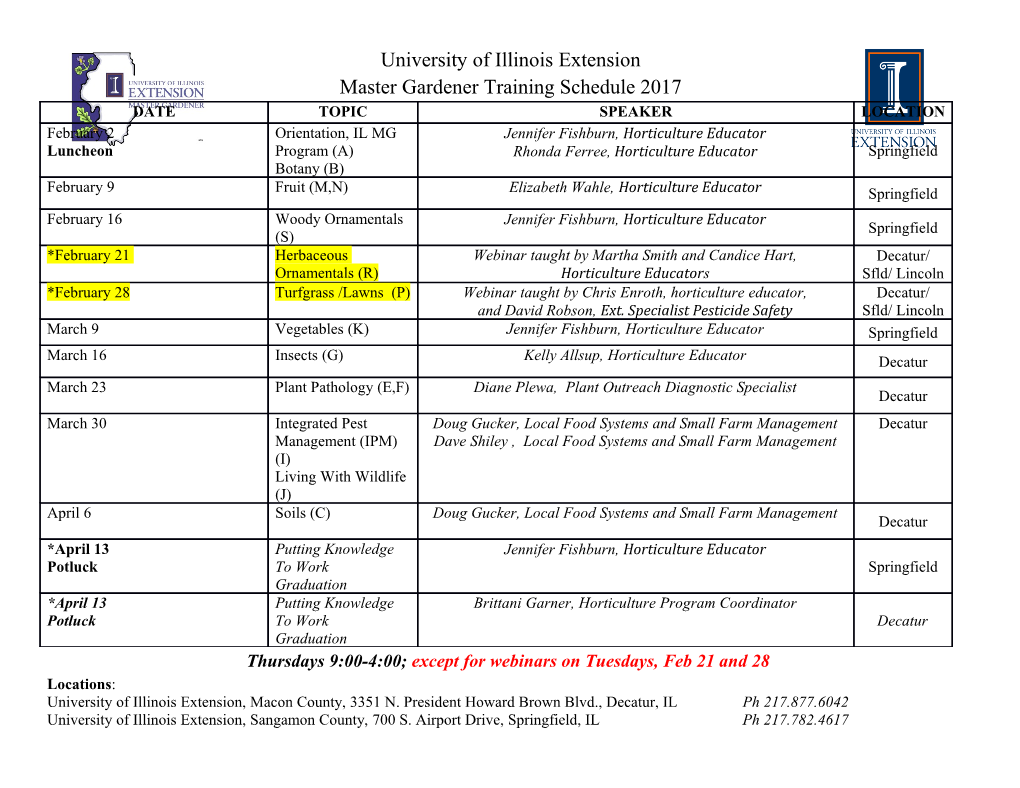
ISSN: 2574-1241 Volume 5- Issue 4: 2018 DOI: 10.26717/BJSTR.2018.06.001398 Robert Skopec. Biomed J Sci & Tech Res Mini Review Open Access Brain as a Anti-Entropic Intelligence Generator Robert Skopec* Researcher-Analyst, Slovakia Received: June 27, 2018; Published: July 11, 2018 *Corresponding author: : Robert Skopec, Analyst-Researcher, Dubnik, Slovakia Abstract There are several spheres of underdevelopment in recent brain research. Brain research (BR) is too oriented to macro-states findings, ignoring the process of reentry between macro-states and micro-states, especially is not reflecting the macro-states influence on internal representations at micro level (links to quantum physics). There is a tendency to empirical isolationism, unilateral concentration only to the system, not studying equally the mechanisms of interplay between system and the environment. We are underestimating the informational impact of a probability factor to the brains behavior. Mathematical approach as a pure method of the artificial intelligence is not enough implemented, despite this, we believe with A. Einstein, that the mathematics is a language of God. Introduction has a set of polar decompositions: Γ=Σkqkϕφ k ⊗ k with the qk The consciousness is a mathematical structure, with q= () qk k complex. They are parametrized by the right toroid T of amplitudes neurobiological semantics. The higher mental processes all state space of U = V+W. The evolution of q is determined via the and comprise a singular bundle over S, the enlarged correspond to well-defined but, at present, poorly understood information processing functions that are carried out by physical (pp12 , ....) connection on this bundle. Each fieber T (axons, dendrites) has systems, our brains[1].The consciousness is the result of a global a unique natural convex partition yielding the correct workspace (GW) in the brain, which distributes information to Γ , and intersects p j in an arc of probabilities,2 since the circle of unit vectors which generate the the huge number of paralell unconscious processors forming q j . In this interpretation, rays in Hilbert space correspond ray corresponding to the state of a rest of the brain. The GW is composed of many different paralell to ensembles, and unit vectors in a ray correspond to individual on the symbolic representations that it receives as input [2]. The length processors, or modules, each capable of performing some task Γ above vector Γ in the unit sphere S of Η member plane waves of such ensemble. An element of the fieber moduls are flexible in that they can combine to form new processors component processors [3]. Ρ . This fieber can be capable of performing novel tasks, and can decompose into smaller polar decomposition of Γ at synapse: thought as an information carrier of the set all the possible complex The Cortex as a Prediction Generator ∑ Γ=qk Γ k k where the qk ∈ C , and the Γk are bi – orthonormal. Thus each Γk consist from two modular subsystems: Global workspace system at the neurobiological level may is of the form ϕφkk⊗ where the φk and theϕk are orthonormal [6]. a) S1 ,composed of a net of parallel, distributed and functionally The first is a processing network of the coputational space workspace S 2 specialised processors (from primary sensory processors The second is the projective modular system of a global – area V1, unimodal processors – area V4, heteromodal , consisting of a set of cortical neurons characterized processors – the resonant mirror neurons in area F5) [4]. by ability to receive from and send back to homologous neurons in other cortical areas horizontal projections through long-range excitatory axons [7]. The populations of neurons are distributed They are processing categorical, semantic information. The among brain areas in variable proportions. It is known that reality is a theory arising from interferrence of computed representation. The interferrence product is therefore “true” long-range cortico-cortical tangential connections, and callosal inputs compared operatinally in GW with a resonant pre- [5]. connections originate from the pyramidal cells of layers 2 and 3, If V is a physical system in interaction with another system W. which give or receive the so called “association“ efferents and afferents [8]. These cortical neurons establish strong vertical and Kochen´s standard state vector Γ(t) reciprocal connections, via layer 5 neurons, with corresponding Biomedical Journal of of the two interacting systems thalamic nuclei, contributing to the direct access to the processing Scientific & Technical Research (BJSTR) 5454 Biomedical Journal of Scientific & Technical Research Volume 6- Issue 4: 2018 input feature X j takes value x is Cc= networks. These projections may selectively amplify, or extinquish interconnected in a way, that only one workspace representation given the value of the class label inputs from processing neurons. The entire workspace is globally [14]. The feature selection filter in the perception can be information between the class variable and each attribute variable used