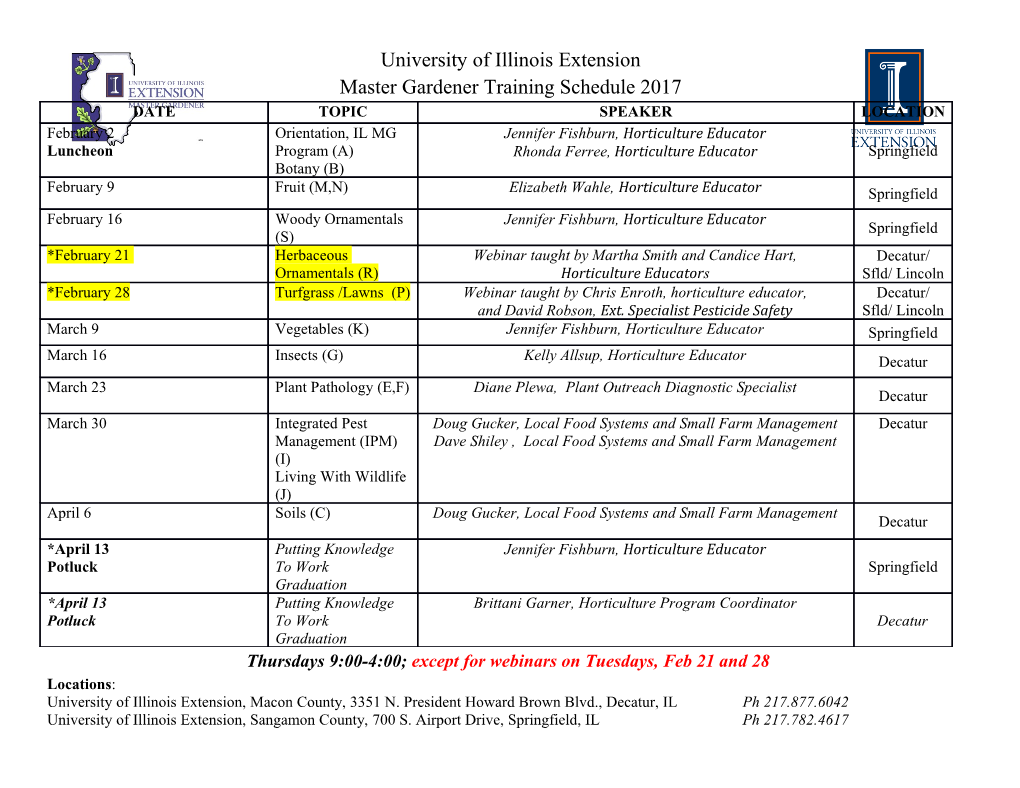
Efficient SNP based Heritability Estimation and Multiple Phenotype-Genotype Association Analysis in Large Scale Cohort studies A DISSERTATION SUBMITTED TO THE FACULTY OF THE GRADUATE SCHOOL OF THE UNIVERSITY OF MINNESOTA BY SOUVIK SEAL IN PARTIAL FULFILLMENT OF THE REQUIREMENTS FOR THE DEGREE OF DOCTOR OF PHILOSOPHY Advised by Dr. Saonli Basu August, 2020 c SOUVIK SEAL 2020 ALL RIGHTS RESERVED Acknowledgements There are many people that I am grateful to for their contribution to my time in graduate school. I would like to thank Dr. Saonli Basu for mentoring me through past few years. Thank you for introducing me to challenging datasets and relevant problems in the world of Statistical Genetics. I would like to thank Dr. Abhirup Datta from Johns Hopkins University for guiding me in my most recent work described in chapter 3. Thank you for exposing me to Spatial Statistics, a branch which was entirely new to me. I would also like to thank Dr. Matt McGue for introducing me to the Minnesota Center for Twins and Family (MCTFR) Study, which I have used in two of my projects. I am thankful to all my teachers whose classes have broadened my biostatistical intellect and greatly helped in my research. I am grateful to my parents Mr. Sanjay Seal and Mrs. Srabani Seal and my girlfriend Manjari Das from Carnegie Mellon University for their incredible support throughout past four years. Manjari, special thanks to you not only for your brilliant ideas from time to time that have helped me in advancing my research but also for helping me get through tough times. Finally, I would like to thank Dr. Saurabh Ghosh and Dr. Kiranmoy Das from Indian Statistical Institute, Kolkata for motivating me to join the Biostatistics PhD program at the University of Minnesota. i Dedication The thesis is dedicated to my grandmother Rani Seal whose company I dearly miss everyday. You and parents have been pivotal in shaping my life. ii Abstract Recent developments in genotyping technologies have opened up many a new pos- sibilities of unravelling the genetic basis of common diseases. The past decade has seen an advent of a bunch of large scale cohort studies giving us, the re- searchers, access to an unprecedented wealth of data providing information on millions of genetic variants and numerous diseases/traits on millions of individu- als. But, efficient analysis of such high-dimensional data demands non-traditional yet novel statistical techniques. The development of a complex human disease is an intricate interplay of genetic and environmental factors. In order to better understand such traits, we are often interested in estimating the overall trait heri- tability: the proportion of total trait variance due to genetic factors within a given population. Accurate estimation and inference of heritability gives us some basic understanding of disease risk and etiology. Traits with high estimated heritabil- ity incite interest among the researchers for a further Genome-Wide Association Study (GWAS) to pinpoint the significant genetic variants. As