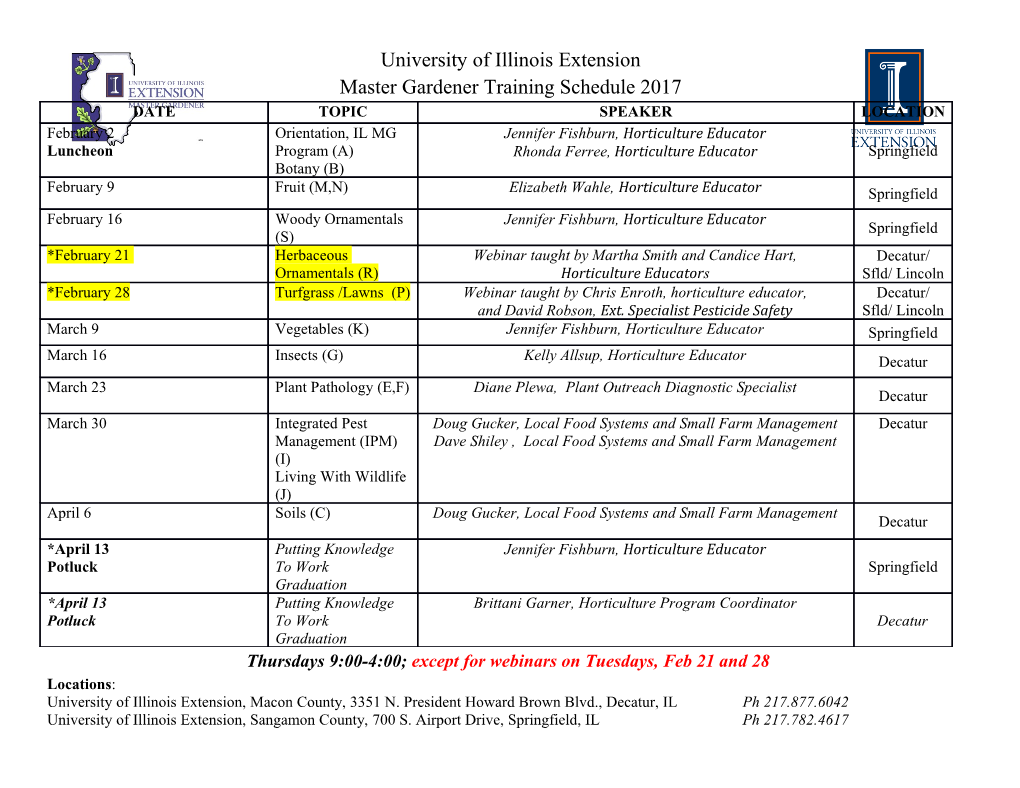
Distinct Molecular Mechanisms Analysis of Obesity Based on Gene Expression Proles Harish Joshi Endocrine and Diabetes Care Center, Hubbali, 580029, Karanataka, India Basavaraj Mallikarjunayya Vastrad SET`S College Of Pharmacy, SR Nagar, Dharwad, 580002, Karanataka, India Nidhi Joshi Dr D Y Patil Medical College, Kolhapur, 416006, Maharastra, India Chanabasayya Mallikarjunayya Vastrad ( [email protected] ) Chanabasava Nilaya https://orcid.org/0000-0003-3615-4450 Research Keywords: adiposities, obesity, differentially expressed genes, modules, protein–protein interaction network Posted Date: October 22nd, 2020 DOI: https://doi.org/10.21203/rs.3.rs-95029/v1 License: This work is licensed under a Creative Commons Attribution 4.0 International License. Read Full License Page 1/98 Abstract Background Obesity is the most common metabolic disorder worldwide. Its progression rate has remained high in recent years. Objectives Therefore, the aim of this study was to diagnose important differentially expressed genes (DEGs) associated in its development, which may be used as novel biomarkers or potential therapeutic targets for obesity. Methods The gene expression prole of E-MTAB-6728 was downloaded from the database. After screening DEGs in each ArrayExpress dataset, we further used the robust rank aggregation method to diagnose 876 signicant DEGs including 438 up regulated and 438 down regulated genes. Pathway enrichment analyses and Gene Ontology (GO) were performed by online tool ToppCluster. These DEGs were shown to be signicantly enriched in different obesity related pathways and GO functions. Then, the mentha, miRNet and NetworkAnalyst databases were used to construct the protein–protein interaction network, target genes - miRNA regulatory network and target genes - TF regulatory network. The module analysis was performed by the PEWCC1 plug‐in of Cytoscape based on the whole PPI network. Results We nally ltered out HSPA8, ESR1, YWHAH, RPL14, SOD2, BTG2, LYZ and EFNA1 hub genes. Hub genes were validated by ICH analysis, Receiver operating curve (ROC) analysis and RT-PCR. The robust DEGs linked with the development of obesity were screened through the ArrayExpress database, and integrated bioinformatics analysis was conducted. Conclusions Our study provides reliable molecular biomarkers for screening and diagnosis, prognosis as well as novel therapeutic targets for obesity. Introduction Obesity comes under metabolic disorder and is now so common in global population [1]. Obesity occurs in children age between 5 to 19 years as well as more common in women than in men [2]. It is linked with pathogenesis of many disorders such as heart disease [3], hyperlipidaemia [4], hyperinsulinaemia [5], hypertension [6], atherosclerosis [7], insulin resistance [8] and cancer [9]. Important candidate genes and relevant signaling pathways linked with obesity remains largely unknown. As a result, seek of an earlier diagnosis and better prognosis, deeper understanding of genetic and molecular mechanisms about obesity is necessary. Accumulative evidence suggested that many genes and signaling pathways participate in obesity progression. Polymorphisms in UCP2 and UCP3 were responsible