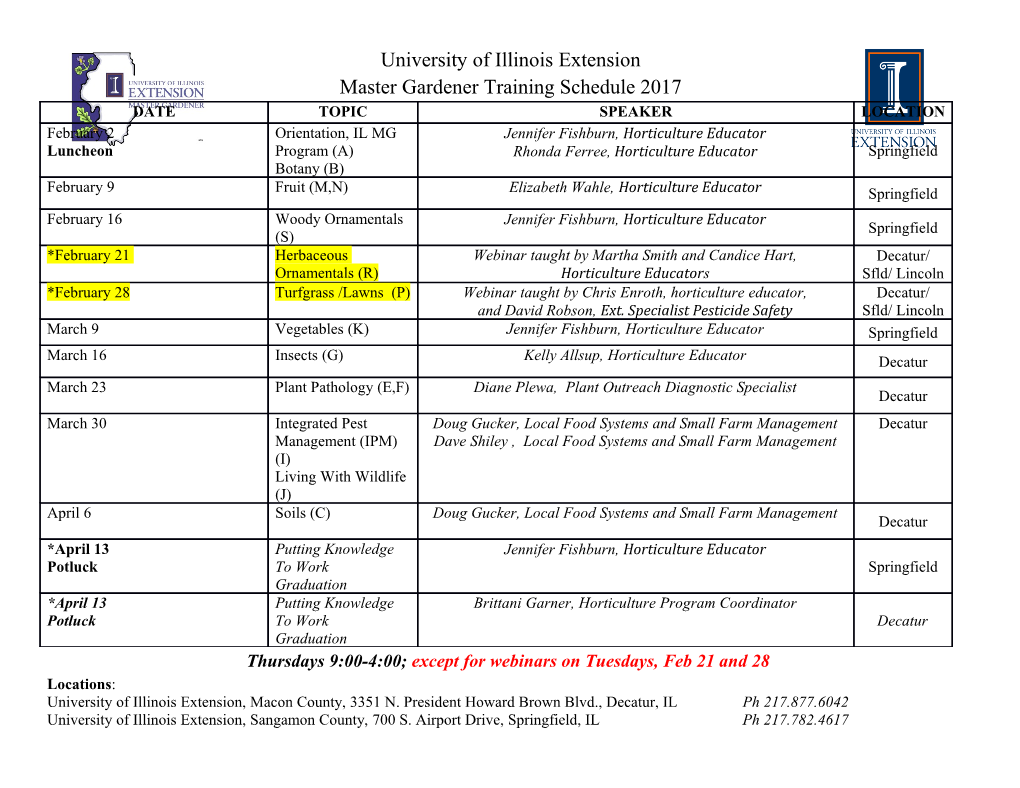
國國國ËËË???»»»'''xxxÇÇÇ訊訊訊科科科xxxûûû Department of Computer Science National Chengchi University ©ë論文 Master’s Thesis 治 政 大 立 學 以使(者音樂國 F}記錄¼音樂L®¨¦K研 v ‧ ‧ N a y Learning User Music Listening Logst for Music t i i s o r Playlistn Recommendatione a i v l C n hengchi U 研 v 生:J淳/ 指導Y授:!銘峰 -華民國 一~零七 t 二 月 February 2018 107 © ë 論 文 以 使 ( 者 音 樂 F } 記 政 治 錄 大 ¼ 音 立 樂 學 L ® 國 ¨ ¦ ‧ K ‧ 研 N v a y t t i i s o r n e a v l i Ch Un ? engchi » ' x Ç 訊 科 x û J 淳 / 以使(者音樂F}記錄¼音樂L®¨¦K研v Learning User Music Listening Logs for Music Playlist Recommendation 研 v 生:J淳/ Student:Chun-Yao Yang 指導Y授:!銘峰 Advisor:Ming-Feng Tsai 國國國ËËË???»»»'''xxx ÇÇÇ訊訊訊科科科xxxûûû 治 政©ë論文 大 立 學 國 A Thesis ‧ submitted‧ to Department of Computer Science N aNational Chengchi University y t t i in partiali fulfillment of the Requirementss o r n e a for the degree of i v l C n heMasterngchi U in Computer Science -華民國 一~零七 t 二 月 February 2018 治 政 大 立 學 國 ‧ ‧ N a y t t i i s o r n e a i v l C n hengchi U 2 致致致謝謝謝 很®x(?'1x期間能跟¨銘峰老+2L研v, (與老+x 習的N程-=能感受0老+(xS研v上的±ñ,以Ê(對OL、 0節上的9NU理K¦, 能有這個_會從Áx習&(hË研v上¥ 受指導,M有我Ê)這,的研v成果。 d外,能(老+的¨¦下與 KKBOX 研v團隊合\,對我政而言/個治A³的¯定。 向志明xw一同 研vx習的N程-,讓我對音樂"m有更ñe大的­ã,&從-r取了 將_hx習應((¨¦立領域á的經W。 從mL、xS不同角r上»é 視ãz¦法,這#的觀點切換經Wk©我(思考上更學H'且實際。 最 後F_同#Í要且讓º開Ã的/,(與銘峰老+、釧9老+、志明x w和致¤的q同ª力國 下,我們的研v順)0( 2016 t¼ ACM RecSys 上和'¶分«。 感謝這一切得來不易的_會,讓這些從*想Ï的事 ‧ Å,一個個美}的發生(生;á。 能有這#的經‧ w,感謝6Í(背後h力的/持我@Z的z定,讓我 N (?'的\謐環境下,更加Ã!Á騖0»x習。 _謝謝實W¤*秀的 a y %4們,嘉P、至I、致¤、²文一同(研v上n%和St #,讓這條 t i dh的路p的更加i 暖Ã。 (d要<外感謝²文和Á惠,s d了(研v上 o r 的ø互%*外,(ún北的生;x}有³們的指引,使e我(外0Bx的 N程更加順B。 而研va 最後階段的ñ文撰ë上,謝謝v Jay 和 Peter 從Á l i f予Ð點,k©我最後能TC成這#的嘗f與挑0n, ddK外,我_很 h U «受與`們¤A的N程, 因ºe背on上g的c不h同i ,讓我x習從ê«領域的 觀點-½ú,Í覆省思&嘗f(不同的¹式»ãËêñ的研v, _感 謝`們尊Í、耐Ã0F},&從各ê的專m領域-f予這Ç研v寶´ 的看法。 謝謝(º工zg領域n%的同Á們@S下的ú石, 讓我能(_h x習的研v-, 透N直º0^比思考»激|不同的x習想法,&從- ~尋有意思的¹式»研vOL, x習(ê«的經w»尋思êñ/透N 怎#的!式和外(環境¥ø,而外L的訊o:激È/如U9變êñ的 LºVe, 我喜a透N這#的切«經w»ø^Á通。 最後期1êñ能 精2x習,K(ûq、科x´9的角¦»ãËê6L-多變的#貌, »­ã這世L, &將@領e的Ã得應((>會上&分«這份喜悅f« Á和喜}的º。 JJJ淳淳淳/// 國Ë?»'xÇ訊科xû February 2018 3 以使(者音樂F}記錄¼音樂L®¨¦K研v ---文文文XXX要要要 音樂L®/1一D多首不同C 、¨<的音樂@D成的,它包+ 了è/者的個ºÁs以Ê因應;L、目的'"生而成。 我們可以透N 樂曲的律動、節O、L曲的;L精^,2而è/一個ø應Q合的û列 L曲。 vÊ的音樂6}市4;要/(²路2As台上2L¨B、¨0 的F},;要的s台有 Spotify、Apple Music 以Ê KKBOX。 各¶m者 不®ê/Ð供使(者L曲的搜"、®曲的F},更Ð供訂±專mL® è/者的L®訂±服務,甚至/讓一,的使(者Ã與L®ê訂è/的 N程。 6而如U(有P的B間g針對使(者的F}習c»Ë9s台上 P富的音樂Ç源/個很'的挑0。 上述的N程我們1Kº¨¦,而v M的音樂¨¦研v'多/(對使(者2治Lø關L曲的¨¦,®少能2 一e(更½ad!上的L®上政2L¨¦。 這邊大我們1d一¨¦應(Ð 供Le式向Ïh:法x立習!型,(有W使(者、L曲、L®的pê' >¤²路上,對使(者2LL®的¨¦。 º了能有H的x習úL®¨ ¦的!型,我們更將使(者、L®和L曲的pê'圖學bÍD成二分圖 (bipartite graph),國 &(d圖b的邊上賦予不I的權Í,d一權Í/ ú¼使(者±式ÍKr得的。 ¥W再透N¨_+e(random walk), 9據邊上的權<2L路徑的½#x取,最後再將路徑上‧ 經N的節點2 LLe式向Ïh:法的x習。 我們使(P~里·距離計算各節點h ‧ :法的0近關ÂN ,再將與使(者較ºø關的L®¨¦f使(者。 實W I的è 我a 蒐 t 的Ç料2L 訓y 練 2L W 分, 們 Æ KKBOX i 份 !