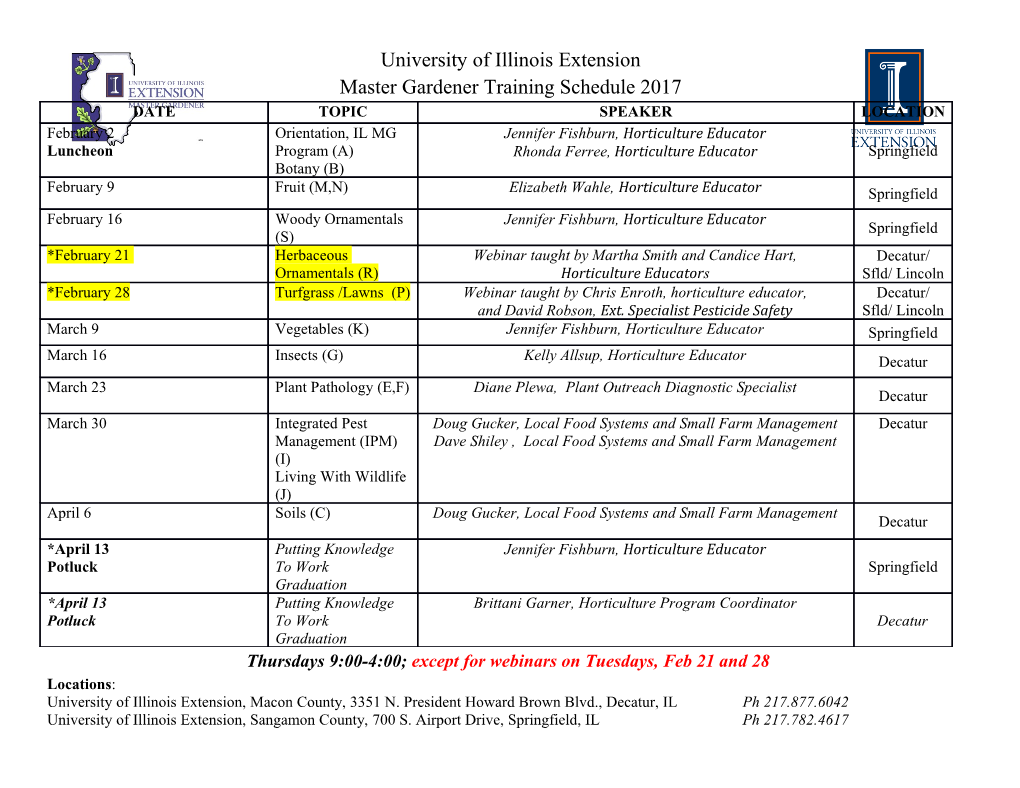
SAMPLE DELIVERABLES 17 PILOT APPLICATIONS Grant agreement No: SSH - CT - 2007 - 217565 Project Acronym: SAMPLE Project Full title: Small Area Methods for Poverty and Living Conditions Estimates Funding Scheme: Collaborative Project - Small or medium scale focused research project Deliverable n.: D17 Deliverable name: Pilot applications WP n.: 2 Lead Beneficiary: 1, 5 Nature: Report Dissemination level: PU Status: Final Due delivery date: September 30th, 2010 Actual delivery date: November 16th, 2010 Project co-ordinator name: Monica Pratesi Title: Associate Professor of Statistics - University of Pisa Organization: Department of Statistics and Mathematics Applied to Economics of the University of Pisa (UNIPI-DSMAE) Tel.: +39-050-2216252, +39-050-2216492 Fax: +39-050-2216375 E-mail: [email protected] Project website address: www.sample-project.eu PILOT APPLICATIONS Project Acronym: SAMPLE Project Full title: Small Area Methods for Poverty and Living Conditions Estimates Project/Contract No: EU-FP7-SSH-2007-1 Grant agreement No: 217565 Work Package 2: Small area estimation of poverty and inequality indicators Document title: Pilot applications Date: October 30th, 2010 Type of document: Deliverable D17 Status: Final Editors: I. Molina, D. Morales, M. Pratesi and N. Tzavidis Authors: Monica Pratesi ([email protected]), UNIPI-DSMAE Caterina Giusti ([email protected]), UNIPI-DSMAE Stefano Marchetti ([email protected]fi.it), UNIPI-DSMAE Nicola Salvati ([email protected]), UNIPI-DSMAE Nikos Tzavidis ([email protected]), Southampton Isabel Molina ([email protected]), UC3M Mar´ıa Durban´ ([email protected]), UC3M Aurea Grane´ ([email protected]), UC3M Juan Miguel Mar´ın ([email protected]), UC3M Maria Helena Veiga ([email protected]), UC3M Domingo Morales ([email protected]), UMH Mar´ıa Dolores Esteban ([email protected]), UMH Angel´ Sanchez´ ([email protected]), UMH Laureano Santamar´ıa ([email protected]), UMH Yolanda Marhuenda ([email protected]), UMH Agust´ın Perez´ ([email protected]), UMH Maria Chiara Pagliarella ([email protected]), UMH J.N.K. Rao ([email protected]), Carleton University Caterina Ferretti ([email protected]fi.it), University of Florence Contents Prologue 1 1 The Spanish data 3 1.1 The Spanish Living Conditions Survey . .3 1.2 The Economically Active Population Survey . .7 2 The Italian data 9 2.1 EU-SILC Data and Census Microdata . .9 3 Area level time models 13 3.1 Area-level model with correlated time effects . 13 3.1.1 The model . 13 3.1.2 The REML estimators . 14 3.1.3 The EBLUP and its mean squared error . 15 3.2 Area-level model with independent time effects . 17 3.2.1 The model . 17 3.2.2 The Henderson 3 estimator . 17 3.2.3 The REML estimator . 18 3.2.4 The EBLUP and its mean squared error . 19 3.3 Estimation of poverty indicators . 20 4 Partitioned area level time model 27 4.1 The partitioned model . 27 4.1.1 The model . 27 4.1.2 The REML estimators . 28 4.1.3 The EBLUP and its mean squared error . 29 2 2 4.1.4 Testing for H0 : sA = sB .............................. 31 4.2 Estimation of poverty indicators . 