for development of obesity [10]. TNFα and lipoprotein lipase were important for advancement of obesity [11]. SLC6A14 [12] and JHDM2A [13] were lined with pathogenesis of obesity. Human salivary (AMY1) and pancreatic (AMY2) amylase genes were diagnosed with growth of obesity [14]. Signaling pathways such as inammatory signaling pathway [15], TLR4 signaling pathway [16], calcineurin-dependent signaling pathways [17], mTOR Complex1–S6K1 signaling pathway [18] and leptin-signaling pathway [19] were important for development of obesity. Therefore, it is key to understand the molecular mechanisms associated in development of obesity, which facilitates effective diagnosis, prognosis and treatment. However, the essential genes of development of obesity remain largely unknown. In present trends, the application of high-throughput analysis in gene expression is becoming more valuable in clinical and medical research [20], molecular classication [21], prognosis prediction [22], diagnoses [23] and new targeted drug discovery [24]. In this study, the original microarray data (E-MTAB-6728) was downloaded from ArrayExpress. The gene expression proles of adipocytes from obese patients were extracted and compared with those in adipocytes from lean persons (controls) to identify the differently expressed genes (DEGs). Subsequently, the DEGs were screened with the application of limma (http://www.bioconductor.org/packages/release/bioc/html/limma.html) followed by gene ontology (GO), pathway enrichment analysis using different pathway databases, Protein–protein interaction network construction and analysis, module analysis, target gene - miRNA interaction network construction and analysis, and target gene - TF interaction network construction and analysis. The results provide information about the key pathways and genes that will contribute to further research on the diagnosis, prognosis and treatment for obesity. Materials And Methods Microarray Data The microarray expression prole of E-MTAB-6728 was downloaded from ArrayExpress (https://www.ebi.ac.uk/). E-MTAB-6728 was based on A-MEXP-1171 - Illumina HumanHT-12 v3.0 Expression BeadChip and was submitted by Bjune et al [25]. The E-MTAB-6728 dataset about expression of genes from adipocytes from obese patients compared to adipocytes from lean persons (controls). There are twenty-four samples including twelve adipocytes from obese patients and twelve adipocytes from lean persons (controls). The overall design of the experiment was microarray analysis of adiposities from obese patients versus adipocytes from lean persons (controls). Page 2/98 Identication of DEGs between obese patients and lean persons The raw data les were acquired for the analysis as IDAT les (Illumina platform) forms and were converted into gene symbols and then processed to background correction and quartile data normalization using the effective multiarray average algorithm in the beadarray package. The analysis was carried out via R software (version 3.5.2). Hierarchical clustering analysis was applied to categorize the samples into two groups with similar expression patterns in twelve adiposities from obese patients and twelve adipocytes from lean persons (controls). The paired Student’s t-test