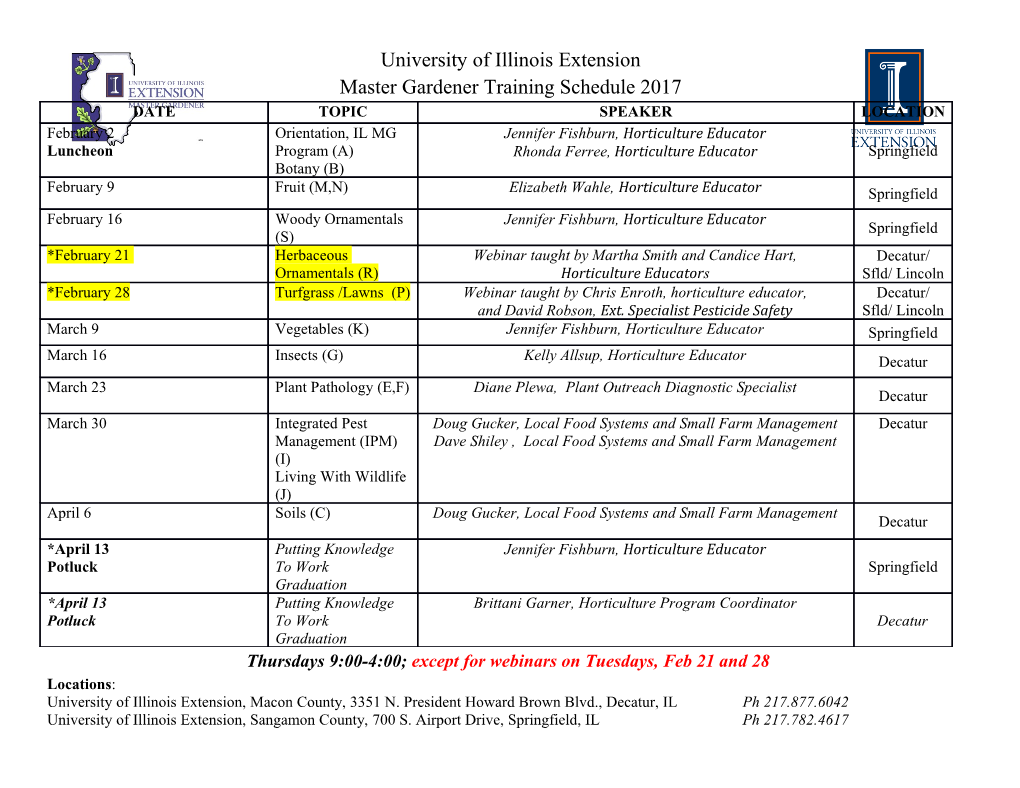
RS – EC2 - Lecture 18 Lecture 11 Unit Roots and Cointegration Brooks (4th edition): Chapter 8 1 Spurious Regression • Suppose yt and xt are non-stationary, I(1). That is, we differentiate them and the changes become stationary, or I(0). We regress yt against xt: What happens? • The usual t-tests on regression coefficients can show statistically significant coefficients, even if in reality it is not so. • This the spurious regression problem (Granger and Newbold (1974)): We find a statistically significant relation between unrelated variables. • In a Spurious Regression contexts, the regression errors would be highly correlated and the standard t-statistic will be wrongly calculated because the variance of the errors is not consistently estimated. 1 RS – EC2 - Lecture 18 Spurious Regression – Simulated Example Example: We simulate two independent random walks (RW1,t, RW2,t) and then regress one against the other: RW1,t = μ + β RW2,t + εt, With test the true H0: β = 0. sim_rw1 <- arima.sim(list(order=c(0,1,0)), sd=.5, n=500) # simulate RW series 1 sim_rw2 <- arima.sim(list(order=c(0,1,0)), sd=.5, n=500) # simulate RW series 2 Spurious Regression – Simulated Example Example: fit_sim_rw <- lm(sim_rw1 ~ sim_rw2) # Regression of two RWs res_sim_rw <- fit_sim_rw$residuals # Extract residuals > summary(fit_sim_rw) Coefficients: Estimate Std. Error t value Pr(>|t|) (Intercept) -4.61541 0.13188 -35.00 <2e-16 *** sim_rw2 -0.47384 0.04076 -11.62 <2e-16 *** Reject H0: β = 0. --- Signif. codes: 0 ‘***’ 0.001 ‘**’ 0.01 ‘*’ 0.05 ‘.’ 0.1 ‘ ’ 1 Residual standard error: 2.356 on 499 degrees of freedom Multiple R-squared: 0.2131, Adjusted R-squared: 0.2115 F-statistic: 135.1 on 1 and 499 DF, p-value: < 2.2e-16 Note: Very significant t-value (& F-goodness of fit stat), and a good R2. But, the model makes no sense. 2 RS – EC2 - Lecture 18 Spurious Regression – Real Examples Examples: (1) Egyptian infant mortality rate (Y), 1971-1990, annual data, on Gross aggregate income of American farmers (I) and Total Honduran money supply (M): 2 Yt = 179.9 - .2952 It -.0439 Mt, R = .918, DW = .4752, F = 95.17 (16.63) (-2.32) (-4.26) Corr(Yt, Xi,t) = .8858, -.9113, -.9445 (2). US Export Index (Y), 1960-1990, annual data, on Australian males’ life expectancy (X): 2 Yt = -2943. + 45.7974 Xt, R = .916, DW = .3599, F = 315.2 (-16.70) (17.76) Corr(Yt, Xt) = .9570 (3) Total Crime Rates in the US (Y), 1971-1991, annual data, on Life expectancy of South Africa (X): 2 Yt = -24569 + 628.9 Xt, R = .811, DW = .5061, F = 81.72 (-6.03) (9.04) Corr(Yt, Xt) = .9008 Spurious Regression – Real Examples Examples (continuation): (2). US Export Index (Y), 1960-1990, annual data, on Australian males’ life expectancy (X): 2 Yt = -2943. + 45.7974 Xt, R = .916, DW = .3599, F = 315.2 (-16.70) (17.76) Corr(Yt , Xt) = .9570 Australian males’ life expectancy US Exports Note: It looks like the trend is the common element between Yt & Xt. 3 RS – EC2 - Lecture 18 Spurious Regression – Statistical