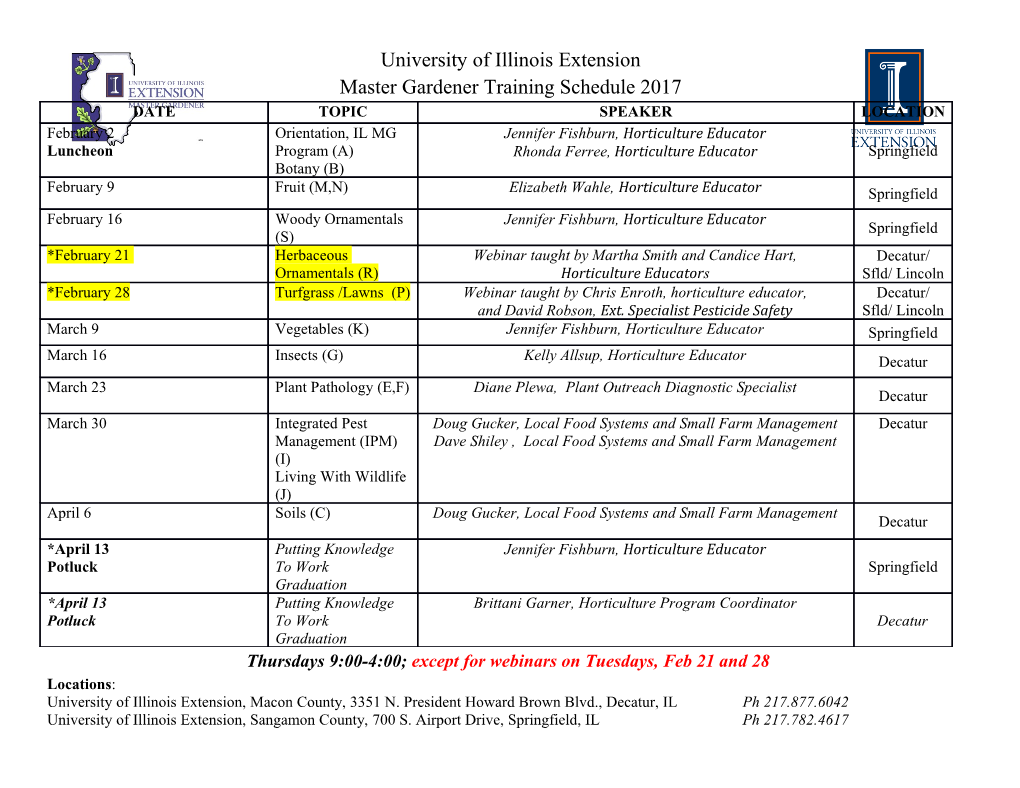
162 MONTHLY WEATHER REVIEW VOLUME 138 Wavelet Analysis on the Variability, Teleconnectivity, and Predictability of the Seasonal Rainfall of Taiwan CHUN-CHAO KUO Department of Hydraulic and Ocean Engineering, National Cheng Kung University, Tainan, Taiwan, and Department of Civil and Environmental Engineering, University of Alberta, Edmonton, Alberta, Canada THIAN YEW GAN Department of Civil and Environmental Engineering, University of Alberta, Edmonton, Alberta, Canada PAO-SHAN YU Department of Hydraulic and Ocean Engineering, National Cheng Kung University, Tainan, Taiwan (Manuscript received 10 July 2008, in final form 5 July 2009) ABSTRACT Using wavelet analysis, the variability and oscillations of November–January (NDJ) and January–March (JFM) rainfall (1974–2006) of Taiwan and seasonal sea surface temperature (SST) of the Pacific Ocean were analyzed. From the scale-average wavelet power (SAWP) computed for the seasonal rainfall, it seems that the data exhibit interannual oscillations at a 2–4-yr period. On the basis of correlation fields between decadal component removed wavelet PC (DCR-WPC1) of seasonal rainfall and decadal component removed scale- averaged wavelet power (DCR-SAWP) of SST of Pacific Ocean at one-season lead time, SST of some do- mains of the western Pacific Ocean (July–September SST around 08–308N, 1208–1608E; October–December SST around 08–608N, 1258E–1608W) were selected as predictors to predict seasonal NDJ and JFM rainfall of Taiwan at one-season lead time, respectively, using an Artificial Neural Network calibrated by the Genetic Algorithm (ANN-GA). The ANN-GA was first calibrated using the 1975–99 data and independently vali- dated using 2000–06 data. In terms of summary statistics such as the correlation coefficient, root-mean-square error (RMSE), and Hanssen–Kuipers (HK) scores, the prediction of seasonal rainfall of northern and western Taiwan using ANN-GA are generally good for both calibration and validation stages, but not so for south- eastern Taiwan because the seasonal rainfall of the former are much more significantly correlated to the SST of selected sectors of the Pacific Ocean than the latter. 1. Introduction tends to spill downstream, causing water shortages if sub- sequent seasons happen to be dry. In Taiwan, dry seasons For Taiwan and East Asia, there have been various (usually November–April in Taiwan except in the north- studies focused on their summer rainfall prediction eastern Taiwan) on the average received about 32% of (Chan and Shi 1999; Chien et al. 2002; Chien and Jou annual rainfall (Water Resources Agency 2008). This 2004; Shiao and Juang 2006; Li and Zeng 2008) but sel- usually posts problems to water resources management dom studies on its winter and spring rainfall prediction. of Taiwan, especially its agriculture sector, which has Summer, as the wet season, tends to receive more rainfall about 70% of consumptive use. Therefore, dependable than other seasons of an average year in Taiwan. How- seasonal prediction of rainfall for the dry season will be ever, the capacity of many reservoirs in Taiwan is general useful to Taiwan’s water resources management. limited due to the steep terrain so that excessive water Past studies provide an overview of the teleconnec- tion patterns