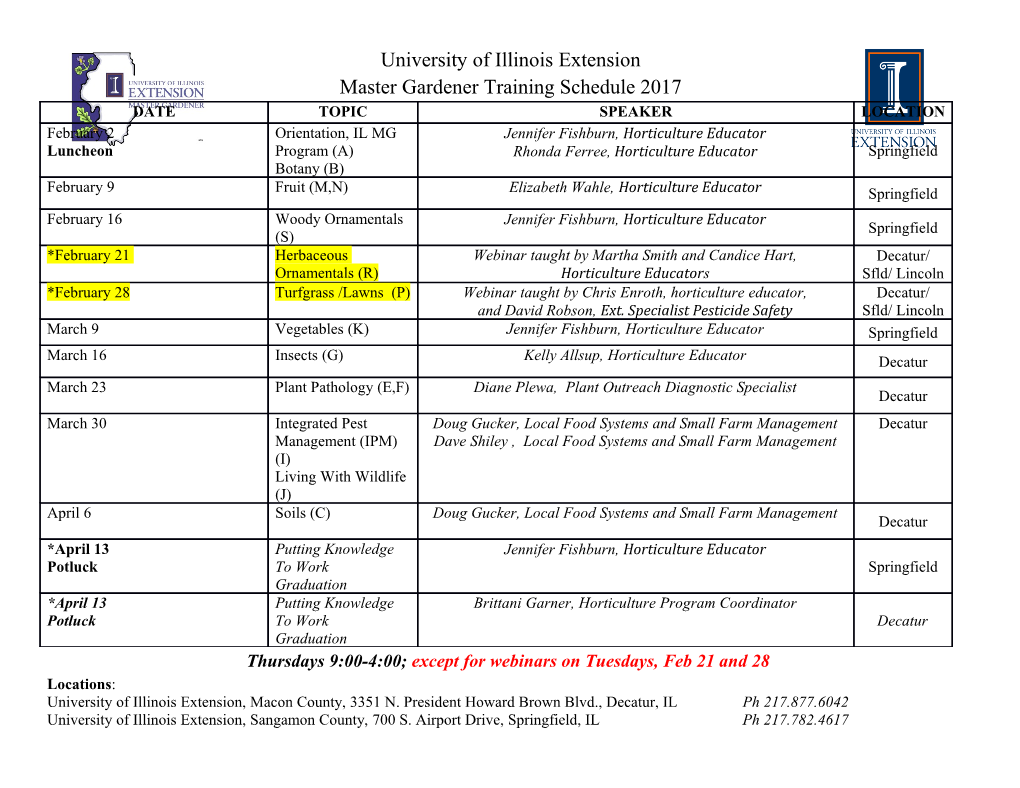
Characterizations of Psychometrics Lisa D. Wijsen Characterizations of Psychometrics Lisa D. Wijsen 2020 Characterizations of Psychometrics ACADEMISCH PROEFSCHRIFT ter verkrijging van de graad van doctor aan de Universiteit van Amsterdam op gezag van de Rector Magnificus prof. dr. ir. K.I.J. Maex ten overstaan van een door het College voor Promoties ingestelde commissie, in het openbaar te verdedigen in de Agnietenkapel op 22 maart 2021 door Lisa Dide Wijsen geboren te Utrecht Characterizations of Psychometrics © Lisa D. Wijsen, 2021. All rights reserved. No part of this thesis may be reproduced in any form or by any means without permission of the author. The research in this dissertation was funded by a NWO Graduate Programme Grant (given out by IOPS). ISBN: 978-94-92332-33-2 Cover & Layout: Esther Scheide, proefschriftomslag.nl Printed by: ProefschriftMaken Promotores: Prof. Dr. D. Borsboom Universiteit van Amsterdam Contents Prof. Dr. W. J. Heiser Universiteit Leiden Chapter 1 General Introduction 9 1.1 Positioning Psychometrics 12 Overige leden: Prof. Dr. Agneta Fischer Universiteit van Amsterdam 1.2 Different Perspectives on Psychometrics 14 Prof. Dr. Han van der Maas Universiteit van Amsterdam 1.2.1 The Historian 14 Prof. Dr. Rens Bod Universiteit van Amsterdam 1.2.2 The Ethnographer 15 Dr. Anna Alexandrova Cambridge University 1.2.3 The Philosopher of Science 16 Dr. Femke Truijens Erasmus Universiteit 1.3 The Merit of this Dissertation 17 Prof. Dr. Klaas Sijtsma Tilburg Universiteit Chapter 2 An Academic Genealogy of Psychometric Society presidents 19 2.1 Introduction 21 2.2 Method 22 2.3 Results 23 2.3.1 The Genealogy of James R. Angell 26 2.3.2 The Genealogy of Wilhelm Wundt, part I 28 2.3.3 The Genealogy of William James 35 2.3.4 The Genealogy of Albert E. Michotte 39 2.3.5 The Genealogy of Carl F. Gauss 40 2.3.6 Lineages of Other Presidents 43 2.4 Conclusion & Discussion 46 2.4.1 Missing Offspring 47 2.4.2 Disciplinary Boundaries 48 2.4.3 Increasing Diversity 49 2.4.4 Limitations 50 Chapter 3 Perspectives on Psychometrics: Interviews with 20 past Psychometric Society Presidents 53 3.1 Introduction 55 3.2 Methods 57 3.3 Themes 58 3.3.1 Key Moments in the History of Psychometrics 59 3.3.2 The Dark Ages of Psychometrics 61 3.3.3 The Relationship between Psychometrics, Psychology, and Statistics 63 3.3.4 The Identity of the Psychometrician: A Multitude of Approaches 68 3.3.5 The Future of Psychometrics 70 3.3.6 Recommendations 73 6.3.1 Eugenics and a New Social Order 128 3.4 Conclusion & Discussion 74 6.3.2 Military Testing as Spin-Off for Standardized Mental 3.4.1 Some Limitations 76 Testing 130 3.4.2 Acknowledgements 77 6.3.3 A Gradual Shift in the Self-Conception of Psychometrics 131 6.4 ‘Value-free’ State-of-the-Art Psychometrics 133 Chapter 4 Reflective, Formative, and Network models: 6.5 Values in Contemporary Psychometrics 135 Causal Interpretations of Three Different Psychometric Models 79 6.5.1 Individual Differences are Quantitative, not Qualitative 135 4.1 Introduction 81 6.5.2 Objectivity 138 4.2 Three Models for the Relation Between Constructs and Measures 82 6.5.3 Fairness 139 4.2.1 Reflective Measurement Models 82 6.5.4 Utility above Truth 140 4.2.2 Formative Measurement Models 85 6.6 Conclusion & Discussion 142 4.2.3 Network Models 87 4.3 Empirical Consequences of the Different Models 90 Chapter 7 General Discussion 145 4.4 Controversies 93 7.1 Aims and Main Findings 147 4.4.1 The Causal Status of Individual Differences 93 7.2 Paradoxes of Psychometrics 148 4.4.2 Generalization 95 7.2.1 Where is the ‘Psycho’ in Psychometrics? 