Implications • Suppose yt and xt are unrelated I(1) variables. We run the regression: y t x t t • True value of β=0. The above is a spurious regression and εt ∼ I(1). • Technical points: Phillips (1986) derived the following results: - β → 0 & β → Non-normal RV not necessarily centered at 0. This is the spurious regression phenomenon. -The OLS t-statistics for testing H0: β=0 diverge to ±∞ as T → ∞. Thus, with a large enough T it will appear that β is significant. - The usual R2 → 1 as T → ∞. The model appears to have good fit well, even though it is a bad (nonsense) model. Spurious Regression – Detection and Solutions • Given the statistical implications, the typical symptoms are: -High R2, t-values, & F-values. -Low DW values. • Q: How do we detect a spurious regression (between I(1) series)? - Check the correlogram of the residuals. (Lecture 9.) - Test for a unit root on the residuals. (This lecture.) 4 RS – EC2 - Lecture 18 Spurious Regression – Detection and Solutions • Statistical solution: When series (yt & Xt) are I(1), work with first differences, instead: Δyt = yt –yt-1 & Δ Xt = Xt– Xt-1 If the relation between the series yt and Xt exists, should be the same in levels (yt, Xt) or first differences (Δyt, ΔXt). Levels: yt = Xt + εt (*) Lagged Levels: yt-1 = Xt-1 + εt-1 (**) Subtract (**) from (*): We have a regression with 1st differences: First Differences: Δyt = ΔXt + ut,where ut = εt - εt-1 Now, we have a valid regression, since both Δyt & ΔXt are I(0). But, the economic interpretation of the regression changes. Spurious Regression – Simulated Example Example: We regress the two RW in first differences: diff_rw1 <- diff(sim_rw1) # First differences for RW 1 diff_rw2 <- diff(sim_rw2) # First differences for RW 2 fit_diff_rw <- lm(diff_rw1 ~ diff_rw2) > summary(fit_diff_rw) Coefficients: Estimate Std. Error t value Pr(>|t|) (Intercept) -0.003199 0.023481 -0.136 0.8917 diff_rw2 0.106339 0.044773 2.375 0.0179 * Reject H0: β = 0. --- Signif. codes: 0 ‘***’ 0.001 ‘**’ 0.01 ‘*’ 0.05 ‘.’ 0.1 ‘ ’ 1 Residual standard error: 0.525 on 498 degrees of freedom Multiple R-squared: 0.0112, Adjusted R-squared: 0.009215 F-statistic: 5.641 on 1 and 498 DF, p-value: 0.01792 Note: Still significant (by chance), but the coefficient changes sign. Clear indication that something is wrong with regression. 5 RS – EC2 - Lecture 18 Spurious Regression – Remarks • The message from spurious regression: Regression of I(1) variables can produce nonsense. Q: Does it make sense a regression between two I(1) variables? Yes, only if the regression errors are I(0). That is, when the variables are cointegrated. I(1) Process – Autoregressive Unit Root • A shock is usually used to describe an unexpected change in a variable or in the value of the error terms at a particular time period. • When we have a stationary system, the effect of a shock will die out gradually. But, when we have a non-stationary system, the effect of a shock is permanent. • We have two types of non-stationarity. In an AR(1) model