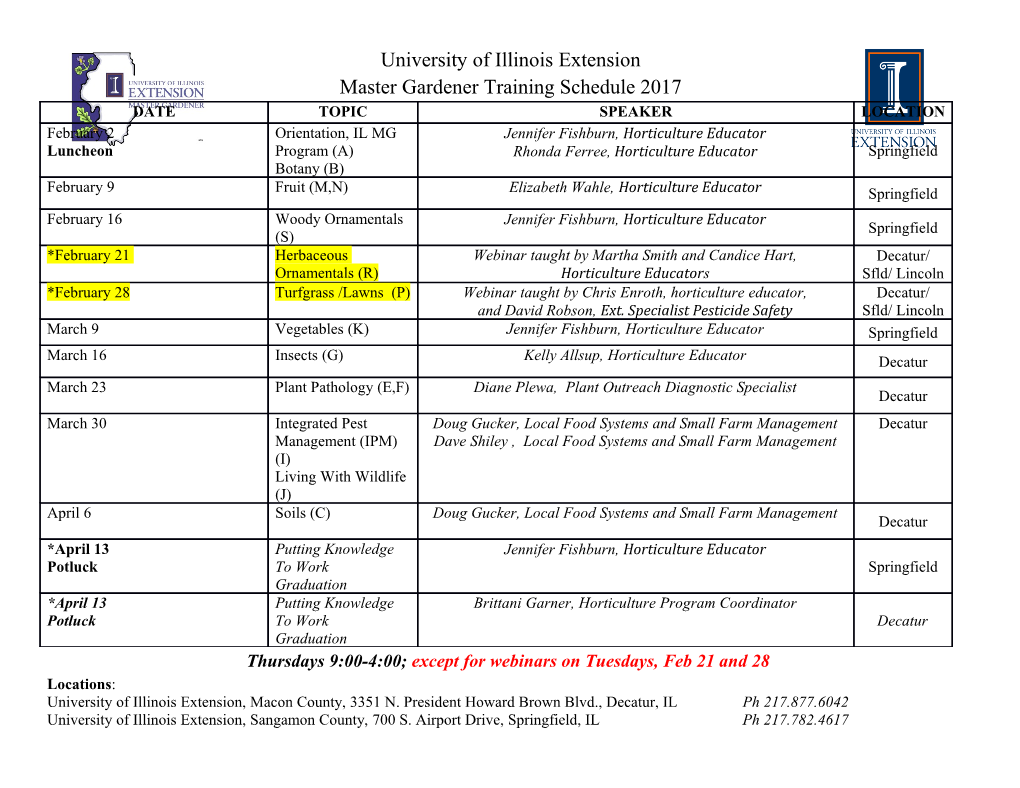
International Journal of Environmental Research and Public Health Article Importance of Geospatial Heterogeneity in Chronic Disease Burden for Policy Planning in an Urban Setting Using a Case Study of Singapore Ken Wei Tan 1, Joel R. Koo 1, Jue Tao Lim 1, Alex R. Cook 1 and Borame L. Dickens 1,* Saw Swee Hock School of Public Health, National University of Singapore, National University Health System, Singapore 117549, Singapore; [email protected] (K.W.T.); [email protected] (J.R.K.); [email protected] (J.T.L.); [email protected] (A.R.C.) * Correspondence: [email protected] Abstract: Chronic disease burdens continue to rise in highly dense urban environments where clustering of type II diabetes mellitus, acute myocardial infarction, stroke, or any combination of these three conditions is occurring. Many individuals suffering from these conditions will require longer-term care and access to clinics which specialize in managing their illness. With Singapore as a case study, we utilized census data in an agent-modeling approach at an individual level to estimate prevalence in 2020 and found high-risk clusters with >14,000 type II diabetes mellitus cases and 2000–2500 estimated stroke cases. For comorbidities, 10% of those with type II diabetes mellitus had a past acute myocardial infarction episode, while 6% had a past stroke. The western region of Singapore had the highest number of high-risk individuals at 173,000 with at least one chronic Citation: Tan, K.W.; Koo, J.R.; Lim, condition, followed by the east at 169,000 and the north with the least at 137,000. Such estimates can J.T.; Cook, A.R.; Dickens, B.L. assist in healthcare resource planning, which requires these spatial distributions for evidence-based Importance of Geospatial policymaking and to investigate why such heterogeneities exist. The methodologies presented can be Heterogeneity in Chronic Disease utilized within any urban setting where census data exists. Burden for Policy Planning in an Urban Setting Using a Case Study of Singapore. Int. J. Environ. Res. Public Keywords: statistical modeling; chronic disease; spatial epidemiology; urbanization; environmen- Health 2021, 18, 4406. https:// tal health doi.org/10.3390/ijerph18094406 Academic Editor: Antonio Palazón-Bru 1. Introduction Chronic diseases are the main cause of mortality and morbidity in developed countries, Received: 21 March 2021 and constitute a large proportion of the healthcare burden [1]. The direct medical costs of Accepted: 16 April 2021 various chronic diseases have been estimated to be US$3200–4700 for each type II diabetes Published: 21 April 2021 mellitus (DM) case annually [2], and US$ 14,000 and US$ 25,000 for each episode of acute myocardial infarction (AMI) and acute ischaemic stroke, respectively [3]. Globally, rising Publisher’s Note: MDPI stays neutral life expectancy has resulted in aging populations such as those in Japan and Taiwan. From with regard to jurisdictional claims in 2006 to 2015, the DM prevalence in Japan increased from 12.3% to 19.5% for men and 8.2% published maps and institutional affil- to 9.2% for women [4]. For Taiwan, the prevalence of multiple chronic conditions increased iations. from 9.6% to 17.1% from 2000 to 2010 [5]. Singapore, an island city state, experienced an increase in DM prevalence from 8.3% to 8.6% from 2010 to 2017 [6], and from 2007 to 2016, the annual number of AMI and stroke cases increased from 6817 to 10,728 [7] and 5578 to 7413 [8]. As the number of chronic disease cases continues to rise, health systems need to Copyright: © 2021 by the authors. evaluate the accessibility of healthcare facilities and whether they can meet the increase in Licensee MDPI, Basel, Switzerland. demand. This article is an open access article To evaluate accessibility and capacity planning, health systems need to identify where distributed under the terms and cases are in physical space. A method to perform such an analysis would be to use electronic conditions of the Creative Commons medical records, registries, or other databases that record incident cases [9]. Another way Attribution (CC BY) license (https:// would be to conduct cross-sectional observational studies with the aim of investigating creativecommons.org/licenses/by/ incidence and prevalence [10]. However, there are certain downsides. Electronic medical 4.0/). Int. J. Environ. Res. Public Health 2021, 18, 4406. https://doi.org/10.3390/ijerph18094406 https://www.mdpi.com/journal/ijerph Int. J. Environ. Res. Public Health 2021, 18, 4406 2 of 12 records only contain information on when an individual made contact with a particular health service which could introduce bias [11]. Multiple regional health systems also have differing diagnostic criteria and capture patient information differently, leading to challenges in linkage to create a single coherent dataset for analysis [12–14]. Health data being held by different organizations or institutions further exacerbates the problem of linkage [13]. The same individual who developed two chronic conditions such as AMI and stroke would be entered into different registries or