31 5 EB prediction of poverty measures with unit level models 43 5.1 EB method for poverty estimation . 43 5.2 Parametric bootstrap for MSE estimation . 44 5.3 Application with the Spanish EU-SILC data . 45 I 6 Fast EB method for estimation of fuzzy poverty measures 53 6.1 Fuzzy monetary and supplementary indicators . 53 6.2 Fast EB method for estimation of fuzzy poverty measures . 55 6.3 Application to EU-SILC data from Tuscany . 57 6.4 Concluding remarks . 59 7 Spatial Fay-Herriot models 61 7.1 The model . 61 7.2 Analytical approximation of the MSE . 62 7.3 Parametric bootstrap estimation of the MSE . 62 7.4 Nonparametric bootstrap . 63 7.5 Application with Spanish EU-SILC data . 65 8 Spatio-temporal Fay-Herriot models 73 8.1 Spatial Fay-Herriot model with uncorrelated time effects . 73 8.1.1 The model . 73 8.1.2 Parametric bootstrap MSE estimation . 74 8.2 Spatial Fay-Herriot model with correlated time effects . 74 8.2.1 The model . 74 8.2.2 Parametric bootstrap estimation of the MSE . 76 8.3 Application with Spanish EU-SILC data . 76 9 Unit level time models 85 9.1 Unit-level model with correlated time effects . 85 9.1.1 The model . 85 9.1.2 The REML estimators . 86 9.1.3 The EBLUP and its mean squared error . 87 9.2 Unit-level model with independent time effects . 88 9.2.1 The model . 88 9.2.2 The REML estimators . 88 9.2.3 The EBLUP and its mean squared error . 89 9.3 Estimation of poverty indicators . 90 10 Review of M-quantile methods for small area estimation 97 10.1 Linear M-quantile regression models . 98 10.1.1 Estimation of small area means and quantiles . 98 10.1.2 Mean Squared Error (MSE) estimation for estimators of small area means and quantiles . 100 10.2 Small Area Models for Poverty Estimation . 102 10.2.1 Definitions of poverty indicators . 102 10.2.2 The M-quantile approach for poverty estimation . 102 10.2.3 Alternative measures for poverty: fuzzy indicators at a small area level with M- quantile models . 103 10.3 Nonparametric M-quantile regression models in small area estimation . 104 II 10.3.1 Small area estimator of the mean and of the quantiles . 106 10.3.2 Mean squared error estimation . 107 10.4 M-quantile GWR models . 108 10.4.1 M-quantile geographically weighted regression . 108 10.4.2 Using M-quantile GWR models in small area estimation . 110 10.4.3 Mean squared error estimation . 111 10.5 Semiparametric Fay-Herriot model using penalized splines . 112 10.5.1 Estimation of small area means . 112 10.5.2 Estimation of the MSE . 114 11 Estimating Small Area Averages 117 11.1 M-quantile Models . 117 11.2 Nonparametric M-quantile Model . 119 11.3 M-quantile GWR Model . 129 11.4 Semiparametric Fay and Herriot model . 130 12 Estimating Small Area Income Distribution Functions 137 12.1 M-quantile Models . 137 12.2 Nonparametric M-quantile Model . 150 13 Estimating Poverty Indicators 163 13.1 M-quantile Models . 