based on the Limma package in R was used to diagnose DEGs between two experimental conditions. Multiple testing correction was performed by the Benjamini–Hochberg method to obtain the FDR (false discovery rate) value. Then, the Log2 Fold change (log2FC) was determined. DEGs with an FDR < 0.05 and | log2FC | ≥ 0.5 were considered to be statistically signicant. Pathway enrichment analysis of DEGs To further analyses the potential pathway of the overlapping DEGs, the online software ToppCluster (https://toppcluster.cchmc.org/) [26] which integrates different pathway data bases such as BIOCYC (https://biocyc.org/) [27], Kyoto Encyclopedia of Genes and Genomes (KEGG) (http://www.genome.jp/kegg/pathway.html) [28], Pathway Interaction Database (PID) (https://wiki.nci.nih.gov/pages/viewpage.action?pageId=315491760) [29], REACTOME (https://reactome.org/) [30], GenMAPP (http://www.genmapp.org/) [31], MSigDB C2 BIOCARTA (v6.0) (http://software.broadinstitute.org/gsea/msigdb/collections.jsp) [32], PantherDB (http://www.pantherdb.org/) [33], Pathway Ontology (http://www.obofoundry.org/ontology/pw.html) [34] and Small Molecule Pathway Database (SMPDB) (http://smpdb.ca/) [35] were used to perform pathway enrichment analysis. P<0.05 and counts >2 were set as the threshold values. Gene ontology (GO) enrichment analysis of DEGs The DEGs (up and down regulated) were subjected to GO (http://www.geneontology.org/) [36] enrichment analysis. The GO analysis having terms under the three categories such as cellular component (CC), molecular function (MF) and biological process (BP). GO enrichment analyses were performed using ToppCluster (https://toppcluster.cchmc.org/) [26] online tool. The cut-off value for a signicant GO term and pathway was set to p-value<0.05 and count ≥2. Integration of PPI network and module analysis Online tool mentha (https://mentha.uniroma2.it/) [37] is the popular online tool which integrates different PPI databases such as The Molecular INTeraction Database (MINT, https://mint.bio.uniroma2.it/) [38], IntAc (https://www.ebi.ac.uk/intact/) [39], Database of Interacting Proteins (DIP, https://dip.doe- mbi.ucla.edu/dip/Main.cgi) [40], MatrixDB (http://matrixdb.univ-lyon1.fr/) [41] and Biological General Repository for Interaction Datasets (BioGRID, https://thebiogrid.org/) [42] for evaluating protein–protein interaction (PPI) network. Therefore, the interactions of genes (up and down regulated) were analyzed by online tool mentha. To prevent uncertain PPI, a high condence with interaction score more than 0.7 was set as signicance. After that, PPI network was constructed by cytoscape software (version 3.5.1) (http://www.cytoscape.org/) [43]. The plug-in CytoNCA [44] showed hub genes based on 5 centrality mathematical calculation methods such as node degree [45], betweenness [46], stress [47], closeness [48] and clustering coecient [49], the number of genes within 5 centrality mathematical calculation methods were represented the signicance of the disorder. Module analysis was done by the plug-in PEWCC1 [50]. Representative modules were listed by the criteria of both
Details
-
File Typepdf
-
Upload Time-
-
Content LanguagesEnglish
-
Upload UserAnonymous/Not logged-in
-
File Pages98 Page
-
File Size-