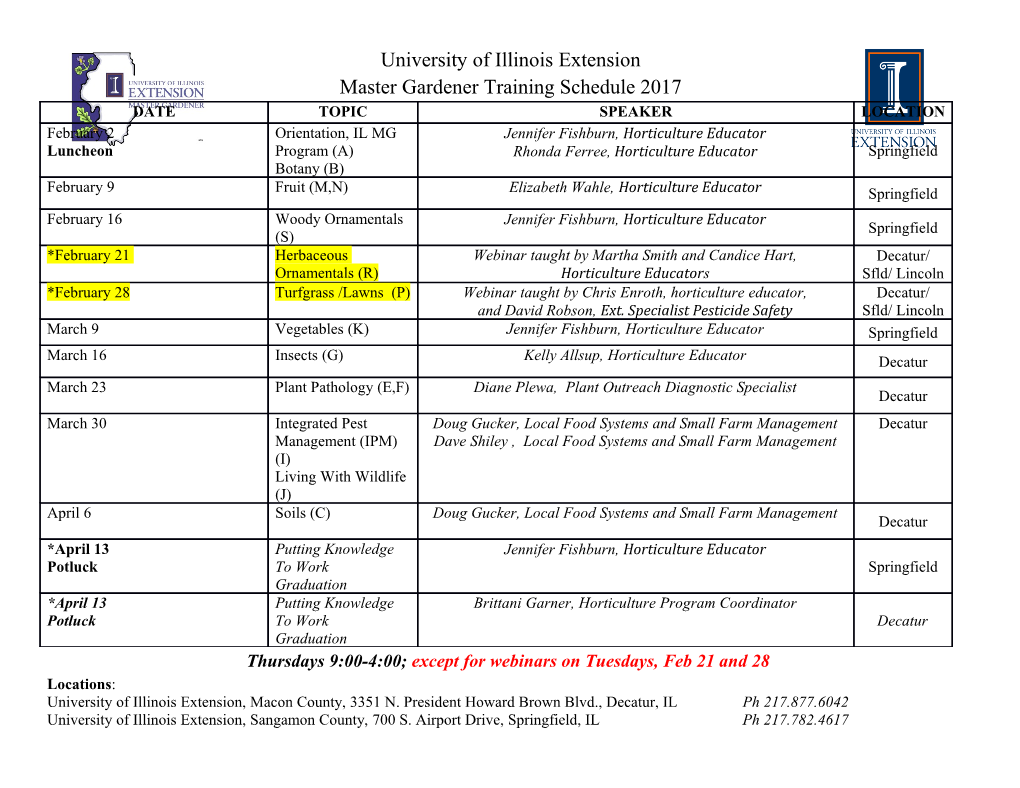
Introduction to Machine Learning Lecture 3 Mehryar Mohri Courant Institute and Google Research [email protected] Bayesian Learning Bayes’ Formula/Rule Terminology: likelihood prior Pr[X Y ]Pr[Y ] Pr[Y X]= | . posterior| Pr[X] probability evidence Mehryar Mohri - Introduction to Machine Learning page 3 Loss Function Definition: function L : R + indicating the penalty for an incorrectY prediction.×Y→ • L ( y,y ) : loss for prediction of y instead of y . Examples: • zero-one loss: standard loss function in classification; L ( y,y )=1 y = y for y,y . ∈Y • non-symmetric losses: e.g., for spam classification; L ( ham , spam) L ( spam , ham) . ≤ squared loss: standard loss function in • 2 regression; L ( y,y )=( y y ) . − Mehryar Mohri - Introduction to Machine Learning page 4 Classification Problem Input space : e.g., set of documents. X N feature vector Φ ( x ) R associated to x . • ∈ ∈X N notation: feature vector x R . • ∈ • example: vector of word counts in document. Output or target space : set of classes; e.g., sport, Y business, art. Problem: given x , predict the correct class y ∈Y associated to x . Mehryar Mohri - Introduction to Machine Learning page 5 Bayesian Prediction Definition: the expected conditional loss of predicting y is ∈Y [y x]= L(y,y)Pr[y x]. L | | y ∈Y Bayesian decision : predict class minimizing expected conditional loss, that is y∗ =argmin [y x]=argmin L(y,y)Pr[y x]. L | | y y y ∈Y zero-oneb loss: y∗ =argmaxb Pr[y x]. • y | Maximum a Posteriori (MAP) principle. b Mehryar Mohri - Introduction to Machine Learning page 6 Binary Classification - Illustration 1 Pr[y x] 1 | Pr[y x] 2 | 0 x Mehryar Mohri - Introduction to Machine Learning page 7 Maximum a Posteriori (MAP) Definition: the MAP principle consists of predicting according to the rule y =argmaxPr[y x]. y | ∈Y Equivalently, by the Bayes formula: Pr[x y]Pr[y] y =argmax | =argmaxPr[x y]Pr[y]. y Pr[x] y | ∈Y ∈Y How do we determine Pr[ x y ] and Pr[ y ] ? Density estimation problem.| Mehryar Mohri - Introduction to Machine Learning page 8 Application - Maximum a Posteriori Formulation: hypothesis set H . Pr[O h]Pr[h] hˆ =argmaxPr[h O]=argmax | =argmaxPr[O h]Pr[h]. h H | h H Pr[O] h H | ∈ ∈ ∈ Example: determine if a patient has a rare disease H = d, nd , given laboratory test O = pos, neg . { } { } With Pr[ d ]= .005 , Pr[ pos d ]= .98 , Pr[ neg nd ]= . 