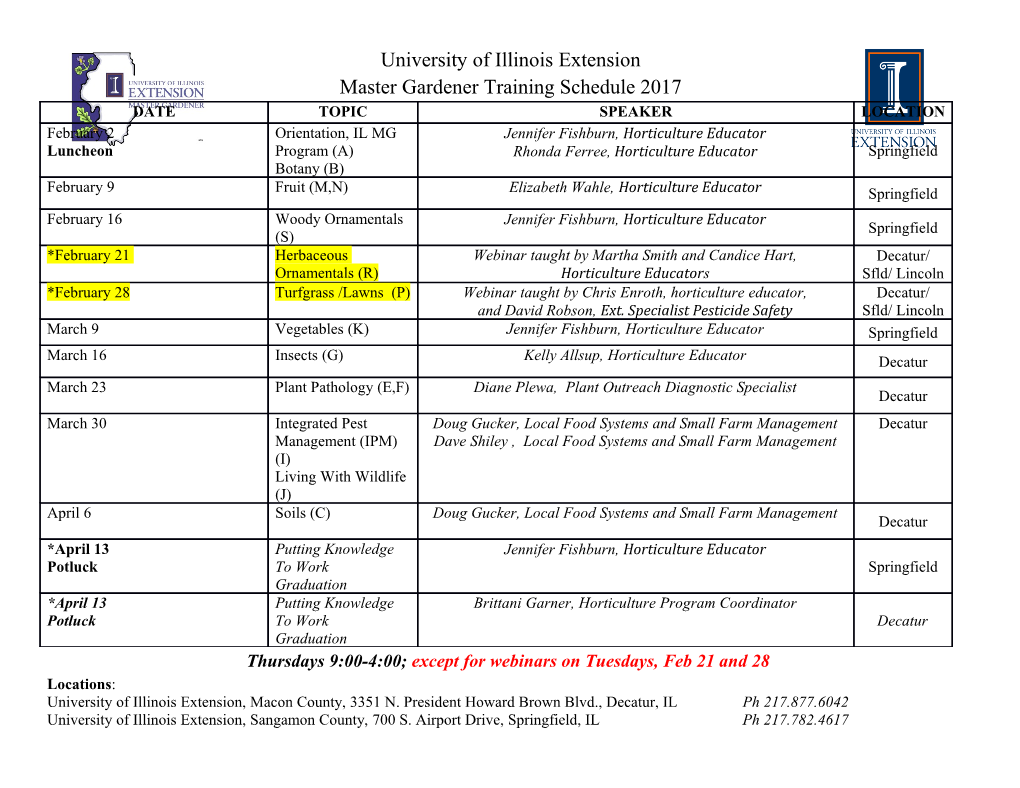
Spatial Analysis of Factors Predicting Bicycle Ridership Andrew Harvey Department of Resource Analysis, Saint Mary’s University of Minnesota, Winona, MN 55987 Keywords: Bicycling Economics, Urban Economics, Transportation Efficiency, Community Health Abstract As cities become more congested and city budgets continue to be strained, both commuters and cities are increasingly looking to bicycles as a viable mode of transportation. At present, bicycle commuting is not very well studied. This study is dedicated to one method that could help target investment helping to increase bicycling as a viable form of transportation in the eyes of the general public. Ordinary Least Squares analysis was applied to variables identified in literature as important factors that potentially correlate with a higher percentage of bicycle commuting ridership. The study area was the extent of Nice Ride Stations located within the City of Minneapolis, Minnesota. Introduction 2017). Despite the comparatively low number of transit miles and comparatively Significance of Research low level of investment in transit infrastructure, transit ridership numbers A majority of American transportation are on the rise (BTS, 2016). Ridership infrastructure has been built for the numbers have increased from below 8 automobile (Bureau of Transportation billion passenger trips per year to over 12 Statistics [BTS], 2016). As of 2013, there billion in 2013 (BTS, 2016). Yet in 2013, were 7,731 miles of commuter rail, 1,622 vehicles driven alone remained the lion's miles of heavy rail (subway like share of the daily commute at 76.5%, and transportation), and 1,836 miles of light at a distant second was carpooling at 9.2% rail (BTS, 2016). At the same time, there (BTS, 2016). were 8,656,070 lane miles of public roads Given that, decisions may be made (BTS, 2016). Over the course of the next to decide whether to reinvest in this several years a significant investment in crumbling road infrastructure (BTS, 2016) the upkeep of this aging infrastructure will with the question to be asked is: are there be necessary (BTS, 2016). There were modes of transportation with a better roughly 50,000 structurally deficient return on investment? In multiple studies, bridges as of 2014 (BTS, 2016). bicycling is demonstrated to be of a net 2,677,771,000,000 miles were benefit per mile ridden, while automobile travelled in 2013 just in passenger cars use has a net cost to society (Blue, 2016). alone (National Highway Traffic Safety So why would people still drive? Because Administration (NHTSA), 2017). it is the easy choice; rather than expending However, there has been a significant the mental and physical energy it takes to reduction in the increase of miles driven ride, just turn the key and the oil does the since the recession of 2008 (NHTSA, work for you. However, are there ________________________________________________________________________ Harvey, Andrew. 2017 Spatial Analysis of Factors Predicting Bicycle Ridership. Volume 20, Papers in Resource Analysis. 12 pp. Saint Mary’s University of Minnesota University Central Services Press. Winona, MN. Retrieved (date) from http://www.gis.smumn.edu impediments that could be removed or Midtown Greenway), were built more as incentives that could be offered that would recreational amenities. Those trails increase the share of commuters taking to though, such as the Midtown Greenway the roads on bicycles, thereby reducing the and Cedar Lake Trails in Minneapolis, are burden on society of the costliest purpose-built commuter trails, although transportation option? they do also function for recreation. In fact, it is faster to get across South Delimitations of the Problem Minneapolis on the Midtown Greenway on a bicycle than it is to traverse the main Delimitations consist of testing variables automobile thoroughfare, Lake Street, by that literature suggested are linked to automobile; not just by a little bit, but by bicycle commuting ridership via Ordinary nearly half (Midtown Greenway Coalition Least Squares Regression analysis, also 2017). The Cedar Lake Trail, shown in known as OLS. Literature suggested Figure 1 was built in 1995 and has three bicycle commuting data is hard to come lanes to help improve traffic flow. One by. Data was mined from the Nice Ride lane is for pedestrians, and the other two bikeshare system’s ridership data from are for bicycles, one lane for each 2016 and tested against various direction. independent variables. The goal was to find several factors that are correlated with ridership and find the most cost-effective infrastructure to invest in. At the same time, variables were added to address the concern of confounding variables. These variables were added to help reduce the risk of correlation based on multicollinearity with some other factor. Data Collection Techniques Funding for bicycle commuter studies has Figure 1. Cedar Lake Trail (Flynn, 2017). traditionally been out of step with the funding provided for similar studies of The most