of East Asia with respect to large-scale climate phenomena such as El Nin˜ o–Southern Oscil- Corresponding author address: Thian Yew Gan, Department of Civil and Environmental Engineering, University of Alberta, lation (ENSO; e.g., Xu et al. 2004; Yang and Lau 2004), Edmonton, AB T6G 2W2, Canada. but different teleconnection patterns exist in East Asia E-mail: [email protected] (Li et al. 2005), so it should be useful to investigate the DOI: 10.1175/2009MWR2718.1 Ó 2010 American Meteorological Society Unauthenticated | Downloaded 10/02/21 02:46 AM UTC JANUARY 2010 K U O E T A L . 163 connectivity between local areas and large-scale cli- tivation of paddy field begins in January and so decisions mate phenomena, especially for a small area such as on water release schedule are usually done at the begin- Taiwan. Besides ENSO, some past studies also link ning of the farming season (January–February). Besides precipitation of Taiwan to large-scale natural variabil- the effect of SST, the spring rainfall of Taiwan starting in ity of sea surface temperature (SST) and Pacific decadal April is subjected to more atmospheric dynamics and cir- oscillation (PDO; Lu and May 2003; Chen et al. 2003; culation and so it will be more challenging to predict the Jiang et al. 2003; Hung et al. 2004). Three precipitation spring rainfall using the Artificial Neural Network cali- associations for Taiwan, Guam, and northwest Australia brated by the Genetic Algorithm (ANN-GA) approach. from January to March have been identified by Lu and First, the spatial, temporal, and frequency variability May (2003). The first type gives rise to wet conditions of the dominant oscillations of the rainfall fields of over Taiwan but dry conditions over the Philippine Sea Taiwan using wavelet analysis and Principal Compo- and northwest Australia, and vice versa by the second nent Analysis (PCA) are examined. Then, sectors of type, but under the third type both Taiwan and north- seasonal SST in the Pacific Ocean that are strongly west Australia will be dry but the Philippine Sea will be correlated to the seasonal rainfall (NDJ and JFM) are wet. Jiang et al. (2003) found that spring (February– identified. Third, the wavelet coherence between selected March) heavy rainfall events were positively correlated SST, ENSO indices, and seasonal rainfall are examined. with the cold season (November–March) Nin˜ o-3 SST Fourth, an ANN-GA was calibrated using the seasonal significantly since the late 1970s but not much correla- rainfall and SST data and the calibrated model is vali- tion between the early 1950s and the late 1970s. The dated by assessing the accuracy of the predicted seasonal spring (February–April) rainfall in northern Taiwan was rainfall based on data not used in the calibration experi- also found to fluctuate simultaneously with the PDO ence. Finally, a composite analysis was also applied to since the early twentieth century (Hung et al. 2004), understand how the warm and cold SST episodes affect and positive (negative) PDO tends to be associated with the precipitation patterns near Taiwan. more (less) spring rainfall in northern Taiwan. These studies show some connectivity between the 3. Climate data rainfall of Taiwan and climate anomalies or SST, which probably implies that predicting seasonal rainfall