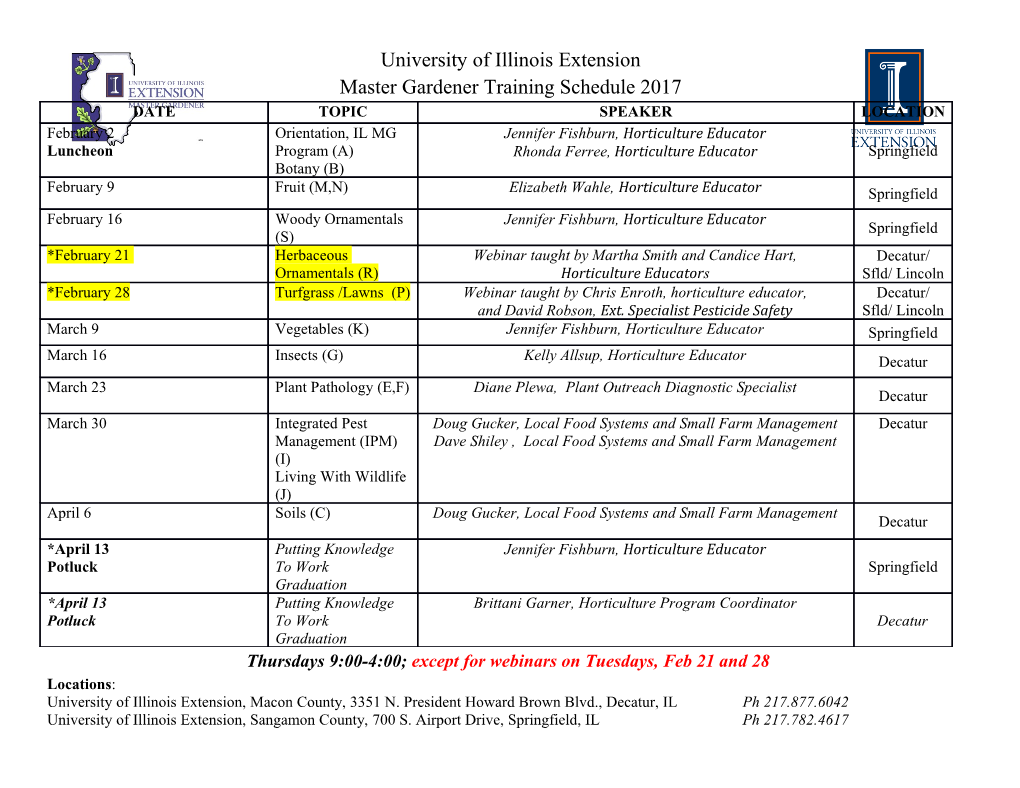
LETTER Communicated by Manu Rastogi ASIC Implementation of a Nonlinear Dynamical Model for Hippocampal Prosthesis Zhitong Qiao [email protected] Yan Han [email protected] Xiaoxia Han [email protected] Institute of Microelectronics and Nanoelectronics, Zhejiang University, Hangzhou 310027, China Han Xu [email protected] School of Medicine, Zhejiang University, Hangzhou 310058, China Will X. Y. Li [email protected] School of Computer Science and Engineering, Nanjing University of Science and Technology, Nanjing 210094, China Dong Song [email protected] Theodore W. Berger [email protected] Department of Biomedical Engineering, Center for Neural Engineering, University of Southern California, Los Angeles, CA 90089, U.S.A. Ray C. C. Cheung [email protected] Department of Electronic Engineering, City University of Hong Kong, Hong Kong 999077, China A hippocampal prosthesis is a very large scale integration (VLSI) biochip that needs to be implanted in the biological brain to solve a cognitive dys- function. In this letter, we propose a novel low-complexity, small-area, and low-power programmable hippocampal neural network application- specific integrated circuit (ASIC) for a hippocampal prosthesis. Itis based on the nonlinear dynamical model of the hippocampus: namely multi-input, multi-output (MIMO)–generalized Laguerre-Volterramodel (GLVM). It can realize the real-time prediction of hippocampal neural Neural Computation 30, 2472–2499 (2018) © 2018 Massachusetts Institute of Technology doi:10.1162/neco_a_01107 Downloaded from http://www.mitpressjournals.org/doi/pdf/10.1162/neco_a_01107 by guest on 29 September 2021 ASIC Implementation of Hippocampal Neural Networks 2473 activity. New hardware architecture, a storage space configuration scheme, low-power convolution, and gaussian random number generator modules are proposed. The ASIC is fabricated in 40 nm technology with acoreareaof0.122mm2 and test power of 84.4 μW. Compared with the design based on the traditional architecture, experimental results show that the core area of the chip is reduced by 84.94% and the core power is reduced by 24.30%. 1 Introduction The hippocampus, an important part of the brain system, is mainly respon- sible for the formation of memory and spatial positioning. Research has found that the hippocampus is mainly responsible for the formation of new memories (Valiant, 2012). Therefore, damage to the hippocampus and sur- rounding regions of the medical temporal lobe can result in a permanent loss of the ability to form new long-term memories, causing cognitive dys- function such as Alzheimer’s disease (AD) and other dementia (Berger, Orr, & Orr, 1983; Eichenbaum, Fagan, Mathews, & Cohen, 1988; Milner, 1970; Squire & Zola-Morgan, 1991). Thus far, most of the drug treatment pro- grams have failed to treat AD. Some drugs can reduce the rate of cognitive decline in patients with early AD but cannot repair nerve damage; more- over, these drugs still present some undesirable side effects (Mullard, 2016; Sevigny et al. 2016). Clearly the effect of drug treatment to alleviate cogni- tive decline is very limited. Hippocampal cognitive neural prosthesis, or hippocampal prosthesis for short, has been proposed to address this issue by replacing damaged tissue with a neurochip that mimics the functions of the original biologi- cal circuitry. It is used to replace the