95 , if the test | | is positive, what should be the diagnosis? Pr[pos d]Pr[d]=.98 .005=.0049. | × Pr[pos nd]Pr[nd]=(1 .95) .(1 .005)=.04975>.0049. | − × − Mehryar Mohri - Introduction to Machine Learning page 9 Density Estimation Data: sample drawn i.i.d. from set X according to some distribution D , x1,...,xm ∈ X. Problem: find distribution p out of a set P that best estimates D . • Note: we will study density estimation specifically in a future lecture. Mehryar Mohri - Introduction to Machine Learning page 10 Maximum Likelihood Likelihood: probability of observing sample under distribution p ∈ P , which, given the independence assumption is m Pr[x1,...,xm]=! p(xi). i=1 Principle: select distribution maximizing sample probability m p! = argmax ! p(xi), ∈P p i=1 m or p! = argmax ! log p(xi). ∈P p i=1 Mehryar Mohri - Introduction to Machine Learning page 11 Example: Bernoulli Trials Problem: find most likely Bernoulli distribution, given sequence of coin flips H, T, T, H, T, H, T, H, H, H, T, T, . ., H. Bernoulli distribution: p(H)=θ, p(T )=1 θ. − Likelihood: l(p)=logθN(H)(1 θ)N(T ) = N(H)logθ +−N(T )log(1 θ). − Solution: l is differentiable and concave; dl(p) N(H) N(T ) N(H) = =0 θ = . dθ θ − 1 θ ⇔ N(H)+N(T ) − Mehryar Mohri - Introduction to Machine Learning page 12 Example: Gaussian Distribution Problem: find most likely Gaussian distribution, given sequence of real-valued observations 3.18, 2.35,.95, 1.175,... 1 (x µ)2 Normal distribution: p(x)= exp − 2 . √2πσ2 !− 2σ " 1 m (x µ)2 Likelihood: l(p)= m log(2πσ2) i − . −2 − 2σ2 i=1 Solution: l is differentiable and concave; m m ∂p(x) 1 ∂p(x) 2 1 2 2 =0⇔ µ = xi =0⇔ σ = x − µ . ∂µ m ! 2 ! i i=1 ∂σ m i=1 Mehryar Mohri - Introduction to Machine Learning page 13 ML Properties Problems: • the underlying distribution may not be among those searched. • overfitting: number of examples too small wrt number of parameters. • Pr[ y ]=0 if class y does not appear in sample! smoothing techniques. Mehryar Mohri - Introduction to Machine Learning page 14 Additive Smoothing Definition: the additive or Laplace smoothing for estimating Pr[ y ] , y , from a sample of size m is defined by ∈Y y + α Pr[y]= | | . m + α |Y| • α =0 : ML estimator (MLE). • MLE after adding α to the count of each class. • Bayesian justification based on Dirichlet prior. • poor performance for some applications, such as n-gram language modeling. Mehryar Mohri - Introduction to Machine Learning page 15 Estimation Problem