149 4.4.3 Interpretational Confounding 97 7.2.2 Psychometrics is Committed to Social Problems, 4.4.4 The Relationship between Causal Models and Data Models 99 but (Almost) Never Explicitly 150 4.4.5 A Realist Philosophy of Psychometrics vs. Psychometric 7.2.3 Psychometrics as Engineering, not as Science 151 Practice 100 7.3 Implications for Further Research 152 4.5 Conclusion 101 7.3.1 A Full Ethnography of Psychometrics 152 7.3.2 A Pragmatist Philosophy of Psychometrics 154 Chapter 5 A Causal Interpretation of the Common Factor Model 105 7.3.3 The Performativity of Psychometric Concepts 155 5.1 Introduction 107 7.3.4 Discipline Formation of Psychometrics 157 5.2 Statistical vs. Causal Models 110 7.4 Conclusion 158 5.3 Correlation Does Not Entail Causation 112 5.4 What is the Common Factor in the Descriptivist Approach? 113 5.5 Toward a Causal Interpretation 113 References 163 5.5.1 “Look, we found shared variance!” 114 Appendix A 181 5.5.2 Why Shared Variance at all? 115 Appendix B 191 5.5.3 Local Independence 116 Nederlandse samenvatting (Dutch summary) 193 5.6 How to Assess whether a Common Cause Structure is Correct 117 English Summary 199 5.7 Conclusion 119 Publications 203 Dankwoord (Acknowledgements) 205 Chapter 6 Values in Psychometrics 123 6.1 Introduction 125 6.2 A Brief Note on Terminology 127 6.3 Values in Early Psychometrics 128 Chapter 1 General Introduction General Introduction Psychometrics is best-known for its applications that so many of us are familiar with. When Dutch school children are about 11 or 12 years old – and I remember this clearly –, they participate in what is called the CITO test: an elaborate assessment that provides 1 information on a child’s progress on skills like reading ability and mathematics, and helps guide teachers in giving advice on a child’s future school choice. When we grow older and apply for a job, we may be asked to do an assessment that maps the applicant’s desirable skills and traits, and for a person with mental health problems, measurement instruments like the Beck Depression Inventory or the Hamilton Anxiety Rating Scale might help in determining the appropriate diagnosis. In different phases of our lives, we encounter psychometric applications, which can be very influential or even decisive in high-stake situations. Was your job assessment satisfactory to your future employer? Are your SAT scores high enough for admission into a top-notch Ivy-League university? However, even though most of us are familiar with different examples of psychometric applications, psychometrics as a research area is much more mysterious. Psychometrics is a very complex scientific discipline: its content is often highly tech nical, heavily embedded in statistics, and its connection with psychological or edu- cational applications is often difficult to grasp (and sometimes, on the face of it, non- existent). These aspects make psychometrics’ purposes, goals, values, and concepts hard to understand for an outsider who is not part of the psychometric community. So, what is it that psychometricians