Y=0.1 • Calculate a 95% confidence interval for mY Revision: 1-12 14 Example 8.4 • Suppose we obtain a single observation Y from an exponential distribution with mean q. Use Y to form a confidence interval for q with confidence level 0.9. • Solution: Revision: 1-12 15 Example 8.4 (continued) Revision: 1-12 16 Example 8.5 • Suppose we take a sample of size n=1 from a uniform distribution on [0,q ], were q is unknown. Find a 95% lower confidence bound for q. • Solution: Revision: 1-12 17 Example 8.5 (continued) Revision: 1-12 18 Large-Sample Confidence Intervals • If q ˆ is an unbiased statistic, then via the CLT qˆ q Z qˆ has an approximate standard normal distribution for large samples • So, use it as an (approximate) pivotal quantity to develop (approximate) confidence intervals for q Revision: 1-12 19 Example 8.6 • Let qˆ ~ N ( q , ) . Find a confidence interval qˆ for q with confidence level 1-a. • Solution: Revision: 1-12 20 Example 8.6 (continued) Revision: 1-12 21 One-Sided Limits • Similarly, we can determine the 100(1-a)% one-sided confidence limits (aka confidence bounds): 100(1a )% lower bound for q qˆ z – a qˆ – 100(1 a )% upper bound for q qˆ z a qˆ • What if you use both bounds to construct a two-sided confidence interval? – Each bound has confidence level 1-a, so resulting interval has a 1-2a confidence level Revision: 1-12 22 Example 8.7 • The shopping times of n=64 randomly selected customers were recorded with y 33 2 minutes and s y 256 . Estimate m, the true average shopping time per customer with confidence level 0.9. • Solution: Revision: 1-12 23 Example 8.7 (continued) Revision: 1-12 24 Example 8.8 • Two brands of refrigerators, A and B, are each guaranteed for a year. Out of a random sample of nA=50 refrigerators, 12 failed before one year. And out of an independent random sample of nB=60 refrigerators, 12 failed before one year. Give a 98% CI for pA-pB. • Solution Revision: 1-12 25 Example 8.8 (continued) Revision: 1-12 26 Example 8.8 (continued) Revision: 1-12 27 What is a Confidence Interval? • Before collecting data and calculating it, a confidence interval is a random interval – Random because it is a function of a random variable (e.g., Y ) • The confidence level is the long-run percentage of intervals that will “cover” the population parameter – It is not the probability a particular interval contains the parameter! • This statement implies that the parameter is random • After collecting the data and calculating the CI the interval is fixed – It then contains the parameter with probability 0 or 1 Revision: 1-12 28 A CI Simulation • Simulated 20 95% confidence intervals with samples of size n=10 drawn from N(40,1) distribution • One failed to cover the true (unknown) parameter, which is what is expected on average Revision: 1-12 29 Another CI Simulation • Simulated 100 95% confidence intervals with samples of size n=10 drawn from N(40,1) distribution • 6 failed to cover the true (unknown) parameter – Close to the expected number: 5 Revision: 1-12 30 Illustrating Confidence Intervals This is a demonstration showing confidence intervals for a proportion. TO DEMO Applets created by Prof Gary McClelland, University of Colorado, Boulder You can access them at www.thomsonedu.com/statistics/book_content/0495110817_wackerly/applets/seeingstats/index.html Revision: 1-12 31 Summary: Constructing a Two-sided Large-Sample Confidence Interval • For an unbiased statistic q ˆ , determine qˆ • Choose the confidence level: 1-a • Find z a /2 – E.g., for a = 0.05, z 0.025 1.96 • Given data, calculate and • Then the 100(1-a)% confidence interval for q is qˆˆzz , q aa/2qqˆˆ /2 Revision: 1-12 32 E.g., Constructing a Two-sided Large-Sample 95% CI for m • Y is an unbiased estimator for m, and we know Y Y n The confidence level is 1-a = 0.95 • So zza /2 0.025 1.96 • Given data, calculate y and the 95% CI for m is y1.96 n , y 1.96 n YY Revision: 1-12 33 E.g., Constructing a Two-sided Large-Sample 95% CI for p • For Y, the number of successes out of n trials, an unbiased estimator for p is pˆ Y/ n • Then note that pˆ p(1 p ) / n – Follows from: Var(Y / n ) Var( Y ) / n22 np (1 p ) / n zza /2 0.025 1.96 – And, since we don’t know p, ˆpˆ p ˆ(1 p ˆ ) / n • As before, for a confidence level of 1-a = 0.95, • So, the 95% CI for m is pˆ1.96 p ˆ 1 p ˆ n , p ˆ 1.96 p ˆ 1 p ˆ n Revision: 1-12 34 How Confidence Intervals Behave • Width of CI’s: wz2 Y a /2 n Y • Margin of error: Ez a /2 n – Bigger s.d.
Details
-
File Typepdf
-
Upload Time-
-
Content LanguagesEnglish
-
Upload UserAnonymous/Not logged-in
-
File Pages62 Page
-
File Size-