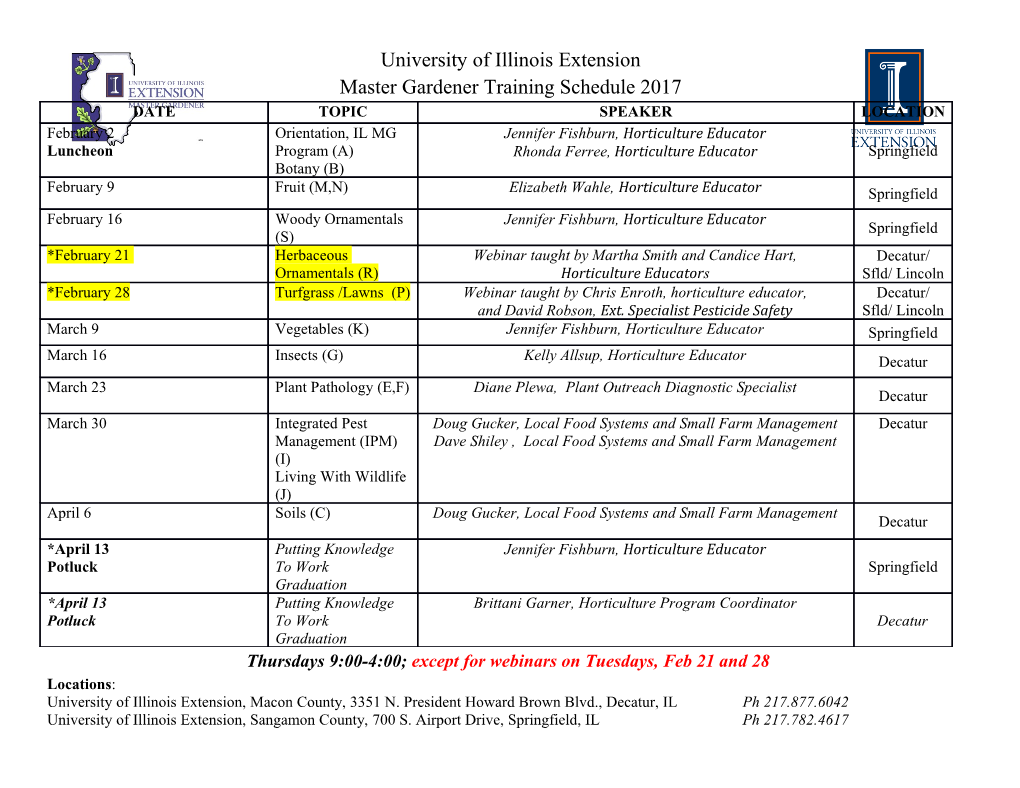
This document is downloaded from DR‑NTU (https://dr.ntu.edu.sg) Nanyang Technological University, Singapore. Transcriptome alterations of vascular smooth muscle cells in aortic wall of myocardial infarction patients Wongsurawat, Thidathip; Woo, Chin Cheng; Giannakakis, Antonis; Lin, Xiao Yun; Cheow, Esther Sok Hwee; Lee, Chuen Neng; Richards, Mark; Sze, Siu Kwan; Nookaew, Intawat; Sorokin, Vitaly; Kuznetsov, Vladimir Andreevich 2018 Wongsurawat, T., Woo, C. C., Giannakakis, A., Lin, X. Y., Cheow, E. S. H., Lee, C. N., et al. (2018). Transcriptome alterations of vascular smooth muscle cells in aortic wall of myocardial infarction patients. Data in Brief, 17, 1112‑1135. https://hdl.handle.net/10356/85590 https://doi.org/10.1016/j.dib.2018.01.108 © 2018 The Authors. Published by Elsevier Inc. This is an open access article under the CC BY license (http://creativecommons.org/licenses/by/4.0/). Downloaded on 09 Oct 2021 06:21:01 SGT Data in Brief 17 (2018) 1112–1135 Contents lists available at ScienceDirect Data in Brief journal homepage: www.elsevier.com/locate/dib Data Article Transcriptome alterations of vascular smooth muscle cells in aortic wall of myocardial infarction patients Thidathip Wongsurawat a,b, Chin Cheng Woo c, Antonis Giannakakis a, Xiao Yun Lin d, Esther Sok Hwee Cheow e, Chuen Neng Lee c,d, Mark Richards f,g, Siu Kwan Sze e, Intawat Nookaew b, Vladimir A. Kuznetsov a,h, Vitaly Sorokin c,d,⁎ a Department of Genome and Gene Expression Data Analysis, Bioinformatics Institute, Agency for Science, Technology and Research (A*STAR), Singapore 138671, Singapore b Department of Biomedical Informatics, College of Medicine, University of Arkansas for Medical Sciences, Little Rock, AR 72205, USA c Department of Surgery, Yong Loo Lin School of Medicine, National University of Singapore, Singapore 119228, Singapore d Department of Cardiac, Thoracic and Vascular Surgery, National University Heart Centre, Singapore, National University Health System, Singapore 119228, Singapore e School of Biological Sciences, Nanyang Technological University, Singapore 639798, Singapore f Cardiovascular Research Institute, National University Heart Centre, Singapore, 119228, Singapore g Department of Medicine, Yong Loo Lin School of Medicine, National University of Singapore, Singapore 119228, Singapore h School of Computer Science and Engineering, Nanyang Technological University, Singapore 639798, Singapore article info abstract Article history: This article contains further data and information from our pub- Received 23 January 2018 lished manuscript [1]. We aim to identify significant transcriptome Accepted 30 January 2018 alterations of vascular smooth muscle cells (VSMCs) in the aortic Available online 6 February 2018 wall of myocardial infarction (MI) patients. Microarray gene ana- lysis was applied to evaluate VSMCs of MI and non-MI patients. Prediction Analysis of Microarray (PAM) identified genes that sig- nificantly discriminated the two groups of samples. Incorporation of gene ontology (GO) identified a VSMCs-associated classifier that DOI of original article: https://doi.org/10.1016/j.atherosclerosis.2018.01.024 ⁎ Corresponding author at: Department of Surgery, Yong Loo Lin School of Medicine, National University