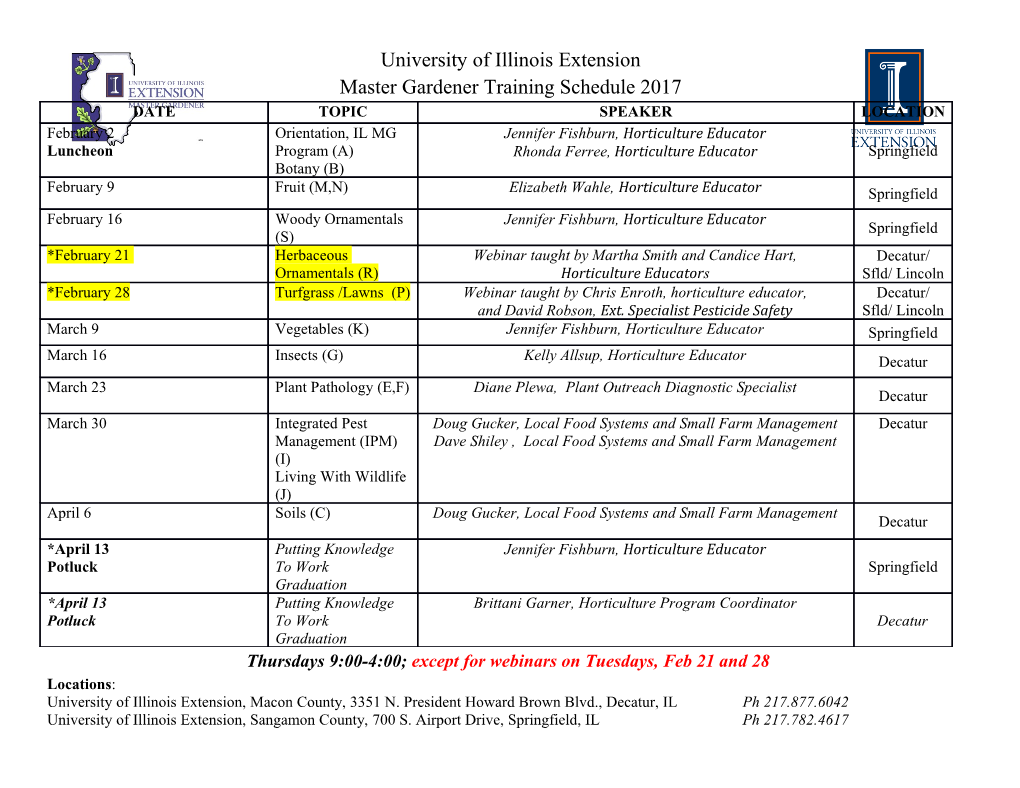
Algebraic methods for biochemical reaction network theory by Anne Joyce Shiu A dissertation submitted in partial satisfaction of the requirements for the degree of Doctor of Philosophy in Mathematics and the Designated Emphasis in Computational and Genomic Biology in the Graduate Division of the University of California, Berkeley Committee in charge: Professor Bernd Sturmfels, Chair Professor Lior Pachter Professor Murat Arcak Spring 2010 Algebraic methods for biochemical reaction network theory Copyright 2010 by Anne Joyce Shiu 1 Abstract Algebraic methods for biochemical reaction network theory by Anne Joyce Shiu Doctor of Philosophy in Mathematics and the Designated Emphasis in Computational and Genomic Biology University of California, Berkeley Professor Bernd Sturmfels, Chair This dissertation develops the algebraic study of chemical reaction networks endowed with mass- action kinetics. These form a class of dynamical systems that have a wide range of applications in the physical and biological sciences. Early results in chemical reaction network theory relied on techniques from linear algebra, dynamical systems, and graph theory. More recently, motivated by problems in systems biology, other areas of mathematics have contributed to this subject. These branches of mathematics include control theory, homotopy theory, and matroid theory. As a complement to these varied perspectives, the approach of this dissertation is algebraic. Chapter 2 develops the basic theory of toric dynamical systems, which are those chemical reaction systems that have the property that for any chemical complex (a product or reactant), the amount produced of that complex at steady state is equal to the amount consumed by reactions. Toric dynamical systems are known as complex-balancing mass-action systems in the mathematical chemistry literature, where many of their properties have been established. Special cases of toric dynamical systems include all zero deficiency systems and all detailed-balancing systems. One feature is that the steady state locus of a toric dynamical system is a toric variety. Furthermore, this variety intersects the interior of each invariant polyhedron (a polyhedron in which a trajectory of the dynamical system is confined) in a unique point. For any chemical reaction network, there is an associated moduli space that consists of those vectors of reaction rate constants for which the resulting dynamical system is a toric dynamical system. The main result states that this moduli space is a toric variety whose combinatorial structure we can characterize. To determine the steady states on the boundaries of invariant polyhedra, the concept of a siphon is important. Siphons in a chemical reaction system are subsets of the chemical species that have the potential of being absent in a steady state. The main result of Chapter 3 characterizes minimal siphons in terms of primary decomposition of binomial ideals. Further, we explore the underlying geometry, and we demonstrate the effective computation of siphons using computer algebra software. This leads to a new method for determining whether given initial concentrations allow for various boundary steady states; this classification arises from a chamber decomposition. Siphons determine which faces of an invariant polyhedron contain steady states, and a rel- evant question is whether any trajectories of a chemical reaction system approach such a boundary 2 steady state. The global attractor conjecture, which is the subject of Chapter 4, implies that no inte- rior trajectories approach boundary steady states in the case of toric dynamical systems. Our main result states that this conjecture holds when all of the siphons correspond to facets (codimension- one faces), vertices (zero-dimensional faces), or empty faces of the invariant polyhedron. As a corollary, the conjecture holds when the associated invariant polyhedra are two-dimensional. Chapter 5 pertains to the topic of multistationarity, which refers to the capacity of a biochemical reaction system to exhibit multiple steady states in one invariant polyhedron. Known results from chemical reaction network theory provide sufficient conditions for the existence of bista- bility, and on the other hand can rule out the possibility of multiple steady states. Understanding small networks is important because the existence of multiple steady states in a subnetwork of a biochemical model sometimes can be lifted to establish multistationarity in the larger network. The main result establishes the smallest reversible, mass-preserving network that admits bistability and determines the semi-algebraic set of parameters for which more than one steady state exists. Chapter 6 focuses on mathematical methods for predicting gene expression from regulatory sequence. The chemical reactions that underlie transcriptional regulation include the bindings of transcription factors to cis-regulatory sequences of genes. For each such sequence, many possible combinations of transcription factors can bind to the sequence. Accordingly, thermodynamic models give quantitative predictions of gene expression levels that are weighted averages over the set of all possible binding configurations. This chapter describes the implementation of such a model in the context of early embryonic development in Drosophila melanogaster. i To my family. ii Contents List of Figures v List of Tables vi 1 Introduction 1 1.1 From molecular biology to systems biology . ............ 