damaged region of the hippocampus (CA3–CA1 path) and thereby repair the memory and cognitive dysfunction caused by damage to the hippocampus. It consists of five modules: a low- noise amplifier (LNA), an analog-to-digital converter (ADC), a spike sorter, a multi-input, multi-output response model (MIMO–GLVM), and a charge- metering stimulus amplifier (CM), as shown in Figure 1 (Berger et al., 2012). The analog front end consists of 16 LNAs and 16 ADCs in parallel, that is, 16 input electrodes implanted in the hippocampus deliver neural signals for amplification and digitization. The digitized signals are then classified by 16 spike sorters into spike event channels, where events are represented by a single bit. Outputs (responses to the spike events) are computed by a single MIMO–GLVM-based hippocampal neural network, which delivers 8 channels of output to 8 CMs. Currently, probe technology and the size of the hippocampus in rats limit the chip to 16 parallel inputs and 8 differential outputs. In this letter, we focus on the research and implementation of the hip- pocampal neural network application-specific integrated circuit (ASIC) for Downloaded from http://www.mitpressjournals.org/doi/pdf/10.1162/neco_a_01107 by guest on 29 September 2021 2474 Z. Qiao et al. Figure 1: Functional block diagram of the hippocampal prosthesis. hippocampal prosthesis. It is based on the nonlinear dynamical model of hippocampus, MIMO–GLVM. The MIMO–GLVM-based hippocampal neu- ral network is an artificial neural network (ANN). It is the core module and mainly realizes the memory function of hippocampus, that is, it converts short-term memory into long-term memory. Because of the similarity be- tween an ANN and a neural network (NN), it can be used to replace the damaged CA3–CA1 pathway in the hippocampus, completing the normal processing and transmission of neural signals. (The structure of the hip- pocampal CA3–CA1 pathway and the implantation diagram of the hip- pocampal prosthesis are in Figures 2 and 3 of Berger et al., 2012.) In recent years, ANN has developed rapidly. An increasing number of research groups are developing VLSI chips that implement hundreds to thousands of spiking neurons with biophysically realistic dynamics, with the intention of emulating brain-like real-world behavior in hardware and robotic systems rather than simply simulating their performance on general-purpose digital computers (Neftci, Chicca, Indiveri, & Douglas, 2011; Martí, Rigotti, Seok, & Fusi, 2016; Cymbalyuk, Patel, Calabrese, De- Weerth, & Cohen, 2000; Bartolozzi & Indiveri, 2007; Giulioni, Pannunzi, Badoni, Dante, & Giudice, 2009). For example, IBM has developed mil- lions of neurons of integrated circuits, TrueNorth. It reaches the level of su- percomputers, but with extremely low power consumption (Merolla et al., 2014; Service, 2014). A convolutional neural network (CNN) is a particular kind of ANN specifically designed for hardware implementation, usually in embedded and real-time systems, such as image processing applications (Karahaliloglu, Gans, Schemm, & Balkir, 2008; Rawat & Wang, 2017; Chen, Downloaded from http://www.mitpressjournals.org/doi/pdf/10.1162/neco_a_01107 by guest on 29 September 2021 ASIC Implementation of Hippocampal Neural Networks 2475 Krishna, Emer, & Sze, 2017). Its main goals are to improve system speed and reduce system power consumption. Unlike these other studies, our