we move into the era of genome editing and personalized medicine, addressing the shared genetic basis of multiple diseases/traits or the genetic basis of a single disease/trait over multiple time-points becomes more and more important. In light of these exciting statistical problems, my thesis focuses on developing robust tools for estimating heritability and performing GWAS in large scale cohort studies both in a univari- ate and multivariate context. iii Contents Acknowledgements i Dedication ii Abstract iii List of Tables vii List of Figures ix 1 Introduction 1 2 Heritability Estimation and Genetic Association Testing in Lon- gitudinal Twins Study 8 2.1 Introduction . 8 2.2 Materials and Methods . 11 2.2.1 Cross-sectional Family Study . 11 2.2.2 Existing Methods for longitudinal Twins Study . 13 2.2.3 Proposed model . 16 2.3 Estimation in RMFM and MFM . 20 2.3.1 RMFM . 20 iv 2.3.2 MFM Method of Moments (MFM-MOM) . 23 2.4 Results . 25 2.4.1 Comparing heritability in simulation setup by different ap- proaches . 25 2.4.2 Univariate heritabilities in Real Data by different approaches 30 2.5 Discussion . 31 3 Efficient SNP-based Heritability estimation using Gaussian Pre- dictive Process 33 3.1 Introduction . 33 3.2 Materials and Methods . 35 3.2.1 Genome-based Restricted Maximum Likelihood Approach 35 3.2.2 Proposed Method . 37 3.3 Results . 43 3.3.1 Simulation using Coalescent Theory . 43 3.3.2 Simulation using UK Biobank data . 45 3.3.3 Analysis of real UK Biobank traits . 55 3.4 Time Comparison . 57 3.5 Discussion . 59 4 Multivariate Association Analysis of Correlated Traits in Related Individuals 61 4.1 Introduction . 61 4.2 Material and Method . 64 4.2.1 Existing Methods . 65 4.2.2 Proposed Method . 72 4.3 Results . 75 v 4.3.1 Simulation Study . 75 4.3.2 Real Data Analysis . 83 4.4 Discussion . 87 References 89 Appendix A. 102 A.1 Calculating variance of MFM-MOM heritability estimate . 102 A.1.1 Theorems . 106 Appendix B. 110 B.1 Additional Figures . 110 B.1.1 Simulation from section 3.3.2.1 . 111 B.1.2 Simulation from section 3.3.2.2 . 112 B.1.3 Simulation from section 3.3.2.3 . 114 Appendix C. 116 C.1 Positive semi-definiteness of RMultiPAR's covariance assumption 116 C.2 Comparing the assumptions of RMultiPAR with the traditional approach . 117 C.3 Development of Adjusted RMultiPAR . 119 C.4 Discussion about Adjusted RMultiPAR . 123 C.5 Simulation with more number of traits . 127 C.5.1 Manhattan Plots . 128 vi List of Tables 2.1 The table compares the computational time of fitting RMFM using Optim in R with the proposed two stage approach of fitting RMFM and also the MFM-MOM, in seconds. Under the simulation setup described in section 2.4, each of the methods, were run 100 times and their minimum, maximum and mean values were listed. 25 2.2 Mean of the univariate heritabilities by OpenMx . 26 2 2.3 Univariate Heritabilities (hk) in Real Data . 30 3.1 Mean comparison of different methods for two cases: Case (1) and Case (2) with true h2 = 0:8 ..................... 