by such Rainfall data from 15 stations of Taiwan’s Central relationships should be feasible. To our best knowledge, Weather Bureau (Fig. 1) collected from 1974 to 2006 was the prediction of rainfall for winter or spring for Taiwan used to evaluate the predictability of 3-month rainfall by the proposed approach has yet been attempted, but (NDJ and JFM) in Taiwan. The Kaplan SST V2 data summer rainfall prediction in Taiwan has been done by (Kaplan et al. 1998; Parker et al. 1994; Reynolds and Huang and Hsu (2005). Through a permutation test, they Smith 1994) of the same period was provided by the identified sectors of the Pacific Ocean where SST is re- National Oceanic and Atmospheric Administration/Of- lated to the summer rainfall of Taiwan. These sectors of fice of Oceanic and Atmospheric Research/Earth System the Pacific Ocean are close to or located within the win- Research Laboratory Physical Sciences Division (NOAA/ dow of the tropical Pacific used to compute the Nin˜o-3 OAR/ESRL PSD), Boulder, Colorado, from their Web index. Selected principal components (PCs) on these site (http://www.cdc.noaa.gov/). The monthly SST anom- sectors of SST data are used as predictors to an Auto- aly at 58358 resolution were selected from the Pacific regressive Integrated Moving Average (ARIMA) and Ocean window (258S–608N, 1208E–708W) and averaged to regression model to predict the summer rainfall of 3-month values, namely, JFM, AMJ, JAS, and OND. The Taiwan. They found that the regression model performed geopotential height and wind fields (Kalnay et al. 1996) better than the ARIMA model. are prepared by the National Centers for Environmental Prediction–National Center for Atmospheric Research (NCEP–NCAR) as a 40-yr reanalysis product and also 2. Research objectives made available online at NOAA/OAR/ESRL PSD’s Web The primary objectives of this study are to analyze site (http://www.cdc.noaa.gov/). These data have a hori- the characteristics of seasonal rainfall of Taiwan, and zontal resolution of 2.5832.58. to predict the seasonal, November–January (NDJ) and January–March (JFM; hereafter all three-month periods 4. Research methodology will be referred to by the first letter of each respective a. Wavelet analysis month) rainfall of Taiwan, which will be useful for de- cision makings in water resources management because The wavelet transform of a real signal X(t), used to November is the beginning of the dry season and the cul- extract time and frequency domain information from Unauthenticated | Downloaded 10/02/21 02:46 AM UTC 164 MONTHLY WEATHER REVIEW VOLUME 138 where Cd is 0.776 for the Morlet wavelet (used in this paper), dj is the scale interval, which is 0.0833. Using smaller values of dj gives finer resolutions in scales. dt is the sampling interval which is 1 in this paper, and j1 to j2 (e.g., j1 is 2 yr while j2 is 4 yr) represent scales over which SAWP is computed. For the choice of wavelet function, we should choose a wavelet function that can effectively reflect the oscillatory features of a time series, which in our case is the rainfall. A broad (in time) wavelet function will have poor time but good frequency reso- lutions, and vice versa.
Details
-
File Typepdf
-
Upload Time-
-
Content LanguagesEnglish
-
Upload UserAnonymous/Not logged-in
-
File Pages14 Page
-
File Size-