of Singapore, Sin- gapore 119228, Singapore. E-mail address: [email protected] (V. Sorokin). https://doi.org/10.1016/j.dib.2018.01.108 2352-3409/& 2018 The Authors. Published by Elsevier Inc. This is an open access article under the CC BY license (http://creativecommons.org/licenses/by/4.0/). T. Wongsurawat et al. / Data in Brief 17 (2018) 1112–1135 1113 discriminated between the two groups of samples. Mass spectro- metry-based iTRAQ analysis revealed proteins significantly differ- entiating these two groups of samples. Ingenuity Pathway Analysis (IPA) revealed top pathways associated with hypoxia signaling in cardiovascular system. Enrichment analysis of these proteins sug- gested an activated pathway, and an integrated transcriptome- proteome pathway analysis revealed that it is the most implicated pathway. The intersection of the top candidate molecules from the transcriptome and proteome highlighted overexpression. & 2018 The Authors. Published by Elsevier Inc. This is an open access article under the CC BY license (http://creativecommons.org/licenses/by/4.0/). Specifications Table Subject area Biology More specific sub- Genomics, Proteomics, Bioinformatics, Cardiovascular ject area Type of data Tables, figures How data was Microarray (Gene Titan Instrument, Affymetrix), mass spectrometry (LC-MS/ acquired MS system comprised of a Dionex Ultimate 3000 RSLC nano-HPLC system, coupled to an online Q-Exactive hybrid quadrupole-Orbitrap mass spectro- meter (Thermo Scientific, Hudson, NH, USA)), RT-qPCR (QuantStudio™ 12K Flex system (Life Technologies; Thermo Fisher Scientific Inc, USA)) Data format Raw, analyzed Experimental Laser capture microdissection, total RNA extraction and protein extraction factors from aortic tissues from surgical patients Experimental Data analysis with Principal Component Analysis (PCA), Prediction Analysis features of Microarray (PAM), Gene Ontology (GO), Ingenuity Pathway Analysis (IPA) Data source Singapore location Data accessibility Data is with this article. Value of the data Combination of multiple technologies and bioinformatics analysis performed in this study reveals the molecular changes induced by myocardial infarction on aortic smooth cells in humans. The alterations of the VSMCs transcriptome are congruent with alterations at the protein levels. Both levels show notably the up-regulation of the superoxide dismutase (SOD) with the activation of superoxide radical degradation pathway. Differentially expressed genes and pathways identified in these comparisons may be used in future experiments investigating response in myocardial infarction. 1. Data 1.1. Clinical analysis The characteristics of the myocardial infarction (MI) and non-MI samples undergoing tran- scriptomics and proteomics studies are presented in Tables 1(A) and 1(B) respectively. The baseline demographic and clinical characteristics of samples undergoing transcriptomics study were 1114 T. Wongsurawat et al. / Data in Brief 17 (2018) 1112–1135 Table 1A Demographic characteristics of MI and non-MI groups undergoing transcriptomics analysis. Characteristics Transcriptomics Transcriptomics p-value MI Non-MI (n¼17) (n ¼19) Ethnic Chinese 12 10 0.557 Malay 2 6 Indian 2 2 Others 1 1 Gender Male 14 16 0.881 Female 3 3 Age (Mean 7 SD) 59.53 7 8.28 59.68 