Conditional probability: Pr[x y]=Pr[x ,...,x y]. | 1 N | • for large N , number of features, difficult to estimate. even if features are Boolean, that is x 0 , 1 , • i ∈{ } there are 2 N possible feature vectors! may need very large sample. Mehryar Mohri - Introduction to Machine Learning page 16 Naive Bayes Conditional independence assumption: for any y , ∈Y Pr[x ,...,x y]=Pr[x y] ...Pr[x y]. 1 N | 1 | N | • given the class, the features are assumed to be independent. • strong assumption, typically does not hold. Mehryar Mohri - Introduction to Machine Learning page 17 Example - Document Classification Features: presence/absence of word x i . Estimation of Pr[ x y ]: frequency of word x i among i | documents labeled with y , or smooth estimate. Estimation of Pr[ y ] : frequency of class y in sample. Classification: N y =argmaxPr[y] Pr[x y]. i | y i=1 ∈Y Mehryar Mohri - Introduction to Machine Learning page 18 Naive Bayes - Binary Classification Classes: = 1 , +1 . Y {− } Pr[+1 x] Decision based on sign of log Pr[ 1 | x ] ; in terms of log-odd ratios: − | Pr[+1 x] Pr[+1] Pr[x +1] log | =log | Pr[ 1 x] Pr[ 1] Pr[x 1] − | − |− Pr[+1] N Pr[x +1] =log i=1 i | Pr[ 1] N Pr[x 1] − i=1 i |− N Pr[+1] Pr[xi +1] =log + log | . Pr[ 1] Pr[x 1] i=1 i − |− contribution of feature/expert i to decision Mehryar Mohri - Introduction to Machine Learning page 19 Naive Bayes = Linear Classifier Theorem: assume that x i 0 , 1 for all i [1 ,N ] . Then, the Naive Bayes classifier∈{ }is defined∈ by x sgn(w x + b), → · Pr[xi=1 +1] Pr[xi=0 +1] where w i =log Pr[ x =1 | 1] log Pr[ x =0 | 1] i |− − i |− Pr[+1] N Pr[xi=0 +1] and b =logPr[ 1] + i=1 log Pr[x =0| 1] . − i |− Proof: observe that for any i [1 ,N ] , ∈ Pr[x +1] Pr[x =1 +1] Pr[x =0 +1] Pr[x =0 +1] log i | = log i | log i | x +log i | . Pr[x 1] Pr[x =1 1] − Pr[x =0 1] i Pr[x =0 1] i |− i |− i |− i |− Mehryar Mohri - Introduction to Machine Learning page 20 Summary Bayesian prediction: • requires solving density estimation problems. N often difficult to estimate Pr[ x y ] for x R . • | ∈ • but, simple and easy to apply; widely used. Naive Bayes: • strong assumption. • straightforward estimation problem. • specific linear classifier. • sometimes surprisingly good performance. Mehryar Mohri - Introduction to Machine Learning page 21.
Details
-
File Typepdf
-
Upload Time-
-
Content LanguagesEnglish
-
Upload UserAnonymous/Not logged-in
-
File Pages21 Page
-
File Size-