actually do, and why do they do it? In this dissertation, I explore different aspects of the scientific domain of psychometrics – its disciplinary structure, its historical development, its practices, its models, and its values – to improve our understanding of this lesser-known and complex discipline. Psychometrics as understood in this dissertation is the field that concerns itself with the measurement and prediction of human behavior. Psychometric research usually entails a thorough investigation into the development and/or application of one or more psychometric models, which are often members of the families of Item Response Theory (IRT, Embretson & Reise, 2000; Van der Linden & Hambleton, 2013), Structural Equation Modeling (SEM, Jöreskog, 1970; 1973), Multidimensional Scaling (Kruskal & Wish, 1978), and, particularly at my university, Network Models (Borsboom & Cramer, 2013; Epskamp, Rhemtulla, & Borsboom, 2017). IRT models are tools for analyzing testing data (item responses), by modeling the relationship between item responses and a latent variable (an unobservable characteristic or attribute such as reading ability or intelligence). Structural Equation Modeling is a method for analyzing structural analyses among two or more latent variables, for example, the structural relationship between reading ability and spatial reasoning. Originally, psychometric research mainly concerned itself with methods for the analysis of test data, and developed concepts such as reliability, validity, and factor – 11 – Chapter One General Introduction analysis (possibly three of the most influential and most exported psychometric con- 2006; Sijtsma, 2006; Groenen & Van der Ark, 2006). This detachment between the cepts). Educational measurement, the domain of IRT, is still one of the most important substantive and the technological has not always been as prominent as it is now: early focus points of psychometrics, but throughout the 20th century, psychometric models psychometricians were often psychologists who were heavily engaged in a substantive 1 (and especially factor and SEM models) have been applied to quantitative research in problem (e.g. the nature of individual differences) and simultaneously tried to solve or a wide variety of domains such as personality (McCrae & Costa, 1987; Marsh et al., explain this phenomenon through technically advanced methods. Nowadays, the shared 2010), mental health (Compas et al., 2006; Caspi et al., 2014), and marketing research ancestry with psychology has become somewhat invisible. Psychometrics is incredibly (Baumgartner & Homburg, 1996). Moreover, not all psychometric models are strictly technical, quantitative, and heavily embedded in statistics; it is highly specialized and measurement models. An important branch in psychometrics that came up in the 1960s difficult to understand for anyone without a thorough training in statistics or psychometrics. is Multidimensional Scaling (MDS), which is – very generally put – a method for the The relationship between psychometric research and topics in psychology or educational representation of similarities and dissimilarities in data and is a popular method in measurement is hard to grasp.
Details
-
File Typepdf
-
Upload Time-
-
Content LanguagesEnglish
-
Upload UserAnonymous/Not logged-in
-
File Pages106 Page
-
File Size-