we have: yt = μ +1 yt-1 + εt. - Unit root: | 1 | = 1: homogeneous non-stationarity - Explosive root: | 1 | > 1: explosive non-stationarity • In the last case, a shock to the system become more influential as time goes on. It is not seen in real life. We will not consider them. 6 RS – EC2 - Lecture 18 I(1) Process – Autoregressive Unit Root • Consider the AR(p) process: 1 2 p (L) yt t where (L) 11L L 2 .... p L As we discussed before, if one of the roots equals 1, Φ(1)=0, or 1 2 .... p 1 • We say yt has a unit root. In this case, yt is non-stationary. Example: AR(1): yt = μ +1 yt-1 + εt. Unit root: 1 = 1. H0 (yt non-stationarity): 1 = 1 (or, 1 –1 = 0) H1 (yt stationarity): 1 < 1 (or, 1 –1 < 0) • A t-test seems natural to test H0. But, the ergodic theorem & MDS CLT do not apply: the t-statistic does not have the usual distributions. Autoregressive Unit Root – Testing • Now, let’s reparameterize the AR(1) process. Subtract yt-1 from yt: yt -yt-1 = μ +1 yt-1 -yt-1 + εt. Δyt = μ + (1 –1) yt-1 + εt = μ + α0 yt-1 + εt • Unit root test: H0 (unit root process): α0 = 1 –1 = 0 H1(stationary process): α0 < 0. • Under H0 (unit root process): α0 = 0, the model is stationary in Δyt. Then, if yt has a unit root: Δyt = μ + εt. That is, Δyt is a stationary process with a drift and WN innovations. If we reject H0 (unit root): α0=0, then yt is a stationary AR(1) process: yt = μ + 1 yt-1 + εt. 7 RS – EC2 - Lecture 18 Autoregressive Unit Root – Testing • We have a linear regression framework: Δyt = μ + α0 yt-1+ εt. • The natural test for H0 (unit root process): α0 = 1 – 1 = 0: A t-test. ϕ 1 ϕ • We call this t-test the Dickey-Fuller (DF) test. • As mentioned above, under H0, the t-test does not have the usual t- distribution. We use a distribution tabulated (simulated) by DF. Autoregressive Unit Root – Testing • We derived the DF test from an AR(1) process for yt, but yt may follow a more general AR(p) process: (L)yt t We rewrite the process using the Dickey-Fuller reparameterization:: yt 0 yt1 1yt1 2yt2 .... p1yt( p1) t Note: Both AR(p) formulations are equivalent. • It can be shown that (1) = -α0. (Roots of (L)=0 equal to 1!) A unit root hypothesis can be stated, again, as H0: α0 = 0. H1: α0 < 0. • Like in the DF test, we have a linear regression framework. A t-test for H0 (unit root) is the Augmented Dickey-Fuller (ADF) test. 8 RS – EC2 - Lecture 18 Autoregressive Unit Root – Testing: DF • The Dickey-Fuller (DF) test is a special case of the ADF: No lags are included in the regression. • From our previous AR(1) process, we have: y t 1 y t 1 t 0 y t 1 t • If α0 = 0, yt has a unit root: H0 :0 0 H1 :0 0 (|0 | 0) ˆ 1 • We can test H0 with a t-test: t 1 SE ˆ Note: There is another associated test with H0, the ρ-test: (T-1) (-1). Autoregressive Unit Root – Testing: DF • is not asymptotically normally distributed and tФ=1 is not asymptotically standard normal.
Details
-
File Typepdf
-
Upload Time-
-
Content LanguagesEnglish
-
Upload UserAnonymous/Not logged-in
-
File Pages32 Page
-
File Size-