the naïve Bayesian classifier based on the empirical mutual Thecan be Predictor active in a given time [2]. [15]. The mutual information is computedP( X jduring= xC, = c)the learning The projective system (PS) is involved in the main computational MI( xjj , c )=∑∑ P ( x = x , C = c ) log process learning. xc PX( j = xPC) ( = c) a a To predict the value of ry r of a particular part of the brain: the predictive metalearning system of has active user a neuromodulator circuits wthin the circuits of basal ganglia, which given the profile For prediction functions are important also the connections of we apply a slightly modifiedV prediction rulea to allow been suggested [9] as major locus of reinforcement learning. a ∂(rwj ,) ry= arg max PR( z= v) PR( jy= wR = v) for missing values. Thev prediction rule is . j≠= yw1 prefrontal cortex, thalamus, cerebellum, etc. In a computational ödel has pointed out, that predictions are like a perception theory on the roles of the ascending neuromodulatory systems, of the objects of set theory. Prediction is a mode of mathematical Kurt G they are mediating the global signals regulating the distributed learning mechanism in the brain [10]. Dopamine represents the intuition, which in sense of perception induces building up theories global learning signal for prediction of rewards and reinforcement to the abstract elements containd in our empirical ideas. The of the future. The given underlying mathematics is closely related of actions. The reinforcement learning in this computational brain seems to have internal theories about what the world is framework is for an agent to take an action in response to the state like. Between [6] brains theories is a internal perceptual rivalry in of environment to be acquired reward maximised in a long run. Darwinian sense. The World as a quantum system can be described A basic algorithm of reinforcement learning could be St( )∈ ( s1 ,..... sn ) considered a Markov decision problem (MDP) assuming a discrete due the polar decomposition, as a whole system consisting from ,takes action at( )∈ ( a1 ,..... an ) state, action and time. The agent observes the state deterministically as a= Gs(),or stochastically P= () as . In response two subsystems, which are mutually observing one another. according to its policy, given either at() During this observation the global workspace is processing reentry between internal representations and influence functional of the deterministically as st()= Fst ((),()) at j th of elements subset to the agent´s action , the state of environment changes either k Pst( (+ 1) st ( ) , at() for each action at(). environment,k between left and right hemisphere, etc [3]. Some ()X j taken from isolated neural system X, and its complement , or stochastically according authors are proposing to consider a The reward +∈ is deterministically rt(+= 1) Rst ( ( ), at ( )) , or XX− k to a Markov transitionrt( 1) matrix R j stochastically Prt( (+ 1) st ( ) , at( )) and the rest of the system introduce statistical dependence (Edelman, 1998)[R]. Interactions between the subset between the two. This is measured by their mutual information ,[11,12] In this setup, the goal of kk k k MIX(jj , X−= X ) H (( X j ) + HX ( −− X j ) HX ( ) reinforcement learning is to find an optimal policy maximising the k k which the entropy of X j is accounted for by the entropy of XX− j expected sum of future rewards. The architecture for reinforcement ,which captures the extent to to predict future rewards in the form of a state value function Vs() and vice versa (H indicates statistical entropy) [16]. learning is the actor-critic, consisting of two parts: The critic learns for the current policy, The actor improves the policy = P() as Learning . in reference to the future reward predicted by the critic. The actor and the critic learn either alternately, or concurrently. The Signalling between neuronal groups occurs via excitatory synaptic weights between two excitatory units are assumed to connections linking cortical areas, usually in a reciprocal fashion. ∆=wpost, pre ε RSpre(2 S Post − 1) be modifiable according to a reward-modulated Hebbian rule According to the theory of the neuronal group slection, selective each network response (R=+1, correct, R = -1, incorrect), pre is the reciprocal connections in a proces called „reentry“ [17]. Reentrant , where R is reward signal provided after dynamical links are formed between distant neuronal groups via presynaptic unit and post the postsynaptic unit [2]. The reward signalling establishes correlations between cortical maps, within than feedback in both connectivity and function in that is not simply signal R influences the stability of workspace activity through or between different levels of the nervous system. It is more general ∆=−wwpost,, pre 0.2(1post pre ) , where w´ is a short-term multiplier on used for error correction or cybernetic control in the classical a short-term depression or potentiation of synaptic weights: sense.
Details
-
File Typepdf
-
Upload Time-
-
Content LanguagesEnglish
-
Upload UserAnonymous/Not logged-in
-
File Pages13 Page
-
File Size-