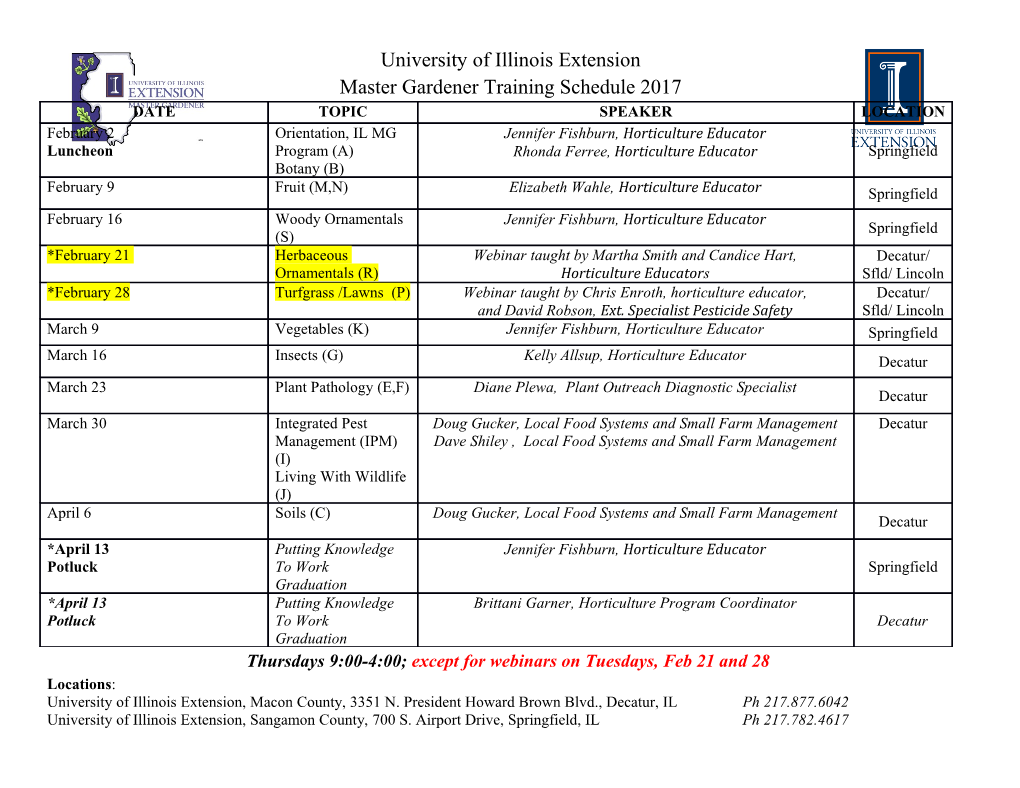
Lecture 11 Market selection in general equilibrium models II: Example when markets are incomplete. The GEI model • General equilibrium and market selection when markets are incomplete • Example that irrational traders are not necessarily wiped out by the market in these models • Details on blackboard • Blume/Easley [5] If You're So Smart Why Aren't You Rich? NB: many(!) (annoying) typos in their example 1 Lecture 12+13 Noise traders and market selection. A detailed discussion References The early years (here we get historical): • De Long, Shleifer, Summers, and Waldmann. “Noise Trader Risk in Financial Markets,” Journal of Political Economy, 98, 703-738, 1990. • De Long, Shleifer, Summers, and Waldmann. “The Survival of Noise Traders in Financial Markets,” Journal of Business, 64, 1-19, 1991. • Predecessors of agent-based models 2 MSH • Fama argued that irrational traders will be wiped out by market forces because they should lose money to the rational traders • Fisher Black (1986): excess returns comes from noise traders i.e. traders who trade on “information” that is, in fact, “noise” Model (OLG, investment decision only, 2 types) • 2 types: rational agents & noise traders • 2 assets: 1 risk-free ( supply), 1 risky (supply 1) both have same constant dividend r (genius at work!) • Agents maximize CARA utility fct [=>myopic mean-variance maximizers] • Rational = knowing true distribution of return • Noise traders = mis-perceive return (typically optimistic) • Additional volatility through noise traders = noise trader risk [in the paper’s parlance] • Noise traders take more risky positions for which they are compensated => Noise traders’ return can be higher! 3 Some more details • On blackboard Applications of this result (in the same paper): Volatility and Mean Reversion in Asset Prices Closed-end Mutual Funds Puzzle Equity Premium Puzzle Corporate Finance: Modigliani-Miller (the irrelevance theorem) Lecture 14 Complex adaptive systems: Trader types; Population and strategy choice dynamics; Price dynamics and stylized facts. 4 Main references • Cars Hommes. “Financial Markets as Nonlinear Adaptive Evolutionary Systems,” Quantitative Finance, 1, 149- 167, 2001. • Blake LeBaron. “A Builder's Guide to Agent-Based Financial Markets,” Quantitative Finance, 1, 254-261, 2001. • Both are survey-style papers with lots of references [the first is better IMHO]. • Thomas Lux. “Herd Behaviour, Bubbles and Crashes,” Economic Journal, 105, 881-896, 1995 • A lot of the work on this topic is computational Philosophy • View of financial markets as “evolutionary systems between different, competing trading strategies” (Cars Hommes) • Agents are boundedly rational: favor strategies that have done well in the past • In particular simple “technical” rules are permitted • Expectations or beliefs dynamics • Rational expectations eq. (REE) as benchmark • Heterogeneous population, difference of opinion, coexistence 5 Main ingredients • Rational traders: [why this term?] fundamentalists (smart money) • Noise traders: chartists, technical analysts (trend, pattern,…) • Belief dynamics (correlates with past performance) and/or trader type dynamics Adaptive belief systems • 1 riskless (infinite supply) & 1 risky asset (zero supply) • Myopic mean-variance maximizers with heterogeneous ‘beliefs’ about expected returns • Forecasting of tomorrow’s price [point estimate, e.g. trend (momentum, mean-reversion), fundamentalists (fund. value)] • Performance indicators: realized/risk-adjusted return • MAIN FOCUS: price dynamics and stylized facts • Minor role played by wealth dynamics (bankruptcy prevalent but not monitored) • Borrow a LOT form the noise trader approach discuss earlier 6 Adaptive belief systems: std model Dynamics between types • Performance of types induces flow between trader types (discrete-choice model with intensities) • Forecasting rules (for time lag =1) forecast * h h * h pt+1 − pt+1 = ft+1 = α ( pt − pt ) + β e.g. fundamentalist α = β = 0 trend follower α > 0, β = 0 biased belief α = 0, β ≠ 0 7 Dynamics of adaptive belief systems (Hommes, p.157+) Here forecasting depends on (discounted) past prices 8 Comparison with noise trader approach and criticism • Covers the standard noise trader model! • REE as benchmark [Is that reasonable?] • Restriction of trading strategy “universe”: too restrictive as lacks e.g. log-optimum investment 9 More comments • LeBaron’s “builder’s guide” survey • Market maker etc. • Price impact • Short-selling (“unrealistic” wealth dynamics) Contagion model for financial markets by Lux [only if time permits] Motivation • Self-organized process of ‘moods of the market’ • Optimism and pessimism as an infectious ‘disease’ [aka herd behavior] • Rooted in ‘psychologist’s attack’ on EMH 10 Model equations • 2N speculative traders • A trader is either optimist (n+) or pessimist (n-) n − n • Average opinion x = + − ∈[−1,+1] 2N • Change of mood probabilities p+− (x) [− → +], p−+ (x) [+ → −] • Change of average opinion dx = (1− x) p (x) + (1+ x) p (x) dt +− −+ Specification of functions p+− (x) = v exp(ax), p−+ (x) = vexp(−ax) • Resulting differential eq. for average opinion has the following fixed points (steady states) If a ≤ 1, x=0 (stable) If a > 1, x=0 (unstable) and two stable x- < 0, x+ 0 [ = - x-] > 0 • Graph on next slide 11 Graph Prices via demand and supply • Net excess demand of traders (each can trade tN units) DN = n+tN − n−tN = x(2NtN ) =: xTN • Only zero (i.e. market clears) if x=0! • Add additional actors(!): Fundamentalists DF = TF ( p f − p) • “Market-maker” induces price adjustment process (though not market clearing in any point in time) dp = β (D + D ) = β[xT +T ( p − p)] dt N F N F f 12 Final model • “Combining the contagion and price dynamics it seems reasonable to include a feedback effect from the price change on the disposition of speculators” (Lux, p.887) p+− (x) = vexp(a1(dp / dt) / v + a2 x), p−+ (x) = v exp(−a1(dp / dt) / v − a2 x) • Now we have a 2-dimensional system Graphs 13 Bear/Bull switching model • Add ‘basic mood’ of the market a0 p+− (x) = v exp(a0 + a1(dp / dt) / v + a2 x), p−+ (x) = vexp(−a0 − a1(dp / dt) / v − a2 x) da 0 =τ[(r +τ −1(dp / dt)) / p − r / p ] dt f • plus instantaneous market clearing dp = (T /T ) dx / dt dt N F 14 Lecture 15 An evolutionary stock market model I: Model. Model basics • I investors/strategies [‘fund’ remark] • K long-lived assets (stocks), each in supply 1 • Payoff is perishable consumption good [Lucas 1978] K A (s) > 0 Ak (s) ≥ 0, k=1 k • Strategies (simple) in simplex of dimension K-1 • Time is discrete • S states of nature (i.i.d. for simplicity) • Common savings rate [consumption rate 1- ] 15 Evolutionary Finance - understanding the dynamics of financial markets - K i i wt+1 = (Ak (st+1 ) + pt+1,k )θt,k k =1 i i I λt,k wt p = ρ λ j w j t+1,k j=1 t+1,k t+1 pt,k Similarities and differences to short-lived asset model • Investment strategy = budget shares • K+1 markets (K assets, 1 consumption good) • Short-term (temporary) equilibrium • Main innovation Resale value => capital gains • Asset market applications are now within reach • Role of consumption (not needed previously) 16 Solving the model On blackboard Steps • Account for consumption • Determine total wealth => relative wealth (wealth shares) • Dealing with the equilibrium problem => map with state [random dividend] • Verifying that everything is correct Lecture 16 An evolutionary stock market model II: Results. 17 Assumptions Stationarity relative dividends and strategies stationary Definition λ∗-market is evolutionary stable if • no strategy can gain against incumbent λ∗ • any µ ≠ λ∗ loses against some mutant strategy I = 2: Inverse matrix tractable! (Note: similar to Evol Game Th.) Local Stability - linearization and the variational equation - Growth rate at fixed point (1,0) » K 1 t+1 ÿ i (1− ρ) Rk (st+1 ) + ρ λk (s ) i t i rt+1 ≈ …ƒ 1 t λk (s )Ÿ rt k =1 λk (s ) ⁄ » K t+1 (1− ρ ) Rk (st+1 ) + ρ λk (s ) t g(µ,λ) = E ln…ƒ t µk (s ) k =1 λk (s ) Growth rate of investor )‘s share at investor *s prices 18 Question Is there a λ * ( s t ) such that for allµ ≠ λ* (1) g λ * ( µ ) < 0 and for some t ? (2) gµ (λ) > 0 λ(s ) » K t+1 (1− ρ ) Rk (st+1 ) + ρ λk (s ) t gλ (µ) = E ln…ƒ t µk (s ) k =1 λk (s ) Result (finitely many states) IID case * t * λk (s ) =λk = ERk Markov case 1− ρ ∞ [λ* (s)]s = ρ m[π m R] k k ƒ ρ m=1 19 0ketch of Proof: IID case 1/4 (1) Why is * stable? λk = ERk ~ 0 gλ* (µ) = — g(...) dP *))))))S Ν +)))))), » K * ρ λk + (1− ρ) Rk (s) 0 ƒπ s ln…ƒ * µk (s ) s∈S k=1 λk Sketch of Proof: IID case 2/4 » K ≈ ’ ÿ ∆ Rk (s) ÷ 0 = ƒπ s ln…ƒ ∆ ρ + (1− ρ) ÷ µk (s )Ÿ s∈S k =1 « ERk ◊ ⁄ 0 K (Strictly) concave inµ(s ) ∈∆ Maximum at µ ( s 0 ) = λ * , and value = 0 there 20 0ketch of Proof: IID case 3/4 (2) Why is µ ≠ λ * unstable? * ~ 0 gµ (λ ) = — g(...) dP *))))))+S Ν)))))), » K ρ µ (s0 , s) + (1− ρ) R (s) ƒπ ln…ƒ k k λ* s µ (s0 ) k &)s∈)S ))' k =)1 )))( k &))'))( K » 0 ÿ ≥ 0, and > 0 if (s0 ) ≠ * 0 * µk (s ) —...dP ≥ ρ Eƒλk ln… 0 Ÿ = 0 k=1 µk (s , s)⁄ 0ketch of Proof: IID case 4/4 » K 0 ÿ » K * * µk (s ) λk ρƒπ s ln…ƒ λk 0 Ÿ + (1− ρ)ƒπ s ln…ƒ Rk (s) 0 s∈S k=1 µk (s , s) ⁄ s∈S k=1 µk (s ) &))))'))))( &))'))( ≥ 0, and > 0 if (s0 ) ≠ * K » 0 ÿ 0 * µk (s ) —...dP ≥ ρ Eƒλk ln… 0 Ÿ = 0 k=1 µk (s , s)⁄ 21 Findings 8nly a λ *-market is evolutionary stable In this stationary market assets are priced at “fundamental values“ (relative dividends) Market selection favors value investors Only λ * strategy is growth-optimal @ own prices Kelly rule for assets instead of states Fundamental value without short sales Global selection results • Evstigneev/Hens/Schenk-Hoppé Working paper NCCR 09/2005 i.i.d.
Details
-
File Typepdf
-
Upload Time-
-
Content LanguagesEnglish
-
Upload UserAnonymous/Not logged-in
-
File Pages44 Page
-
File Size-