型 t & ¨ ¦,&將¨¦的Pt 果與使(者@喜愛的L®2L準º¦i (Precision) io s U0, P果I實@得n0的¨¦H果較一,±門L®的¨r ¦來的},且 更 化的L ¦ e º w個º ®¨a 。 i v l C n hengchi U 4 Learning User Music Listening Logs for Music Playlist Recommendation Abstract Music playlist is crafted with a series of songs, in which the playlist cre- ator has controlled over the vibe, tempo, theme, and all the ebbs and flows that come within the playlist. To provide a personalization service to users and discover suitable playlists among lots of data, we need an effective way to achieve this goal. In this paper, we modify a representation learning method for learning the representation of a playlist of songs, and then use the repre- sentation for recommending playlists政 to users.治 While there have been some well-known methods that can model the preference大 between users and songs, little has been done in立 the literature to recommend music playlists. In light of this, we apply DeepWalk, LINE and HPE to a user-song-playlist學 network. To better encode the國 network structure, we separate user, song, and playlist nodes into two different sets, which are grouped by the user and playlist set ‧ and song as the other one. In the bipartite graph, the user and playlist node ‧ are connected toN their joint songs. By adopting random walks on the con- a y structed graph, we can embed users and playlists via the commont informa- t i tion between each other.io Therefore, users can discover their favorites playlists n r through the learned representations.a After the embeddinge process, we then l i v use the learned representations C to perform playlist recommendationn task. Ex- h U periments conducted on a real-worlden datasetgch showedi that these embedding methods have a better performance than the popularity baseline. In addition, the embedding method learns the informative representations and brings out the personal recommendation results. 5 治 政 大 立 學 國 ‧ ‧ N a y t t i i s o r n e a i v l C n hengchi U 6 Contents 致致致謝謝謝 3 ---文文文XXX要要要 4 Abstract 5 治 1 Introduction政 大 1 2 Related Work立 5 2.1 Word Embedding . .學 . .5 2.2 Social Network Representation . .6 2.3 Preserving Network國 Structure . .6 ‧ 3 Methodology 9 3.1 Music Dataset‧ and Creating the Bipartite Graph . .9 3.2 DeepWalk .N . 11 a y 3.3 Large-Scale Information Network Embedding . .t . 12 t i 3.4 Heterogeneous Preferencei Embedding . .s . 13 o r n e 4 Experimental Resultsa v 17 l i 4.1 Experimental Settings .C . .h . .U .n . 17 4.1.1 Dataset and Ground Trutheng . .c .h .i . 17 4.1.2 Similarity Calculation . 18 4.1.3 Evaluation Metrics . 19 4.2 Experimental Results . 20 4.2.1 DeepWalk . 20 4.2.2 LINE . 21 4.2.3 HPE . 22 4.3 Case Study . 23 5 Conclusions 29 Bibliography 31 7 治 政 大 立 學 國 ‧ ‧ N a y t t i i s o r n e a i v l C n hengchi U 8 List of Figures 3.1 Music social network . 