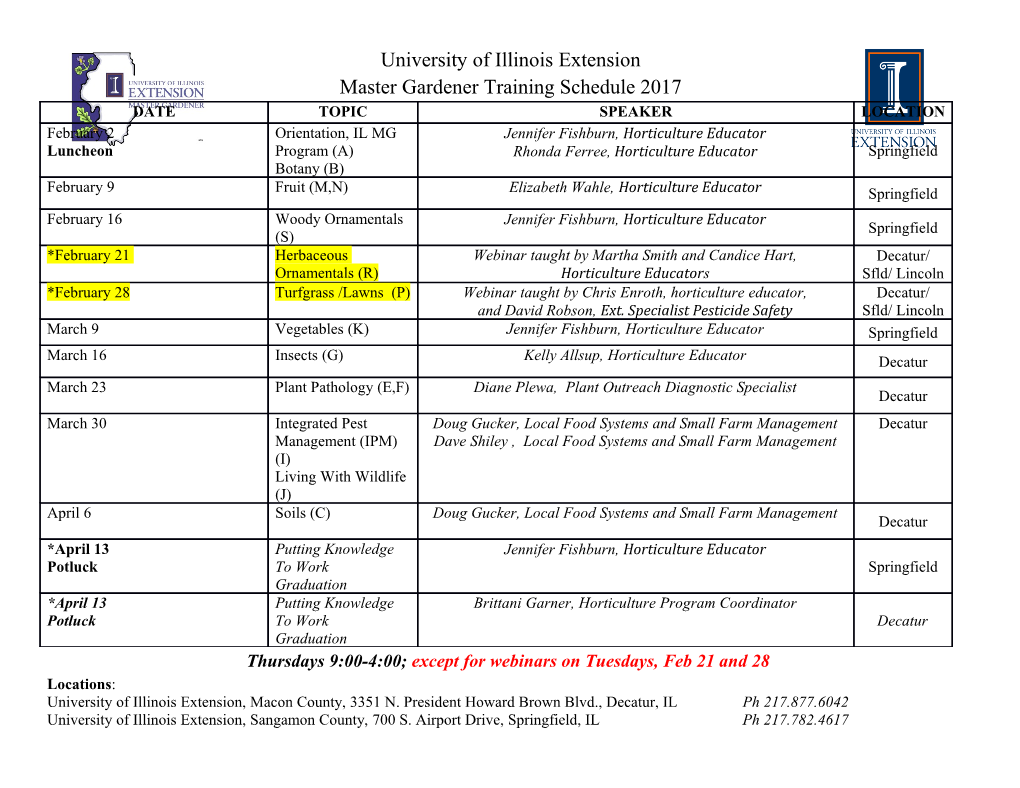
Shot Quality Model A logistic regression approach to assessing NHL shots on goal Ken Krzywicki – January 2005 Abstract The concept of examining NHL shot quality is not new; both Alan Ryder and Graeme Johns have published papers on the subject1. Due to data limitations at the time of their papers, defense was examined team-wide; individual level data was available only for offense, whereas goalie data was not. Ryder’s paper focused mainly on team defense and Johns wrote mainly about offense. Recently, data assigning each goaltender to the shot against became available. While mentioning the offensive side of the game, this paper will center on individual goalie (and some team level observations) defense. The conclusions reached here are very similar to those arrived at by both Johns and Ryder. In order to assess NHL shot quality, data was collected from the 2003-04 regular season. A logistic regression model was built to predict the probability of a goal with four predictor variables—distance, shot type, rebound and situation. One minus this probability is the predicted probability of a save. The model fit the data rather well, with a c-statistic value of 0.733, and had a Kolmogorov-Smirnov (KS) statistic2 of 35.05. The model was validated against the 2002-03 shot data published by Alan Ryder. This data does not contain all 1,230 games, but has roughly 90% and is sufficient for an out- of-time model validation. KS, population stability and characteristics analyses were used to validate the model to this data. For a given team, the predicted shooting percentages were compared to that of the average predicted as well as actual. Those above the league’s average predicted value were taking higher quality shots, while those below lower. Comparisons to actual shooting percentage were also made. For the flip side of the coin, the predicted save percentages for each goaltender were compared to that of the average predicted value. Those falling below were said to have faced “tougher” shots, those above “easier” shots and those at the mean, “average” shots. One could make inferences from this classification. The predicted save 1 See Shot Quality – A Method for the Study of the Quality of a Hockey Team’s Shots Allowed [Ryder, January 2004] and Statistical Shot Quality Weighting – An Investigation of NHL Shot Quality and Offensive Performance [Johns, October 2004] 2 See Glossary for definition of KS. Shot Quality Model Ken Krzywicki – January 2005 1 percentages were also compared to actual save percentages and indexed to see who outperformed predictions and vice-versa. This also speaks to which teams faced higher percentage (i.e., higher quality) shots versus those that did not. Perhaps some teams played differently in front of their different goalies. This paper attempts to address these issues. Data The data used for the model was collected from the NHL play-by-play (PBP) files as well as game summary (GS) files—both available at www.nhl.com. A few PBP files stopped short of recording the entire game; the data does not tie out to the year-end statistics published by the NHL, but is very close and any differences are statistically immaterial. The PBP