hospital records, making these data sources challenging to work with to answer research questions on comorbidities. Surveys and cross-sectional studies provide a useful single estimate for a point in time, but performing regular cross-sectional studies akin to that of annual population health surveys quickly becomes time and resource intensive [15]. An alternative to answer these research questions is using computational epidemiological methods that provides a quick way to obtain data-driven estimates of incidence or prevalence for research or capacity planning purposes [16]. As computing power improves, computational simulation models are becoming more widely used in public health research. Specifically, chronic diseases develop over a long period of time, and as repeated epidemiological studies are costly and infeasible, computational approaches are useful tools to obtain preliminary estimates, a statement echoed by Barhak et al. [17]. Models such as the Population Health Model in Canada and the United Kingdom Prospective Diabetes Study risk engine are examples of simulation tools that have benefited public health research by providing chronic disease estimates to inform policy [18,19]. For geospatial analyses, a study in New York City developed models from claims data to predict diabetes, hypertension, and asthma prevalence by census tract and visualized the results on a map, creating a view of the geographic distribution of chronic diseases that is easy to understand at a glance [20]. Together, these studies showcase some applications of computational modeling to improve population health. In this study, we aimed to estimate the present health risk of the resident population of Singapore, an island city state measuring 50 by 27 kilometers, for DM, specifically type II DM, AMI, and stroke using an individual-based model and identify where cases are located geospatially. This model allows us to investigate the presence of comorbidities in the local population quickly without the need to link up patient identifiers between different registries while at the same time provide information as to where these cases are concentrated in physical space. Given that individuals with multiple comorbidities are high-risk and are most likely to seek medical care, we aggregated the number of high-risk individuals by regional health system to generate estimates that are useful for capacity planning in local health institutions. 2. Materials and Methods To perform spatial imputation for a given population, information on the demographic breakdown of the population and the relationship between demographics and geography is required. In this study, we used a temporally explicit agent-based model of the population of Singapore with the demographic attributes of ethnicity, gender, and age by Phan et al. to create a synthetic population of Singapore [21]. This model simulates the population of Singapore from 1990 to 2050 by aging the population year on year, allowing individuals to develop disease such as DM, as well as give birth and die with rates derived from the birth and death registry of Singapore. The population engine was further augmented with disease models of AMI and stroke by Tan et al. to simulate onset of AMI and stroke to create a comprehensive population engine that simulates multiple chronic conditions [22]. As the population engine predicts population health over 60 years, we extracted a cross-section of the population in 2020 to investigate the spatial distribution of chronic disease cases. The General Household Survey 2015 contains census tables that correlate demographic attributes such as ethnicity, gender and age with physical location [23]. However, a common limitation of censuses with the intention to protect privacy is to obscure individual-level data by publishing anonymized aggregate statistics [24]. This is observed in the General Int. J. Environ. Res. Public Health 2021, 18, 4406 3 of 12 Household Survey where one census table relates physical location to gender and ethnicity while another relates physical location to age. This data was used to recreate a consistent synthetic population across the dimensions of physical location, ethnicity, gender, and age. A hill climbing algorithm was chosen to optimize across the multiple dimensions. The steps of the algorithm within the context of the study are as follows: 1. Seed random initial physical locations for the synthetic population; 2. Calculate the chi-square statistic for each census table using the synthetic population as the observed values and the census as the expected values; 3. Select two random individuals in the population and swap their physical locations; 4. Calculate the new chi-square statistic for each census table. If the new chi-square statistic is lower than the previous one, accept the swap. If not, reject the swap and revert the physical locations to their original values; 5. Repeat steps 2–4 until improvement in the chi-square statistic is marginal, implying optimal convergence.
Details
-
File Typepdf
-
Upload Time-
-
Content LanguagesEnglish
-
Upload UserAnonymous/Not logged-in
-
File Pages12 Page
-
File Size-