10 3.2 The bipartite graph for preference embedding . 11 3.3 Extract a random walk from social network with the specified window size and walking steps . .治 . 12 3.4 An example of first-order and政 second-order proximity大 . 13 3.5 Neural network for learning立 social network representations . 14 3.6 Neural network architecture . .學 . 15 4.1 Playlist: A collection國 of songs . 18 4.2 Comparison between DeepWalk, LINE and HPE on precision‧ rate . 22 4.3 UserA listening‧ behavior . 24 N 4.4 UserB listening behavior . 26 a y t t i i s o r n e a i v l C n hengchi U 9 治 政 大 立 學 國 ‧ ‧ N a y t t i i s o r n e a i v l C n hengchi U 10 List of Tables 4.1 Dataset statistics . 17 4.2 Genre statistics . 17 4.3 DeepWalk parameters definition . 20 4.4 Precision at 20 of DeepWalk with different治 parameter settings . 20 4.5 Precision at 20 of LINE with政 different parameter大 settings . 21 4.6 Precision at 20 of HPE立 with different parameters . 23 4.7 MAP at 20 with different embedding methods . .學 . 23 4.8 User listening statistic國 . 24 4.9 UserA label reference . 25 ‧ 4.10 Playlist: Recommendation playlist . 25 ‧ 4.11 UserB label referenceN . 26 a y 4.12 User performance . .t . 27 t i i s o r n e a i v l C n hengchi U 11 治 政 大 立 學 國 ‧ ‧ N a y t t i i s o r n e a i v l C n hengchi U 12 Chapter 1 Introduction Music streaming services provide various ways治 for users to explore music they like, such as through creating and sharing music政 playlists. To deliver大 songs to users more efficiently, lots of music platforms deploy立 the recommender system to achieve this goal. For music recommendations, the explicit method for recording users’ tastes學 is to let users input their preferences, such as through國 liked or disliked songs. Afterwards, we can apply well known recommendation techniques like content-based filtering or collaborative filtering ‧ [1, 7] approach to predict what a user may enjoy. In current literature, much has been stud- ‧ ied about how to modelN the preference of users and songs for an effective recommender a y system [6, 13, 14]. However, little has been studied how to recommendt a combined set t i of items (i.e., playlists) basedio on user preference logs on individuals items (i.e., songs). n r Therefore, when we want toa recommend playlists to the usere without any pre-existing l i v user preference toward playlist, the C above conventional methodsn obviously become inad- h U equate. In light of this, we proposed to useen Heterogeneousgchi Preference Embedding (HPE) approach [3] based on their past listening songs and construct a user-song-playlist bipar- tite graph to recommend music playlists.
Details
-
File Typepdf
-
Upload Time-
-
Content LanguagesEnglish
-
Upload UserAnonymous/Not logged-in
-
File Pages46 Page
-
File Size-