files were generated by the Real Time Scoring System (RTSS), of which much has already been written. These files provide some information on each of the “events” occurring throughout a game and the shots on goal data was used for this study. Goals Saves Total Shot Type # Dist Int Rate # Dist Int Rate # Dist Backhand 567 9.0% 11.2% 4,484 7.2% 88.8% 5,051 7.3% Slap 1,645 26.0% 6.4% 23,969 38.2% 93.6% 25,614 37.1% Snap 988 15.6% 9.7% 9,185 14.7% 90.3% 10,173 14.7% Tip-In 755 12.0% 21.4% 2,770 4.4% 78.6% 3,525 5.1% Wrap 54 0.9% 5.4% 941 1.5% 94.6% 995 1.4% Wrist 2,308 36.5% 9.8% 21,328 34.0% 90.2% 23,636 34.3% Total 6,317 100.0% 9.2% 62,677 100.0% 90.8% 68,994 100.0% Table 1: Shot Type As shown in Table 1 above, slap shots were employed the most, 37.1% of the time, with a success rate of 6.4% (accounting for 26.0% of all goals). Methodology A binary target variable called GOAL was created with a value of 1 for a goal and 0 for a save. The data was then randomly split 75%/25% for model training, or development, and validation. A logistic regression model was constructed on the 75% training sample and validated on the 25% holdout portion of the data. This was done to ensure the model does not over fit the data. The model was subsequently validated against an out- of-time dataset, namely that from the 2002-03 regular season. Johns also observed that Shot Quality Model Ken Krzywicki – January 2005 2 a logistic function fit the shot distance. The model herein considered several variables for inclusion: The variables considered for the model included: • Distance (measured in feet) • Shot Type • Situation • Period • Penalty Shot • Rebound • Scramble Shot • Long Shot • Normal Shot Rebound, Scramble, Long and Normal shots were defined per Alan Ryder’s paper3. True interaction terms were not considered here, but are inherent in the model since several variables are working simultaneously. Model Results Four variables (plus an intercept) remained in the final model—distance, shot type, rebound and situation; the others were either too correlated to these or insignificant. 3 See Glossary for definitions. Shot Quality Model Ken Krzywicki – January 2005 3 V a ria b le R a n g e P o in ts In te rc e p t A d d to a ll re c o rd s -2 .2 3 6 9 D is ta n c e L e s s th a n 1 0 ft 0 .6 8 8 4 1 0 - 1 2 ft 0 .6 3 7 4 1 3 - 1 4 ft 0 .5 5 6 4 1 5 - 1 6 ft 0 .5 1 7 4 1 7 - 2 2 ft 0 .3 6 5 4 2 3 - 3 1 ft 0 .0 0 0 0 3 2 - 3 6 ft -0 .3 8 0 5 3 7 - 3 8 ft -0 .4 7 5 8 3 9 - 4 4 ft -0 .8 1 5 5 4 5 - 5 7 ft -1 .0 8 4 8 5 8 ft o r m o re -1 .3 8 2 4 S h o t T yp e W ra p -0 .0 7 4 2 S la p -0 .0 5 7 3 W ris t 0 .0 0 9 3 S n a p 0 .0 1 3 0 B a c k h a n d 0 .0 3 6 1 T ip -In 0 .1 4 8 7 R e b o u n d Y e s 1 .3 3 6 2 N o 0 .0 0 0 0 S itu a tio n E V -0 .1 2 4 4 S H 0 .0 3 9 9 P P 0 .4 0 0 7 Table 2: Model Scorecard The model score, or predicted probability of a goal, is defined using Table 2 as follows: 1 P(GOAL) = . −points 1+ e The c-statistic for the model was 0.733. The c-statistic is the probability that an observation with an event (goal, in our case) has a higher predicted probability than an observation without an event. Values less than 0.50 mean the model does no better than randomly assigning a goal to a