1 1.2 Overview of main results in this dissertation . .............. 2 1.3 Chemical reaction network theory . .......... 3 1.3.1 Chemicalreactionsystems. ...... 4 1.3.2 Rateformationfunctions . ..... 6 1.3.3 Steady states and invariant polyhedra . ......... 7 1.3.4 The deficiency of a chemical reaction network . .......... 7 1.3.5 Ideals, varieties, and primary decomposition . ............. 8 1.3.6 Connection to polyhedral geometry . ........ 8 1.4 Examplesofreactionnetworks . ........ 9 2 Toric dynamical systems 12 2.1 Introduction to toric dynamical systems . ............ 12 2.2 Ideals, varieties, and chemistry . ........... 14 2.2.1 The Matrix-Tree Theorem and the toric balancing ideal ............ 16 2.2.2 Mainresults................................... 20 2.3 The Global Attractor Conjecture . ......... 22 2.4 Connections to deficiency theory . ......... 24 2.4.1 Deficiencytheorems ............................. 24 2.4.2 Stochastic complex-balancing chemical systems . ............. 25 2.5 Biological applications . ......... 25 2.6 Detailed-balancing systems . ......... 28 3 Siphons and primary decomposition 30 3.1 Introductiontosiphons . ....... 30 3.2 Reaction networks, siphons, and steady states . .............. 32 3.3 Binomial ideals and monomial ideals . .......... 34 3.4 Computingsiphonsinpractice . ........ 39 iii 3.5 Siphonsandpersistence . ....... 44 4 The global attractor conjecture 45 4.1 Introduction.................................... ..... 45 4.2 Boundary steady states, persistence, and siphons . ............... 46 4.3 Historyoftheconjecture. ........ 48 4.4 Preliminaryresults .............................. ....... 50 4.5 Results for detailed-balancing systems . ............. 51 4.6 Mainresults..................................... 54 4.6.1 Result concerning vertices of invariant polyhedra . .............. 54 4.6.2 Result concerning facets of invariant polyhedra . ............. 55 4.6.3 Connection to dynamically non-emptiable siphons . ............ 58 4.7 Applications to the global attractor conjecture . ............... 61 4.8 Examples ........................................ 62 5 The smallest multistationary mass-preserving chemical reaction network 65 5.1 Introduction to multistationarity . ............ 65 5.2 The smallest multistationary network . ........... 66 5.3 Parametrizing multistationarity . ............ 70 5.4 Multistationary subnetworks of the Square network . ............... 73 5.5 Connection to known biochemical networks . ........... 75 6 Predicting gene expression from regulatory sequence 77 6.1 Introduction.................................... ..... 77 6.2 The gene expression function of Segal et al. ....................... 78 6.2.1 Configurationmodels . .. .. .. .. .. .. .. .. 78 6.2.2 Configuration probabilities and expression level prediction . 79 6.2.3 Parameters .................................... 82 6.2.4 Clarifying notes on the Segal et al. gene expression function . 82 6.3 Computingthegeneexpressionfunction . ........... 84 6.3.1 Dynamicprogramming. 84 6.3.2 Head state Markov chain . 88 6.3.3 Logcoordinates................................ 91 6.3.4 Maximum-weight configurations . ...... 92 6.4 Computing the number of configurations . .......... 92 7 Further directions 95 7.1 Basic chemical reaction network theory questions . ............... 95 7.2 Existence of positive steady states . ........... 95 7.3 Homotopy methods for multiple steady states . ............ 96 7.4 Thermodynamic feasibility of toric dynamical systems . ................ 96 7.5 Boundary steady states without repelling neighborhoods................ 96 iv Bibliography 97 v List of Figures 3.1 The chamber decomposition of the cone Q for the network in Example 3.1.1. The cone is spanned by the columns of the matrix A in (3.3). Each of the three maximal chambers Ω(1), Ω(2), and Ω(3) contains a picture of the corresponding 3-dimensional polyhedron Pc(0) . The vertices of each polyhedron are labeled by their supports. The star “⋆” indicates the unique vertex steady state, which arises from one of the two relevant siphons
Details
-
File Typepdf
-
Upload Time-
-
Content LanguagesEnglish
-
Upload UserAnonymous/Not logged-in
-
File Pages116 Page
-
File Size-