7 8.85 0.957 Ejection Fraction Good (445%) 11 13 0.292 Fair (30–45%) 4 6 Poor (o30%) 2 0 Smoking No 8 9 0.985 Yes 9 10 Renal Impairment No 15 19 0.124 Yes 2 0 Diabetes Mellitus No 9 7 0.332 Yes 8 12 Hypertension No 1 3 0.345 Yes 16 16 Hyperlipidaemia No 0 0 – Yes 17 19 Antihyperlipidemic Medication No 0 0 – Yes 17 19 Troponin I (µg/L) 12.20 7 20.86 0.01 7 0.004 o0.05 (Mean 7 SD) (n¼15) (n ¼4) Table 1B Demographic characteristics of MI and non-MI proteomics groups. Characteristics Proteomics Proteomics p-value MI Non-MI n¼25 n¼25 Ethnic Chinese 11 13 0.745 Malay 8 7 Indian 5 5 Others 1 0 Gender Male 20 18 0.508 Female 5 7 Age (Mean 7 SD) 60.88712.34 61.6878.26 0.789 Ejection Fraction Good (445%) 14 16 0.344 Fair (30–45%) 9 9 Poor (o30%) 2 0 Smoking No 12 12 1 Yes 13 13 Renal Impairment No 25 25 NA Yes 0 0 Diabetes Mellitus No 10 9 0.771 Yes 15 16 Hypertension No 3 1 0.297 Yes 22 24 Hyperlipidaemia No 1 0 0.312 Yes 24 25 Antihyperlipidemic Medication No 4 1 0.157 Yes 21 24 Troponin I (µg/L) 19.54 7 19.24 0.015 7 0.006 o0.05 (Mean 7 SD) (n¼22) (n¼9) T. Wongsurawat et al. / Data in Brief 17 (2018) 1112–1135 1115 Table 2 Comparative demographic characteristics of transcriptomics and proteomics groups. Characteristics Transcriptomics Proteomics p-value n¼36 n¼50 Ethnic Chinese 22 24 0.404 Malay 8 15 Indian 4 10 Others 2 1 Gender Male 30 38 0.41 Female 6 12 Age (Mean 7 SD) 59.61 7 8.47 61.28 7 10.40 0.431 Ejection Fraction Good (445%) 24 30 0.708 Fair (30–45%) 10 18 Poor (o30%) 2 2 Smoking No 17 24 0.943 Yes 19 26 Renal Impairment No 34 50 0.092 Yes 2 0 Diabetes Mellitus No 16 19 0.548 Yes 20 31 Hypertension No 4 4 0.624 Yes 32 46 Hyperlipidaemia No 0 1 0.393 Yes 36 49 Antihyperlipidemic Medication No 0 5 0.051 Yes 36 45 Troponin I (µg/L) 9.63 719.09 13.87 7 18.45 0.445 (Mean 7 SD) (n¼19) (n ¼31) compared with those of the samples from the proteomics study (Table 2). In addition, the char- acteristics of the transcriptomic MI and non-MI samples with those of the independent cohorts comprising additional MI and non-MI patients undergoing RT-qPCR were compared (Tables 3 and 4). 1.2. Gene expression data analysis and class prediction by Prediction Analysis of Microarray (PAM) The samples were preprocessed through several steps, including quality assessment and outlier identification, normalization, batch effect correction and evaluation (Fig. 1). To interrogate differen- tially expressed genes between MI and non-MI we conducted gene-expression profiling using the Affymetrix U219 microarray platform. The R ‘limma’ package (https://www.bioconductor.org/help/ workflows/arrays/) identified 4,357 probe sets, selected at a ‘limma’-defined p-value o 0.05. Based on this set of differentially expressed genes (DEGs), we performed principal component analysis (PCA) (Fig. 1). To determine subgroup of genes distinguishing MI from non-MI subjects, we performed super- vised PAM [2] and identified a set of differentially expressed genes (DEGs) that discriminated between the two subtypes at Wilcox FDR o 0.1 (Table 5). Gene Ontology (GO) analysis of the DEGs was performed using DAVID Bioinformatics tools [3] (http://david.abcc.ncifcrf.gov/).
Details
-
File Typepdf
-
Upload Time-
-
Content LanguagesEnglish
-
Upload UserAnonymous/Not logged-in
-
File Pages25 Page
-
File Size-