particular observation. The KS statistic was used to validate the model. When comparing the training and validation datasets, we wish to see a small difference in KS values. Table 3 below illustrates this and we can safely say the model does not over fit (35.09 compared to 34.91). Shot Quality Model Ken Krzywicki – January 2005 4 KS Report for Training Sample Pred Probability (%) Totals Cuml % Saves Int Rate Cuml % Goals Int Rate Cuml % KS 17.05 - 100.00 5,267 100.00% 3,912 74.27% 100.00% 1,355 25.73% 100.00% 0.00 14.22 - 17.04 5,207 89.81% 4,372 83.96% 91.67% 835 16.04% 71.20% 20.47 12.07 - 14.21 4,757 79.74% 4,156 87.37% 82.37% 601 12.63% 53.45% 28.92 8.70 - 12.06 5,185 70.54% 4,617 89.05% 73.53% 568 10.95% 40.68% 32.85 6.13 - 8.69 5,523 60.51% 5,076 91.91% 63.70% 447 8.09% 28.61% 35.09 4.85 - 6.12 4,158 49.82% 3,943 94.83% 52.90% 215 5.17% 19.11% 33.79 3.76 - 4.84 6,398 41.78% 6,118 95.62% 44.51% 280 4.38% 14.54% 29.97 2.93 - 3.75 4,560 29.40% 4,446 97.50% 31.49% 114 2.50% 8.59% 22.90 2.35 - 2.92 5,284 20.58% 5,106 96.63% 22.02% 178 3.37% 6.16% 15.86 0.00 - 2.34 5,355 10.36% 5,243 97.91% 11.16% 112 2.09% 2.38% 8.78 Total 51,694 46,989 90.90% 4,705 9.10% 35.09 KS Report for Validation Sample Pred Probability (%) Totals Cuml % Saves Int Rate Cuml % Goals Int Rate Cuml % KS 17.16 - 100.00 1,641 100.00% 1,218 74.22% 100.00% 423 25.78% 100.00% 0.00 14.25 - 17.15 1,709 90.51% 1,441 84.32% 92.24% 268 15.68% 73.76% 18.48 12.07 - 14.24 1,796 80.64% 1,535 85.47% 83.05% 261 14.53% 57.13% 25.92 8.70 - 12.06 1,709 70.25% 1,512 88.47% 73.27% 197 11.53% 40.94% 32.32 6.13 - 8.69 1,870 60.38% 1,723 92.14% 63.63% 147 7.86% 28.72% 34.91 4.85 - 6.12 1,338 49.57% 1,256 93.87% 52.65% 82 6.13% 19.60% 33.04 3.76 - 4.84 2,136 41.83% 2,023 94.71% 44.64% 113 5.29% 14.52% 30.12 2.93 - 3.75 1,588 29.49% 1,551 97.67% 31.74% 37 2.33% 7.51% 24.24 2.35 - 2.92 1,781 20.31% 1,728 97.02% 21.86% 53 2.98% 5.21% 16.65 0.00 - 2.34 1,732 10.01% 1,701 98.21% 10.84% 31 1.79% 1.92% 8.92 Total 17,300 15,688 90.68% 1,612 9.32% 34.91 Table 3: KS Reports for Training and Validation Samples The overall score, or predicted probability, distribution of the model is as follows: KS Report for Entire Dataset Pred Probability (%) Totals Cuml % Saves Int Rate Cuml % Goals Int Rate Cuml % KS 17.05 - 100.00 7,092 100.00% 5,278 74.42% 100.00% 1,814 25.58% 100.00% 0.00 14.22 - 17.04 6,958 89.72% 5,852 84.10% 91.58% 1,106 15.90% 71.28% 20.30 12.07 - 14.21 6,327 79.64% 5,504 86.99% 82.24% 823 13.01% 53.78% 28.47 8.70 - 12.06 6,894 70.47% 6,129 88.90% 73.46% 765 11.10% 40.75% 32.71 6.13 - 8.69 7,393 60.47% 6,799 91.97% 63.68% 594 8.03% 28.64% 35.05 4.85 - 6.12 5,496 49.76% 5,199 94.60% 52.83% 297 5.40% 19.23% 33.60 3.76 - 4.84 8,534 41.79% 8,141 95.39% 44.54% 393 4.61% 14.53% 30.01 2.93 - 3.75 6,148 29.42% 5,997 97.54% 31.55% 151 2.46% 8.31% 23.24 2.35 - 2.92 7,065 20.51% 6,834 96.73% 21.98% 231 3.27% 5.92% 16.06 0.00 - 2.34 7,087 10.27% 6,944 97.98% 11.08% 143 2.02% 2.26% 8.82 Total 68,994 62,677 90.84% 6,317 9.16% 35.05 Table 4: KS Report for Entire Dataset Another method of assessing the model is a fit chart.
Details
-
File Typepdf
-
Upload Time-
-
Content LanguagesEnglish
-
Upload UserAnonymous/Not logged-in
-
File Pages20 Page
-
File Size-