45 3.2 Mean (Variance) comparison of GREML (sub) and PredLMM with different subsample sizes with true h2 = 0:7 and 40,000 individuals 48 3.3 Mean (Variance) comparison of GREML (sub) and PredLMM with different subsample sizes under case (a) with true h2 = 0:7: . 51 3.4 Mean (Variance) comparison of GREML (sub) and PredLMM with different subsample sizes under case (b) with true h2 = 0:2: . 51 3.5 Mean (Variance) comparison of GREML (sub) and PredLMM with different subsample sizes under case (a) with true h2 = 0:6: . 54 3.6 Mean (Variance) comparison of GREML (sub) and PredLMM with different subsample sizes under case (b) with true h2 = 0:2: . 54 vii 3.7 Time comparison of different methods in seconds for the simulation from section (3.3.1) with 5k (8k SNPs) and 8k (13k SNPs) individuals. 57 3.8 Time comparison of PredLMM in minutes for varying different knot (subsample) sizes with Bolt-REML under the simulation from sec- tion (3.3.2.3) . 58 4.1 The table lists the common SNPs detected by all three methods: RMultiPAR, PCA and MinP at p-value threshold of 1 × 10−8. 85 4.2 The table lists the SNPs detected only by RMultiPAR at p-value threshold of 1 × 10−8.......................... 86 C.1 The table lists the mean (and sd in the bracket) of the values the p p function f takes in the interval ((1− τ)2; (1+ τ)2) for datapoints separated by 0.002. 126 viii List of Figures 2.1 Comparing univariate heritabilities obtained by three different meth- ods. Shows that the estimates of the heritabilities are biased from the true value 0.8 in case of OpenMx. 26 2.2 Histograms of the univariate heritabilities obtained by marginal ACE models . 28 2.3 Histograms of the univariate heritabilities obtained by MFM-MOM 28 2.4 Histogram of the multivariate heritability by marginal ACE models 29 2.5 Histogram of the multivariate heritabilities obtained by MFM-MOM 29 3.1 The figure compares MSE of different methods for case (1) and (2). 44 3.2 The figure plots the pairwise principal components of the genetic data of the individuals from the UK Biobank cohort along with their self-reported ancestries. 46 3.3 The figure compares MSE of GREML (sub) and PredLMM for three different subsample (knot) sizes, 4000, 8000 and 16,000. 48 3.4 The figure compares MSE of GREML (sub) and PredLMM for five different subsample sizes under case (a) (top) and case (b) (bottom). 50 3.5 The figure compares MSE of GREML (sub) and PredLMM for five different subsample sizes for case (a) (top) and case (b) (bottom). 53 ix 3.6 The figure shows barplot of the heritability estimates by two meth- ods with different subsample sizes. 56 4.1 The plot shows the type 1 error and power of the methods under the simulation setup of the section (4.3.1.1). 77 4.2 The plot compares the type 1 error and power of different methods under the simulation setup of the section (4.3.1.2) for three different values of ρ at level 0.05 . 79 4.3 The histogram of RMMLR test statistic for the case of ρ = 0:5 (on the left) and ρ = 0:8 (on the right) in section (4.3.1.3) .
Details
-
File Typepdf
-
Upload Time-
-
Content LanguagesEnglish
-
Upload UserAnonymous/Not logged-in
-
File Pages141 Page
-
File Size-