study is aimed at the neural prosthesis that needs to be implanted in the biological brain. Because the frequency of neural spike signal is very low, the working speed of the MIMO hip- pocampal NN is not very high as long as the oversampling frequency can be achieved. It achieves the computation performance of super computers with extremely low power consumption (Merolla et al., 2014; Service, 2014). The ASIC platform has the following outstanding advantages com- pared with the field programmable gate array (FPGA) software simulation platform: 1. The architecture is customized, so it is efficient in area, power, and speed. 2. The die area is very small, so that it can be implanted in the organism. 3. It can integrate digital and analog circuits on a single chip. The ASIC will serve as the main platform for the realization of hip- pocampal prosthesis. As the core module, the ASIC design of the MIMO hippocampal NN is very important. Currently, the only available work on ASIC-based MIMO hippocampal NNs is in Berger et al. (2012). That paper proposes a prototype of the hip- pocampal prosthesis ASIC, which was fabricated in a 180 nm process. The study gives a detailed introduction to the GLVM algorithm, but little in- formation on the specific implementation of the circuit and corresponding area, power consumption, accuracy, and functional test results of the chip. An FPGA-based MIMO hippocampal NN hardware architecture is pro- posed to realize the coefficient’s estimation of the GLVM and prediction of neuronal population firing activity (Li, Cheung et al., 2013a, 2013b; Li,Xin et al., 2014; Li, Chan et al. 2011a, 2011b). Actually, the coefficient’s estimation module is very complex, and does not need to be implanted in the brain. Therefore, the coefficient’s estimation function can be realized outside the brain; only the prediction function needs to be realized by ASIC to be im- planted into the brain. After the coefficient’s estimation process is finished, the coefficients can be sent to the ASIC, so the hippocampal NN needsto be programmable. Considering that nerve cells are sensitive to heat and that battery life is vital, researchers found that chips with low power are critical. However, not much work is available on appropriate low-power design technology. In this letter, we present an entire MIMO–GLVM based programmable hippocampal NN ASIC. Compared with other work, we focus on the new architecture, low-power, and low complexity ASIC design, based on the ad- vanced 40 nm process. We offer a detailed ASIC implementation scheme. In addition, our test has validated the function. Downloaded from http://www.mitpressjournals.org/doi/pdf/10.1162/neco_a_01107 by guest on 29 September 2021 2476 Z. Qiao et al. Our work makes the following contributions: 1. A novel power- and area-efficient programmable hippocampal NN ASIC architecture. The ASIC is fabricated in a 40 nm process with a core area of 0.122 mm2 and test power of 84.4 μW. Compared with the traditional architecture, our experimental results show that the core area of the chip is reduced by 84.94% and the core power by 24.30%. 2. A highly efficient storage space configuration scheme for the hip- pocampal NN that has high utilization of physical space with little wasted storage space.
Details
-
File Typepdf
-
Upload Time-
-
Content LanguagesEnglish
-
Upload UserAnonymous/Not logged-in
-
File Pages28 Page
-
File Size-