164 14 References 173 A Table of the average equivalised income at municipality level in Toscana; M-quantile GWR model 183 B Table of the average equivalised income at municipality level in Lombardia, Toscana and Campania; semiparametric Fay-Herriot model 191 III Prologue This report contains the pilot applications based on the final small area developments of the WP2 partners in the SAMPLE project. The target of the report is to illustrate the use of the developed statistical methodology by means of applications to real data. The manuscript is organized in ten chapters. Chapters 1 and 2 introduce the Spanish and Italian data. They present a descriptions of the variables and data sources. Chapters 3 and 4 describe the applications to real data of the introduced area level time models with correlated or independent time effects and with different random effect variances for men and women. Chapters 5 and 6 deal with the prediction of poverty measures by using EB prediction or fast EB prediction under unit level models. Chapters 7 and 8 present EBLUP estimates of poverty measures based on Spatial Fay-Herriot models and Spatio-temporal Fay-Herriot models. Chapter 9 proposes to estimate poverty measures by aggregating data at a subdomain level and by applying unit level model to at that level. Chapter 10 reports a review of the M-quantile methodologies for small area estimation. Chapter 11 treats the problem of estimating Small Area Averages. It uses M-quantile models, non- parametric M-quantile models, M-quantile GWR models and semiparametric Fay and Herriot models. Chapter 12 presents some procedures to estimate the small area income distribution functions. They are based on M-quantile models and nonparametric M-quantile models. Chapter 13 gives an application to the estimation of poverty indicators by using M-quantile models. The manuscript has also a section of references and two appendixes with Tables of numerical results. This report has been coordinated by Domingo Morales (UMH). He has also been in charge of writing Chapters 1, 3, 4 and 9. Isabel Molina (UC3M) has been responsible for the elaboration of Chapters 5, 6 and 7. Chapter 8 has been produced in collaboration by the UMH and UC3M teams. Finally, Nikos Tzavidis (Southampton) and Monica Pratesi (UNIPI-DSMAE) have coordinated the production of the contents of Chapters 2, 10, 11, 12 and 13. 1 2 Chapter 1 The Spanish data 1.1 The Spanish Living Conditions Survey The Living Conditions Survey (LCS) is an annual survey with a rotating panel design with a sample formed by four independent subsamples, each of which is a four-year panel. Each year the sample is renewed in one of the panels. In order to select each subsample, a two-stage design is used with first stage unit stratification. The first stage is formed by census sections and the second stage by main family dwellings. Within these no sub-sampling is carried out, investigating all dwellings that are their usual residence. The framework used for the sample selection is an area framework formed by the relation of existing census sections used in the 2003 Municipal Register of Inhabitants. Second stage units use the list of main family dwellings in each of the sections selected for the sample.
Details
-
File Typepdf
-
Upload Time-
-
Content LanguagesEnglish
-
Upload UserAnonymous/Not logged-in
-
File Pages258 Page
-
File Size-