common ridership data automobile traffic. That is to say collection method is the one the City of traditionally the funding is just not there. Minneapolis uses. The city selects a day in Financial outlays for bicycle infrastructure the fall – usually in September – and posts have been low relative to other forms of trained “counters” at random places which transportation infrastructure. Traditional are rotated each count year as well as pre- bicycle infrastructure such as bike lanes, selected places that are counted year after or sharrows, cost so little that the problem year (Minge, Falero, Lindsey, Petesch, and is less about funding than it is about taking Vovick, 2017). A sample of the City of space from other uses, usually parking Minneapolis ridership counts can be seen (Blue, 2016). The more costly pieces of in Figure 2. bicycle infrastructure, such as off-street bicycle trails, many of which are placed in former railroad right of ways (e.g., 2 that have the highest potential to increase commuting ridership in the City of Minneapolis? Nice Ride Data was parsed into several different subsets, which will be covered in further detail. The main independent variables tested were educational attainment, canopy cover, educational enrollment, distance to the next nearest station, and distance to the nearest bicycle infrastructure of different types. Figure 2. Sample bicycle counts (red dots) represents points collected every year. Yellow dots Methods represent areas collected every three to four years (City of Minneapolis Department of Public Works, 2017). Nice Ride makes their ridership data freely available. The data contains start station, New methods are emerging that end station, member status, and time could increase the effectiveness of analysis stamps for both the start and end of the trip on bicycle commuting data. There is an in tabular format. An example of a few increasing amount of road sensors that can Nice Ride raw records can be seen in detect the particular characteristics of Figure 3. In addition, Nice Ride provides bicycles and count them as they ride by. the geographic locations of their stations These are similar counts as would be in tabular format with XY coordinates. obtained by the typical rubber hose counters, but will not be confused by cars that may also be passing over the sensors. There is a particularly interesting method in Minneapolis called Zap. The product uses an RFID sensor the user attaches to Figure 3. Example of raw Nice Ride records your bicycle. When a bicycle passes by (dependent variable source). Zap sensors, located throughout the city, The 2016 dataset from Nice Ride the sensor will count the bike as having contained over 1 million rider trips. These ridden past the checkpoint. Dero, the data formed the backbone of the company producing Zap, partnered with dependent variables. These individual trips other businesses to provide the additional can be consolidated into ridership totals at incentive of drawings and business each station and then subtotals can be discounts throughout the city to those extracted based upon numerous factors, users who have passed the sensors most such as time of day, member vs casual often. ridership, etc. Each subset represents The most complete and accessible potential differences in ridership. For open source dataset is the data available example, the people riding Nice Rides at from Nice Ride Minnesota and other bike 9AM may have different habits and shares throughout the country and the routines than those who are riding at world. Nice Ride data was chosen to 1:45PM. There is likely more of a chance answer the question: What are the factors that the 9AM rider is commuting to work, 3 while perhaps there is a higher chance that to bicycles using a special code or key. the afternoon rider may be riding to class, These memberships usually indicate that or home from school. Extracting subsets of these are frequent riders and the the data allows the potential for the hypothesis would be that they would have statistical analysis of those factors. more consistent patterns in their riding. Following are the subsets of rider The “casual” rider type purchases a rental trips extracted from the ridership dataset. on an hourly or daily basis. They typically Each dependent variable dataset was do not ride as frequently, and judging by placed into a single Esri geodatabase the locations and timestamps of these awaiting conflation of data from the users, more of these trips appear to be independent variables listed later in the recreational trips (e.g., a trip that both study. begins and ends at the Lake Calhoun station from 7 to 8 pm). Given the frequent Dependent Variables use of bicycles by members, as compared to the casual user, this is more likely a Time of Day dataset with a higher subset of commuters, who have more predictable patterns than Time of day could be a significant the casual rider.
Details
-
File Typepdf
-
Upload Time-
-
Content LanguagesEnglish
-
Upload UserAnonymous/Not logged-in
-
File Pages12 Page
-
File Size-